Unsupervised Feature Learning For Visual Place Recognition In Changing Environments
2019 INTERNATIONAL JOINT CONFERENCE ON NEURAL NETWORKS (IJCNN)(2019)
摘要
Visual place recognition in changing environments is a challenging and critical task for autonomous robot navigation. Deep convolutional neural networks (ConvNets) have recently been used as efficient feature extractors and obtained excellent performance in place recognition. However the success of ConvNets' learning highly relies on the availability of large datasets with millions of labeled images, the collection of which is a tedious and costly burden. Thus we develop an unsupervised learning method (the siamese VisNet) to autonomously learn invariant features in changing environments from unlabeled images. The siamese VisNet has two identical branches of sub-networks. With a Hebbian-type of learning rule incorporating a trace of previous activity patterns, the siamese VisNet learns features with increasing invariance in changing environments from layer to layer. Experiments conducted on multiple datasets demonstrate the robustness of the siamese VisNet against viewpoint changes, appearance changes, and joint viewpoint-appearance changes. In addition, the siamese VisNet, with lower complexity in architecture, outperforms the state-of-the-art place recognition ConvNets such as the CaffeNet and the PlaceNet. The proposed siamese VisNet constitutes a biologically plausible yet efficient method for unsupervised place recognition.
更多查看译文
关键词
visual place recognition, changing environments, unsupervised learning, siamese VisNet
AI 理解论文
溯源树
样例
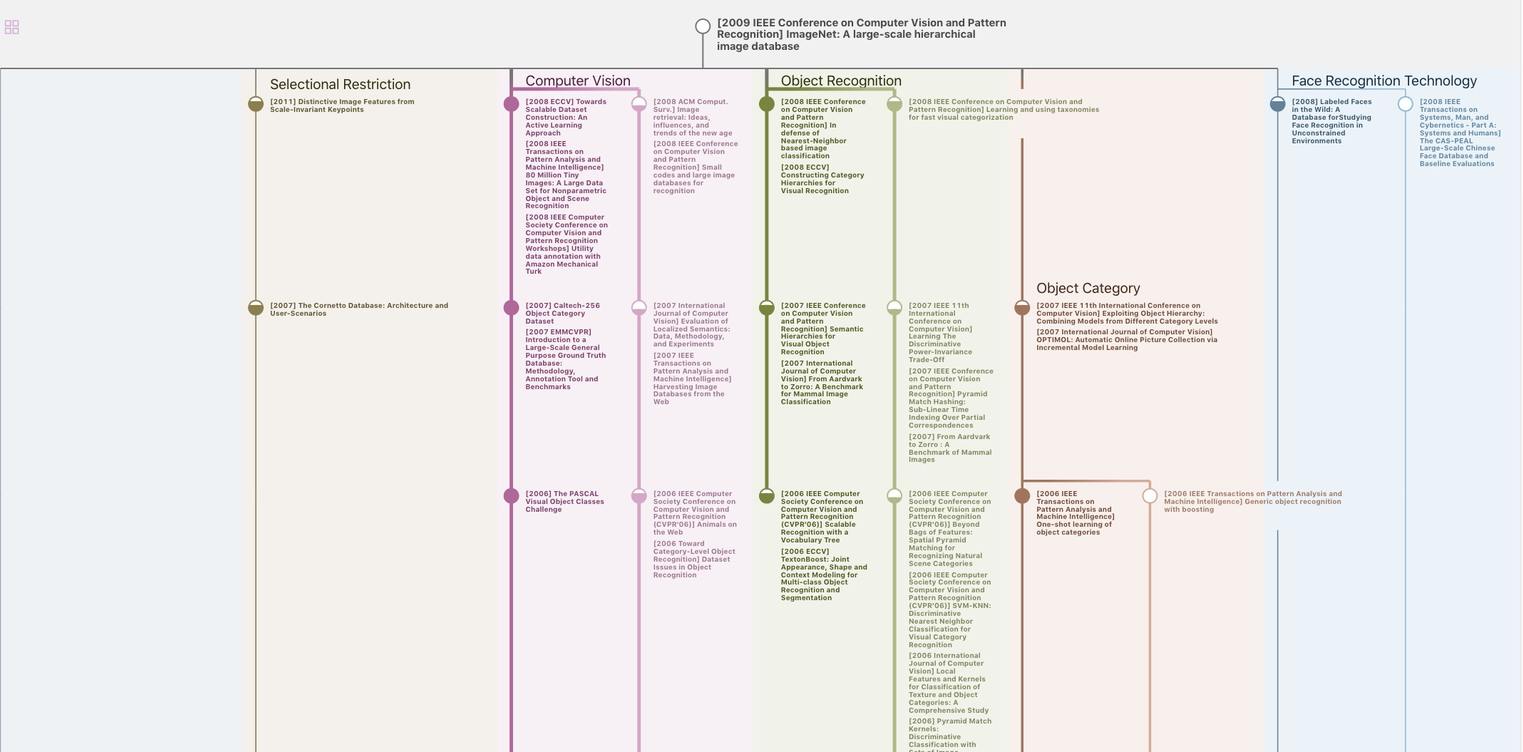
生成溯源树,研究论文发展脉络
Chat Paper
正在生成论文摘要