Lookahead Contraction Policies For Bayesian Ranking And Selection With Pairwise Comparisons
2019 WINTER SIMULATION CONFERENCE (WSC)(2019)
摘要
We propose and evaluate novel sampling policies for a Bayesian ranking and selection problem with pairwise comparisons. We introduce the lookahead contraction principle and apply it to three types of value factors for lookahead policies. The resulting lookahead contraction policies are analyzed both with the minimal number of lookahead steps required for obtaining informative value factors, and with fixed number of lookahead steps. We show that lookahead contraction reduces the minimal number of required lookahead steps, and that contraction guarantees finiteness of the minimal lookahead. For minimal lookahead we demonstrate empirically that lookahead contraction never leads to worse performance, and that lookahead contraction policies based on expected value of improvement perform best. For fixed lookahead, we show that all lookahead contraction policies eventually outperform their counterparts without contraction, and that contraction results in a performance boost for policies based on predictive probability of improvement.
更多查看译文
关键词
predictive probability,Bayesian ranking selection,lookahead contraction policies,lookahead steps,lookahead contraction principle,selection problem,sampling policies,contraction results,fixed lookahead
AI 理解论文
溯源树
样例
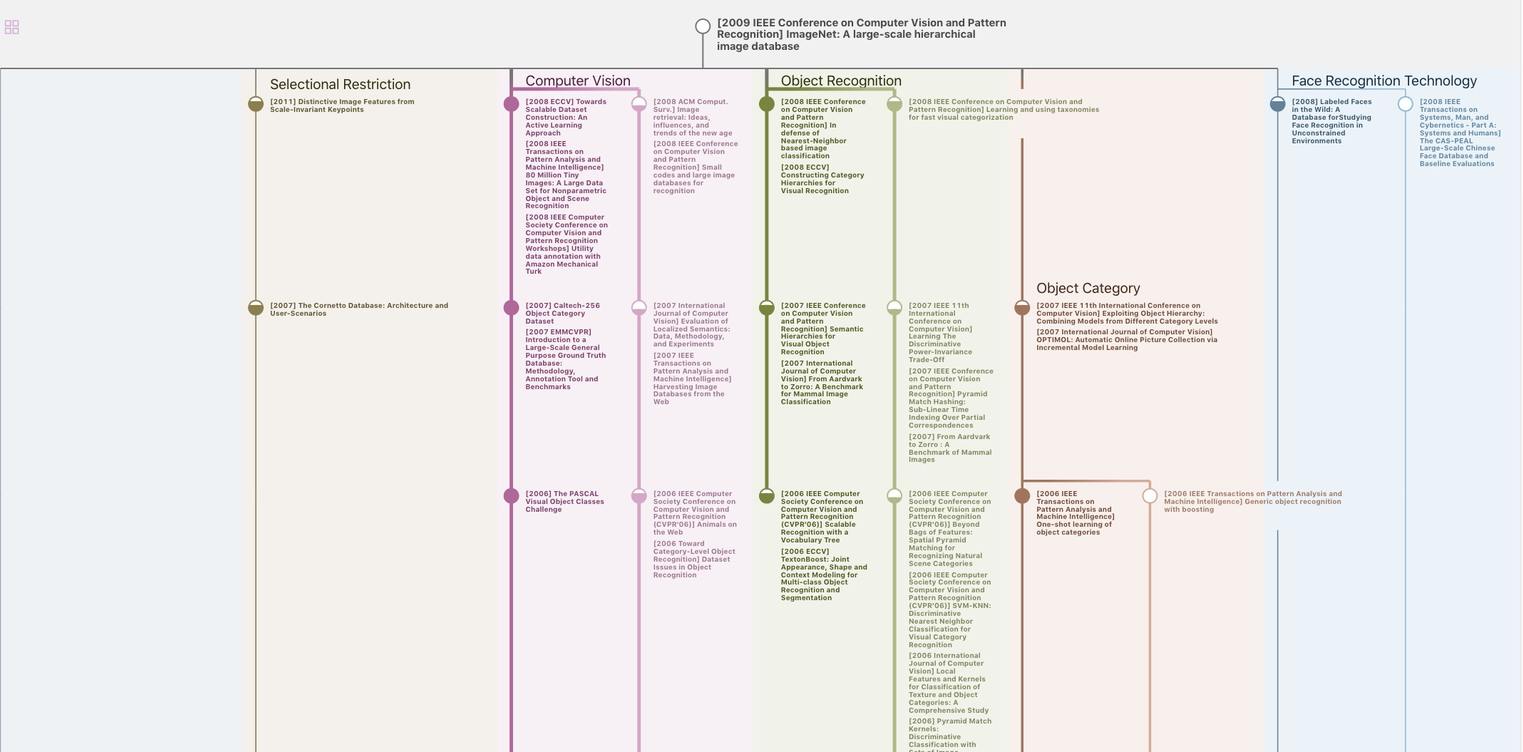
生成溯源树,研究论文发展脉络
Chat Paper
正在生成论文摘要