A Locally-Constrained Yolo Framework For Detecting Small And Densely-Distributed Building Footprints
INTERNATIONAL JOURNAL OF GEOGRAPHICAL INFORMATION SCIENCE(2020)
摘要
Building footprints are among the most predominant features in urban areas, and provide valuable information for urban planning, solar energy suitability analysis, etc. We aim to automatically and rapidly identify building footprints by leveraging deep learning techniques and the increased availability of remote sensing datasets at high spatial resolution. The task is computationally challenging due to the use of large training datasets and large number of parameters. In related work, You-Only-Look-Once (YOLO) is a state-of-the-art deep learning framework for object detection. However, YOLO is limited in its capacity to identify small objects that appear in groups, which is the case for building footprints. We propose a LOcally-COnstrained (LOCO) You-Only-Look-Once framework to detect small and densely-distributed building footprints. LOCO is a variant of YOLO. Its layer architecture is determined by the spatial characteristics of building footprints and it uses a constrained regression modeling to improve the robustness of building size predictions. We also present an invariant augmentation based voting scheme to further improve the precision in the prediction phase. Experiments show that LOCO can greatly improve the solution quality of building detection compared to related work.
更多查看译文
关键词
Building detection, deep learning, locally constrained, YOLO, remote sensing
AI 理解论文
溯源树
样例
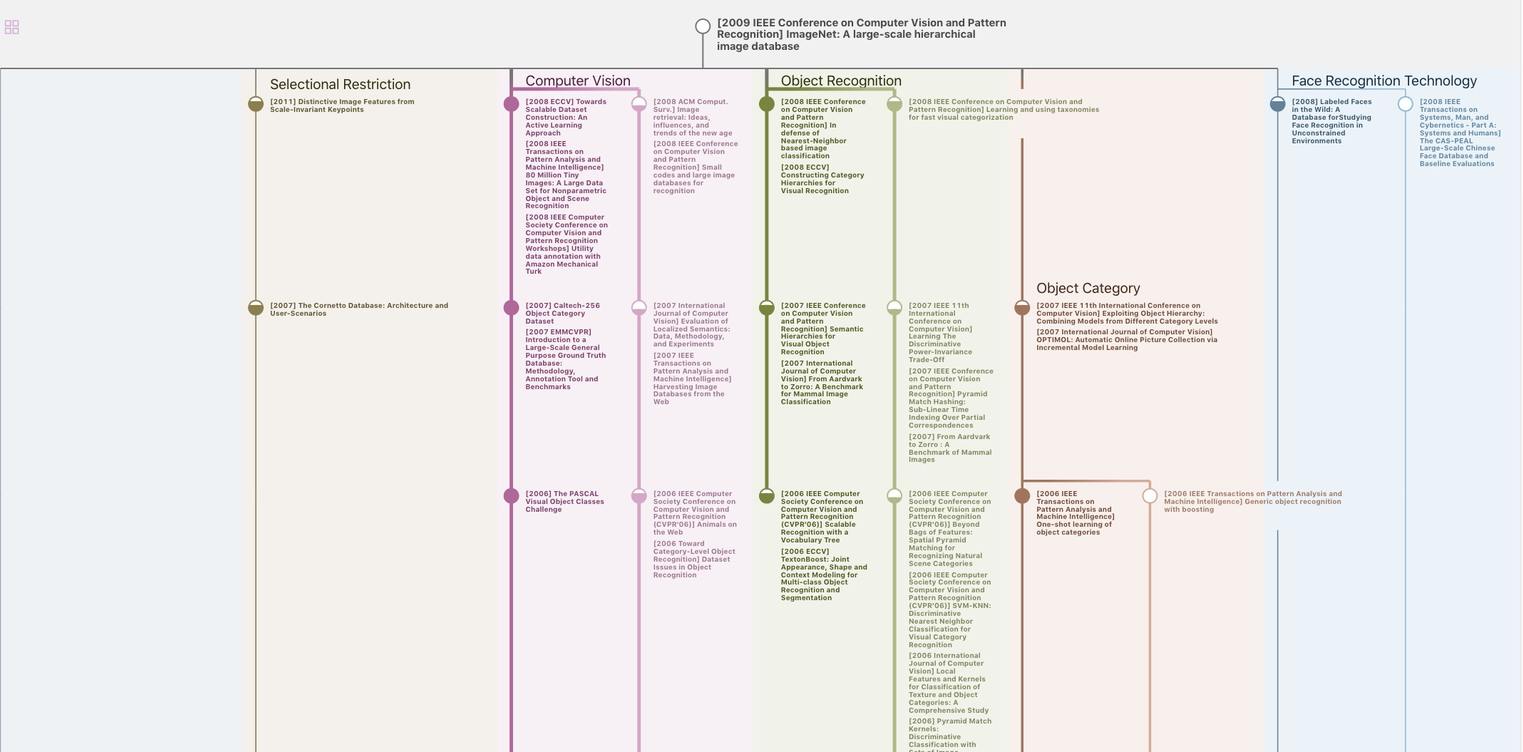
生成溯源树,研究论文发展脉络
Chat Paper
正在生成论文摘要