Attention-Driven Driving Maneuver Detection System
2019 INTERNATIONAL JOINT CONFERENCE ON NEURAL NETWORKS (IJCNN)(2019)
摘要
Driving Maneuver early Detection (DMD) is one of the most important tasks in Advanced Driver Assistance Systems (ADAS), it provides the early notification necessary for ADAS to predict dangerous circumstances and take appropriate actions. The end-to-end architectures such as Recurrent Neural Networks (RNNs) take advantage of deep networks to automatically learn non-linear discriminative features, which significantly boost the performances of DMD systems. However, due to the large number of parameters in the deep architectures, learning effective discriminative features requires millions of labeled images. Moreover the discriminative features are generally meaningless to human being, which makes the diagnose of end-to-end architectures extremely difficult. In this paper, we propose a novel DMD system, denoted as Attention-driven Driving Maneuver Detection system (ADMD), which uses drivers' attention as an intermediate concept (hint) to explore and understand the causal relation between driving surroundings and driving maneuvers. In the training phase, ADMD distills the knowledge from drivers' attention prediction model to provide initial search areas for learning effective features, which minimizes the labeled data requirements for training deep architectures and results in a fast optimization process. We compared the performances of ADMD with the state-of-the-art methods on 1953 miles (37 hours) of natural driving data collected from 7 drivers. The experimental results shows ADMD is capable of achieving better performances, which are mostly attributed to the proposed novel learning mechanism used to obtain meaningful interpretations of the driving surroundings that are closely related to the driver's intend maneuvers.
更多查看译文
关键词
ADAS,end-to-end architectures,deep networks,nonlinear discriminative features,DMD system,deep architectures,ADMD,natural driving data,advanced driver assistance systems,recurrent neural networks,learning mechanism,attention-driven driving maneuver detection system,driving maneuver early detection,labeled images,fast optimization process
AI 理解论文
溯源树
样例
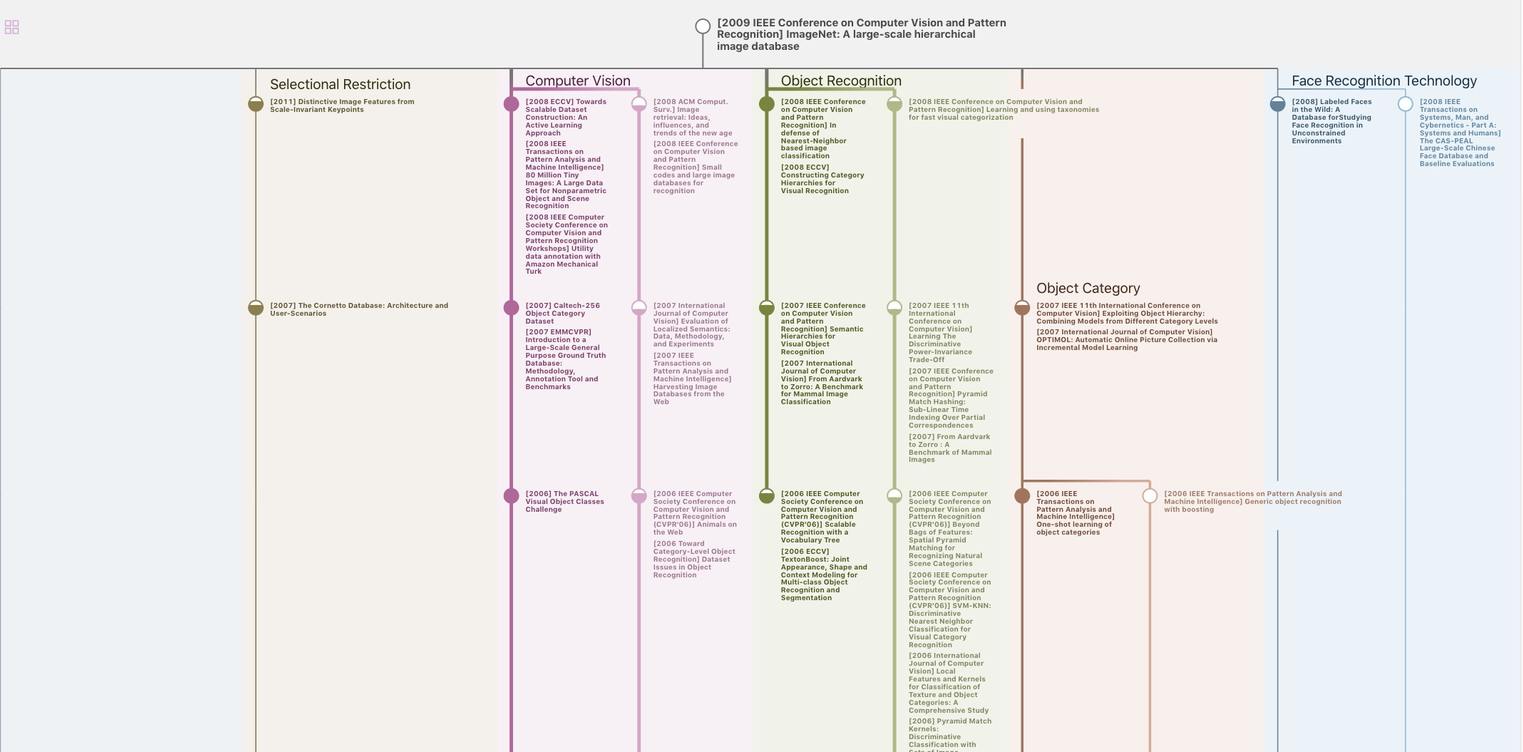
生成溯源树,研究论文发展脉络
Chat Paper
正在生成论文摘要