Social Regularized Collaborative Metric Learning.
HPCC/SmartCity/DSS(2019)
摘要
In recommender systems, Collaborative Metric Learning (CML) which learns a joint metric space to encode not only users' preferences but also the user-user and item-item similarity has achieved superior performance in comparison with matrix factorization techniques. However, how to integrate social information into metric learning based recommendation models to cope with the challenges of data sparsity remains as an open issue. For this, we present a Social Regularized Collaborative Metric Learning model (SRCML) for item recommendation. SRCML first defines a similarity function which integrates social preferences and rating preferences to measure user similarity, and then constructs a regularization component to reconstruct the loss function of collaborative metric learning, so that users with similar hobbies are closer to each other in the metric space. At the same time, the metric function that measures the distance between the users and the items is optimized. Finally, a model learning method based on gradient descent is given. Extensive experiments show that SRCML can improve the accuracy and recall rate of recommendations.
更多查看译文
关键词
Recommender Systems,Metric Learning,Social Regularization,Top-n Recommendation
AI 理解论文
溯源树
样例
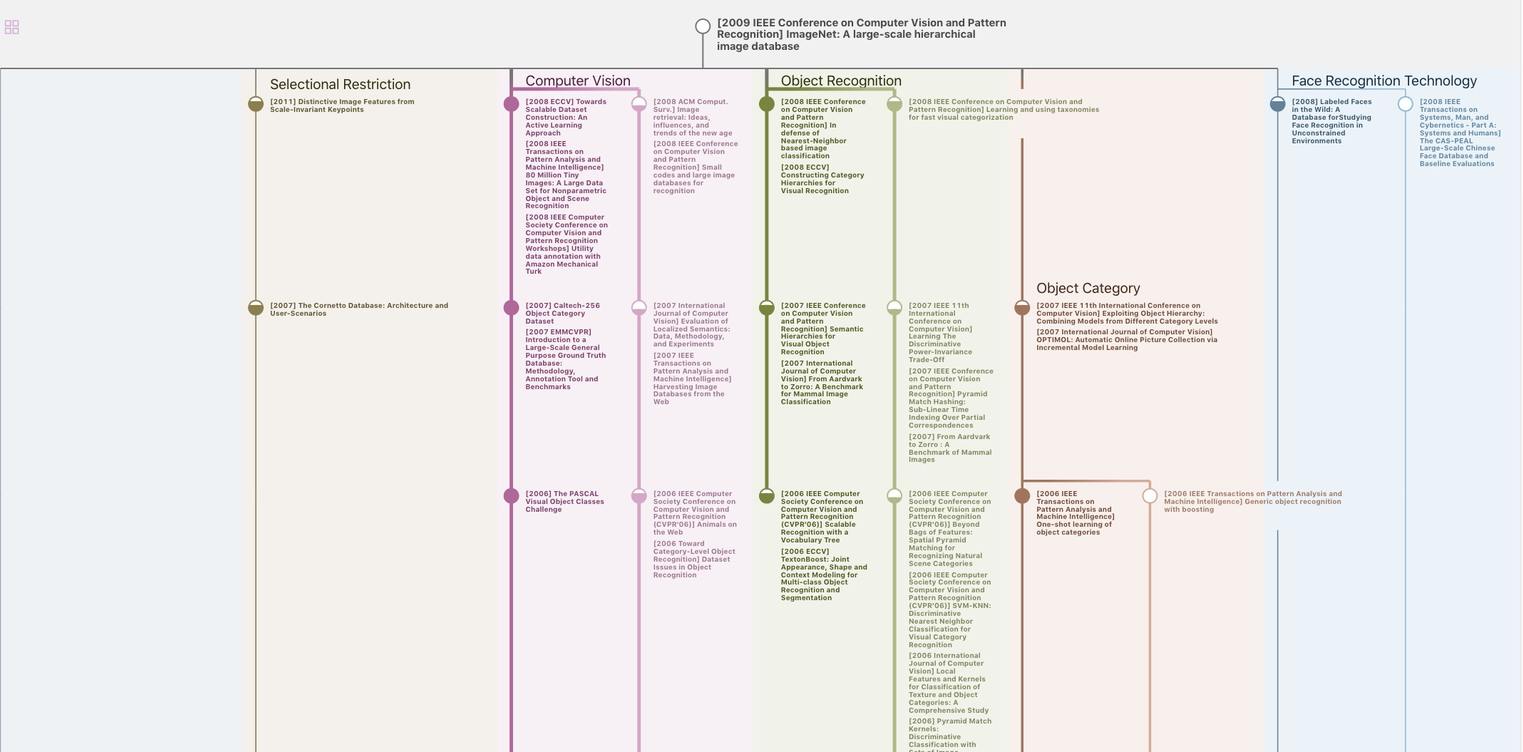
生成溯源树,研究论文发展脉络
Chat Paper
正在生成论文摘要