Where to find needles in a haystack?
TEST(2021)
摘要
In many existing methods of multiple comparison, one starts with either Fisher’s p value or the local fdr. One commonly used p value, defined as the tail probability exceeding the observed test statistic under the null distribution, fails to use information from the distribution under the alternative hypothesis. The targeted region of signals could be wrong when the likelihood ratio is not monotone. The oracle local fdr based approaches could be optimal because they use the probability density functions of the test statistic under both the null and alternative hypotheses. However, the data-driven version could be problematic because of the difficulty and challenge of probability density function estimation. In this paper, we propose a new method, Cdf and Local fdr Assisted multiple Testing method (CLAT), which is optimal for cases when the p value based methods are optimal and for some other cases when p value based methods are not. Additionally, CLAT only relies on the empirical distribution function which quickly converges to the oracle one. Both the simulations and real data analysis demonstrate the superior performance of the CLAT method. Furthermore, the computation is instantaneous based on a novel algorithm and is scalable to large data sets.
更多查看译文
关键词
Neyman-Pearson lemma, Monotone likelihood ratio, Convergence rate, 62H15
AI 理解论文
溯源树
样例
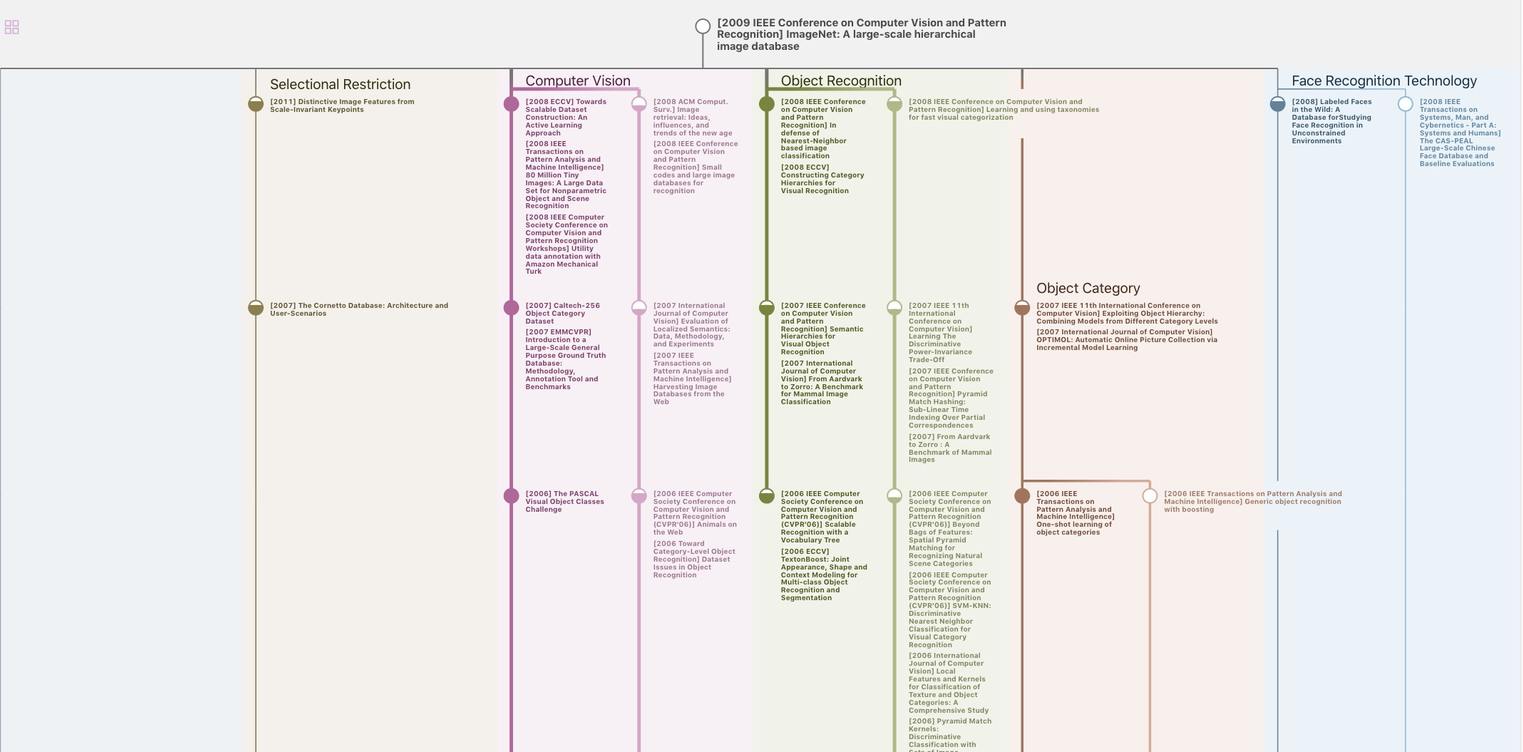
生成溯源树,研究论文发展脉络
Chat Paper
正在生成论文摘要