Sparsity As The Implicit Gating Mechanism For Residual Blocks
2019 INTERNATIONAL JOINT CONFERENCE ON NEURAL NETWORKS (IJCNN)(2019)
摘要
Neural networks are the core component in the recent empirical successes of deep learning techniques in challenging tasks. Residual network (ResNet) architectures have been instrumental in improving performance in object recognition and other tasks by enabling training much deeper neural networks. Studies of residual networks reveal that they are robust to removing layers. However, it is still an open question of why residual networks behave well and how they make it feasible to train networks with many layers. In this paper, we show that sparsity of the residual blocks acts as the implicit gating mechanism. When a neuron is inactive, it behaves as a node in an information highway, allowing the information from the previous layer to pass to the next layer unchanged. As the identity function has a derivative of 1, it avoids the exploding or vanishing gradient problem that is known to contribute to the difficulty of training deep neural networks. When a neuron is active, it captures input-output relationships that are necessary to achieve good performance. By using the ReLu activation functions, residual blocks produce sparse outputs for typical inputs. We perform systematic experimental analysis on the residual blocks of trained ResNet models and show that sparsity acts as the implicit gate for deep residual networks.
更多查看译文
关键词
implicit gating mechanism,residual blocks,deep learning techniques,residual network architectures,deep neural networks,deep residual networks,object recognition,input-output relationships,ReLu activation functions
AI 理解论文
溯源树
样例
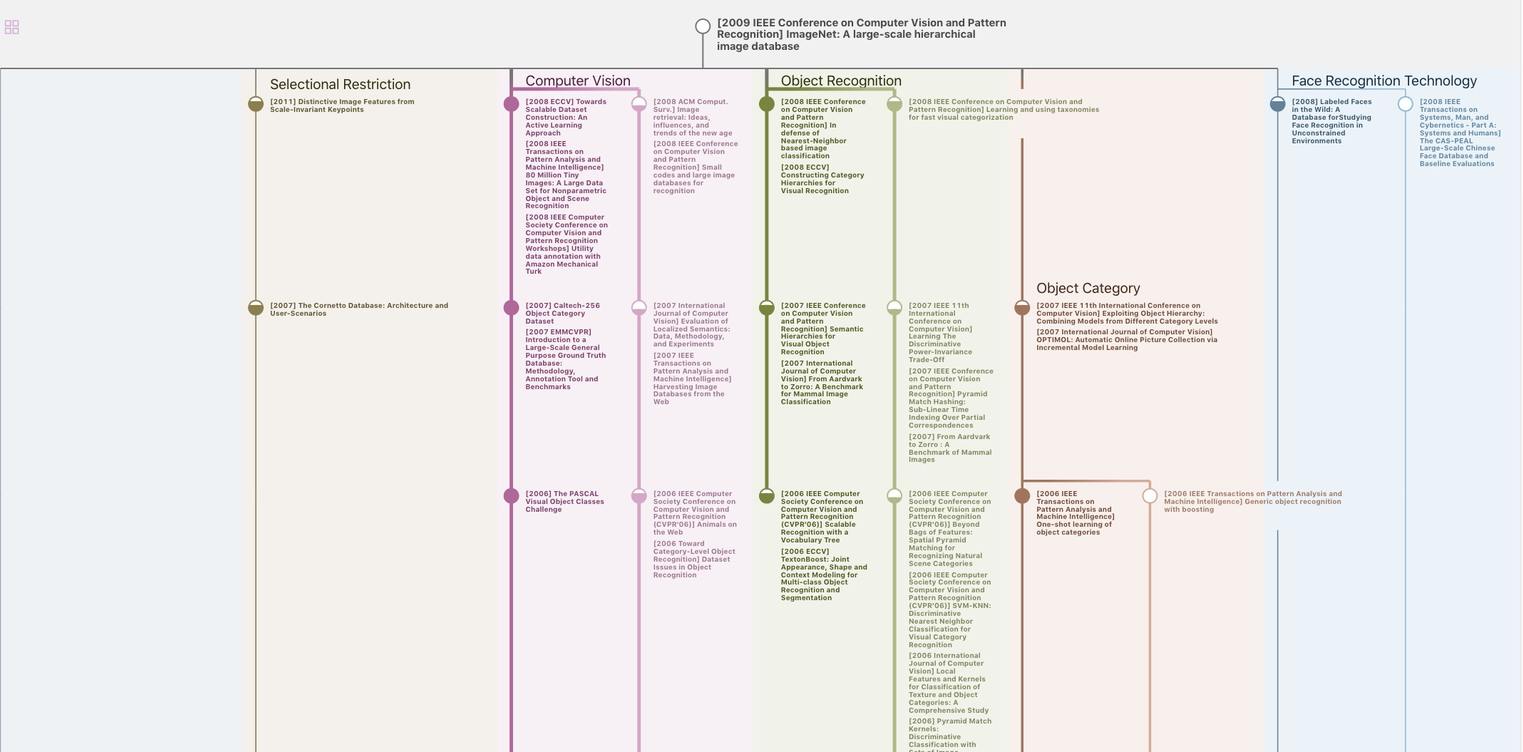
生成溯源树,研究论文发展脉络
Chat Paper
正在生成论文摘要