xYOLO: A Model For Real-Time Object Detection In Humanoid Soccer On Low-End Hardware
2019 International Conference on Image and Vision Computing New Zealand (IVCNZ)(2019)
摘要
With the emergence of onboard vision processing for areas such as the internet of things (IoT), edge computing and autonomous robots, there is increasing demand for computationally efficient convolutional neural network (CNN) models to perform real-time object detection on resource constraints hardware devices. Tiny-YOLO is generally considered as one of the faster object detectors for low-end devices and is the basis for our work. Our experiments on this network have shown that Tiny-YOLO can achieve 0.14 frames per second (FPS) on the Raspberry Pi 3 B, which is too slow for soccer playing autonomous humanoid robots detecting goal and ball objects. In this paper we propose an adaptation to the YOLO CNN model named xYOLO, that can achieve object detection at a speed of 9.66 FPS on the Raspberry Pi 3 B. This is achieved by trading an acceptable amount of accuracy, making the network approximately 70 times faster than Tiny-YOLO. Greater inference speed-ups were also achieved on a desktop CPU and GPU. Additionally we contribute an annotated Darknet dataset for goal and ball detection.
更多查看译文
关键词
CNN,RoboCup,Object Detection
AI 理解论文
溯源树
样例
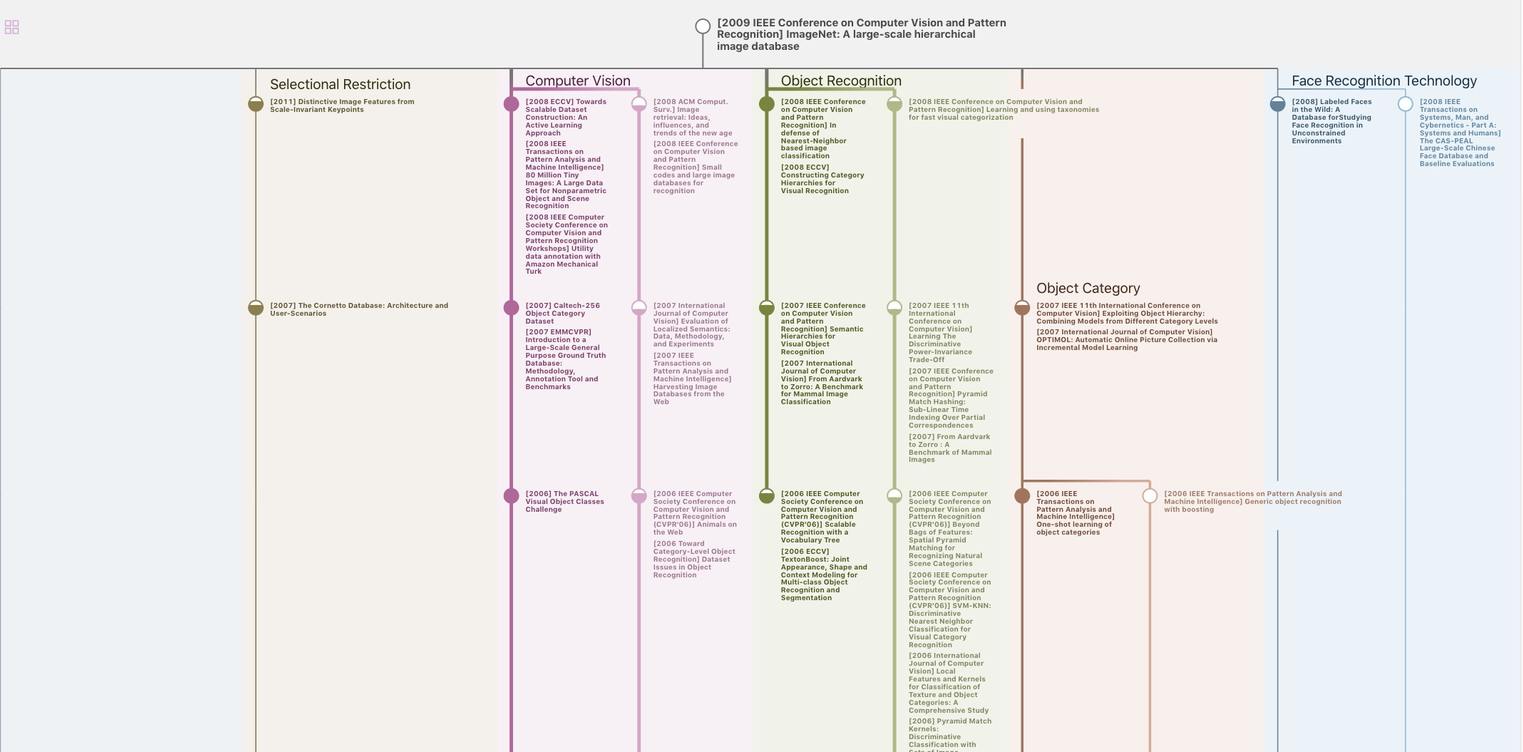
生成溯源树,研究论文发展脉络
Chat Paper
正在生成论文摘要