A Novel Two-Factor Attention Encoder-Decoder Network Through Combining Temporal And Prior Knowledge For Weather Forecasting
2019 INTERNATIONAL JOINT CONFERENCE ON NEURAL NETWORKS (IJCNN)(2019)
摘要
This paper proposes a novel two-factor attention based encoder-decoder model (TwoFactorEncoderDecoder) for multivariate weather prediction. The proposed model learns attention weights from two factors, namely, temporal information and prior knowledge inferred information. Here, temporal information contains change patterns hidden in observed time series data, while prior knowledge inferred information gives various types of meteorological observations in weather forecasting. Attention weights of the two factors are used to select the intermediate outputs of the encoder, and then combine the selected result with information inferred by prior knowledge for weather forecasting by a more effective way. In addition, this paper proposes a loss function for multivariate prediction. Compared with Mean Square Error (MSE) loss function, the proposed loss function can fit small variances more accurately in performing multivariate prediction. Compared with the attention model that only uses temporal information or the prior knowledge inferred information, the proposed TwoFactorEncoderDecoder model has encouraging improvements in prediction accuracy on the public weather forecasting dataset, namely, the MAPE of t2m is increased by 5.42%, the MAPE of rh2m is increased by 2.92%, and the MAPE of w2m is increased by 1.67%, which shows the effect of the two-factor attention mechanism. Source code for the complete system will be available at https://github.com/YuanMLer/TFAEncoderDecoder.
更多查看译文
关键词
two-factor attention, weather forecasting, encoder-decoder model
AI 理解论文
溯源树
样例
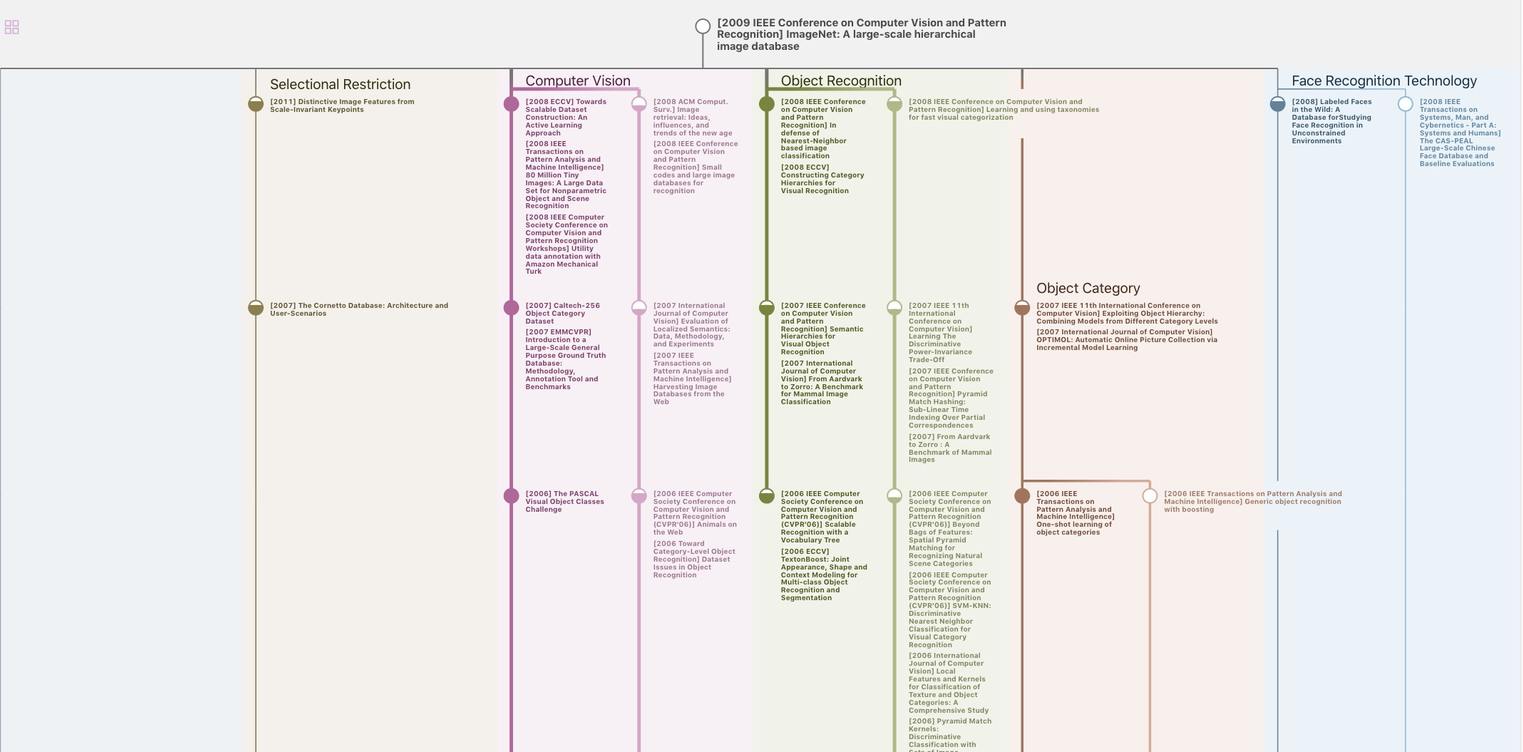
生成溯源树,研究论文发展脉络
Chat Paper
正在生成论文摘要