Multi-Agent Deep Reinforcement Learning with Emergent Communication.
IJCNN(2019)
摘要
When compared with their single-agent counterpart, multi-agent systems have an additional set of challenges for reinforcement learning algorithms, including increased complexity, non-stationary environments, credit assignment, partial observability, and achieving coordination. Deep reinforcement learning has been shown to achieve successful policies through implicit coordination, but does not handle partial-observability. This paper describes a deep reinforcement learning algorithm, based on multi-agent actor-critic, that simultaneously learns action policies for each agent, and communication protocols that compensate for partial-observability and help enforce coordination. We also research the effects of noisy communication, where messages can be late, lost, noisy, or jumbled, and how that affects the learned policies. We show how agents are able to learn both high-level policies and complex communication protocols for several different partially-observable environments. We also show how our proposal outperforms other state-of-the-art algorithms that don't take advantage of communication, even with noisy communication channels.
更多查看译文
关键词
multi-agent systems,neural networks,noisy communication,reinforcement learning,distributed deep learning
AI 理解论文
溯源树
样例
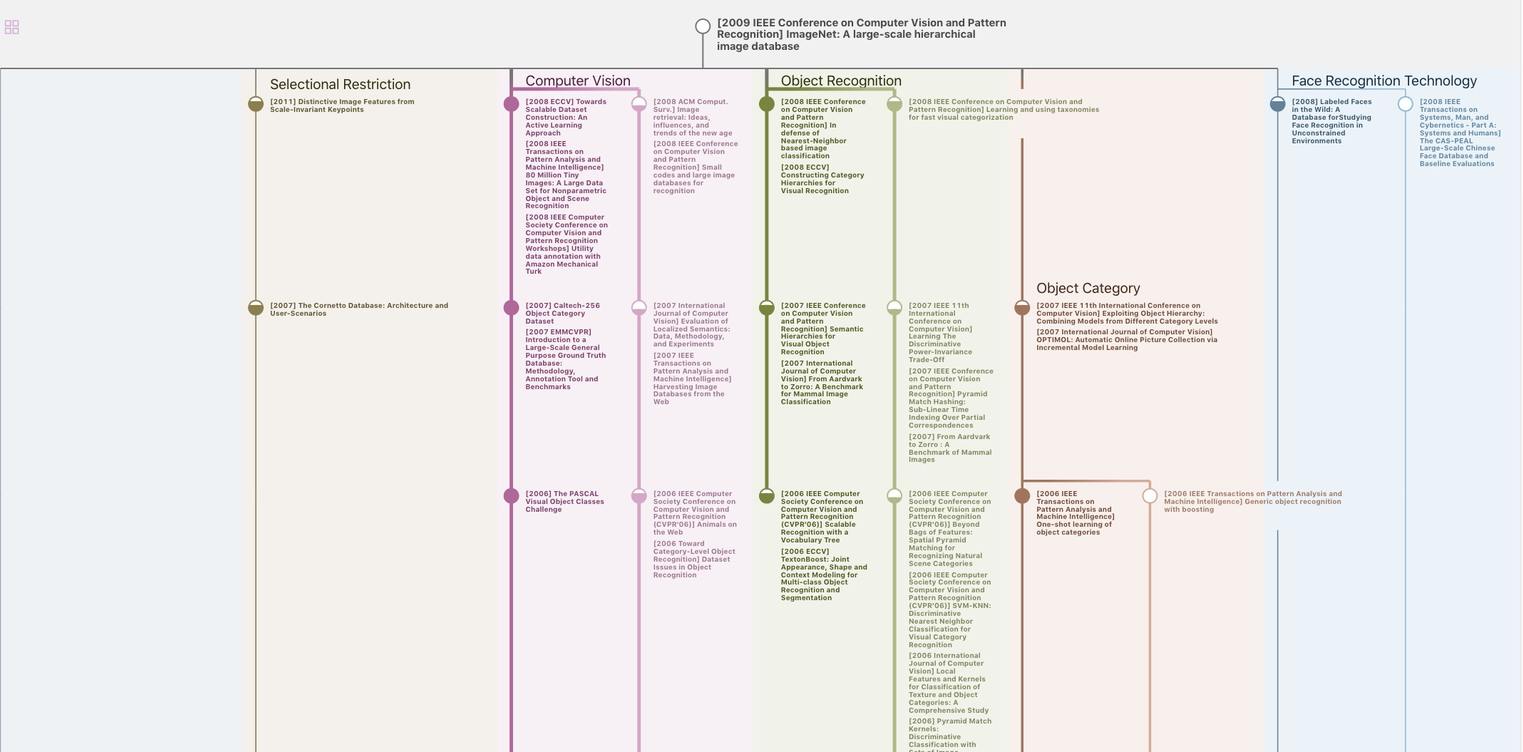
生成溯源树,研究论文发展脉络
Chat Paper
正在生成论文摘要