Insider Threat Detection Via Hierarchical Neural Temporal Point Processes
2019 IEEE INTERNATIONAL CONFERENCE ON BIG DATA (BIG DATA)(2019)
摘要
Insiders usually cause significant losses to organizations and are hard to detect. Currently, various approaches have been proposed to achieve insider threat detection based on analyzing the audit data that record information of the employee's activity type and time. However, the existing approaches usually focus on modeling the users' activity types but do not consider the activity time information. In this paper, we propose a hierarchical neural temporal point process model by combining the temporal point processes and recurrent neural networks for insider threat detection. Our model is capable of capturing a general nonlinear dependency over the history of all activities by the two-level structure that effectively models activity times, activity types, session durations, and session intervals information. Experimental results on two datasets demonstrate that our model outperforms the models that only consider information of the activity types or time alone.
更多查看译文
关键词
insider threat detection, temporal point process, hierarchical recurrent neural network
AI 理解论文
溯源树
样例
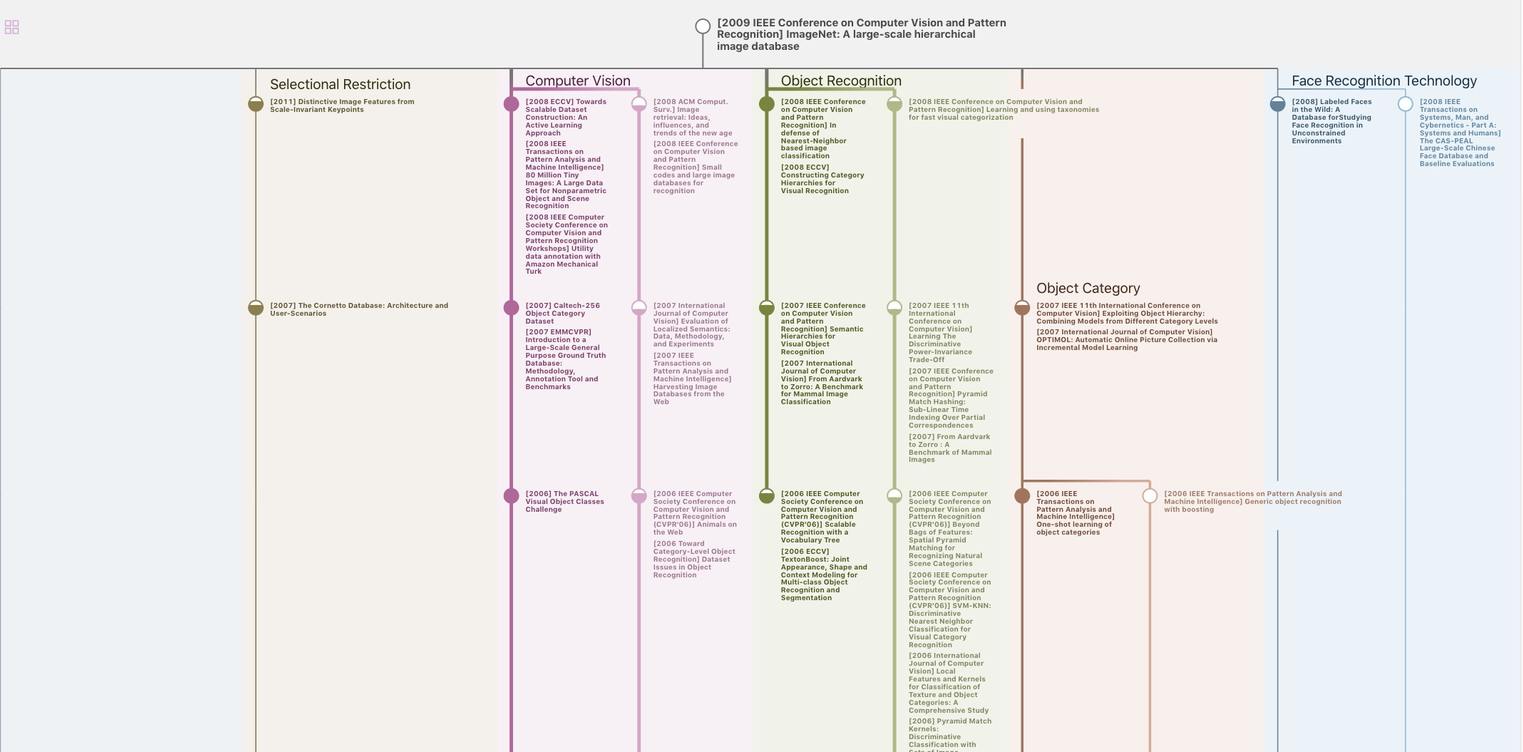
生成溯源树,研究论文发展脉络
Chat Paper
正在生成论文摘要