Attention-Based Adversarial Training For Seamless Nudity Censorship
2019 INTERNATIONAL JOINT CONFERENCE ON NEURAL NETWORKS (IJCNN)(2019)
摘要
The amount of digital pornographic content over the Internet grows daily and accessing such a content has become increasingly easier. Hence, there is a real need for mechanisms that can protect particularly-vulnerable audiences (e.g., children) from browsing the web. Recently, object detection methods based on deep neural networks such as CNNs have improved the effectiveness and efficiency of identifying and blocking pornographic content. Even though improvements in detecting intimate parts have been significant, the occlusion of the content is still primarily done by either blurring or removing regions of the image in an intrusive fashion. A recent study has addressed the problem of censoring the pornographic content in a non-intrusive way by generating the so-called seamless censorship via cycle-consistent generative adversarial networks. Such an approach has managed to automatically add bikinis to naked women without explicit supervision or paired training data. In this paper, we extend that method by designing a novel cycle-consistency framework that leverages sensitive information from an attention-based multi-label convolutional neural network. We evaluate the quality of our novel generative model by conducting a web survey with over 1000 opinions regarding the resulting images from our method and from baseline approaches. Results of the survey show that our method considerably improves the state-of-the-art on the seamless censorship task.
更多查看译文
关键词
adversarial training, attention, convolutional neural networks, deep learning, GANs, pornography censorship
AI 理解论文
溯源树
样例
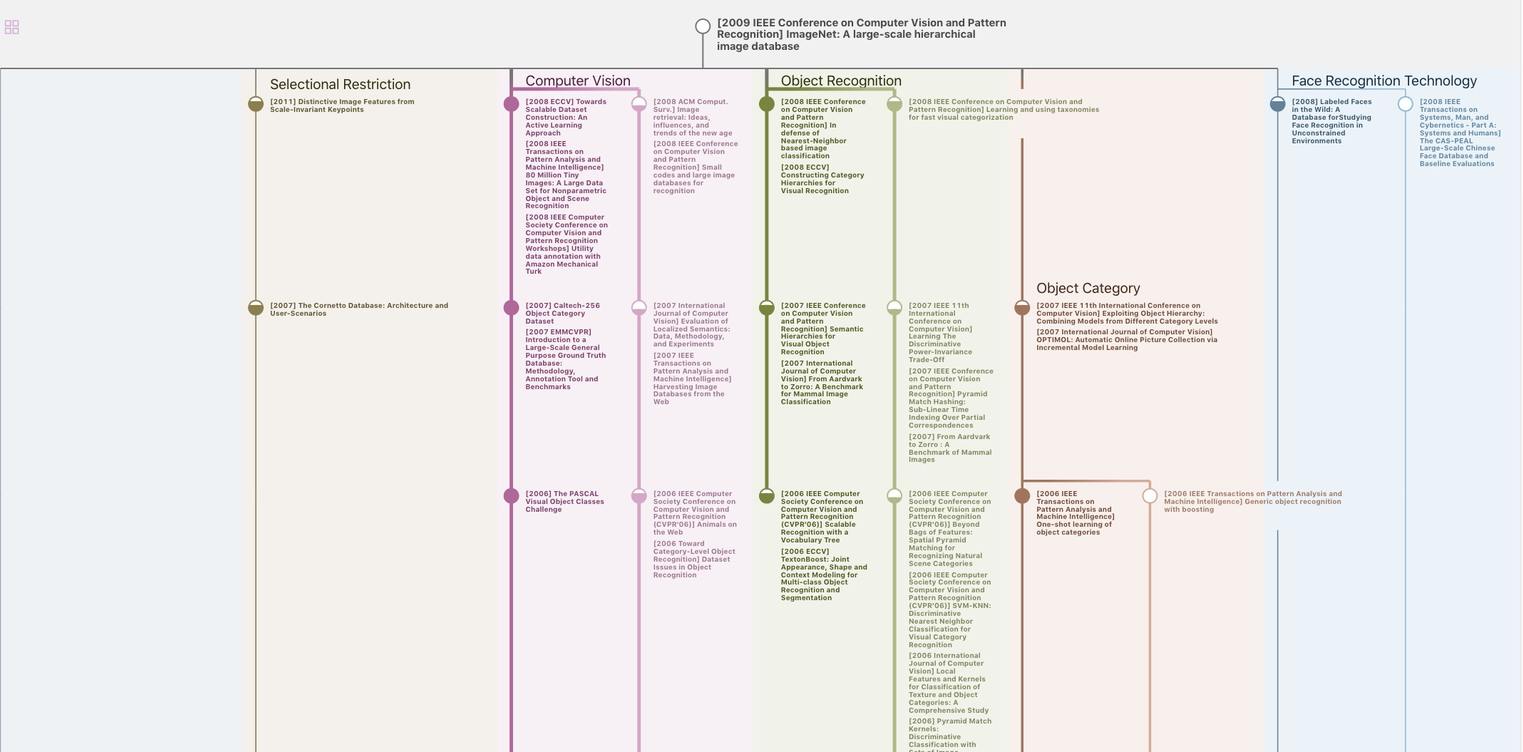
生成溯源树,研究论文发展脉络
Chat Paper
正在生成论文摘要