Spatio-Temporal Active Learning For Visual Tracking
2019 INTERNATIONAL JOINT CONFERENCE ON NEURAL NETWORKS (IJCNN)(2019)
摘要
The success of state-of-the-art deep learning based trackers is fuelled by the large-scale datasets. However, not all training data has a gain on model performance, and some data can even degrade the performance of the model. Therefore, we aim to train state-of-the-art trackers using a part of labeled frame with high information and less data. To this end, we propose a novel framework called STAL(spatio-temporal active learning strategy), which is integrated with an efficient deep tracker and a spatio-temporal active learning strategy. Specifically, we first mine the most informative frames to boost the deep tracker based on the corresponding spatio-temporal response scores of the target. Then, the hard and easy frames are labeled from annotation and machine auto-annotation, respectively. Hard and easy sample pairs are generated from selected frames. To alleviate the impact of sample pairs with large loss, a self-paced fully convolutional Siamese network is proposed by introducing a norm negative regularization. The STAL framework will converge well and output promising tracking performance on several publicly available datasets.
更多查看译文
关键词
training data,labeled frame,spatio-temporal active learning strategy,efficient deep tracker,informative frames,hard frames,easy frames,deep learning based trackers
AI 理解论文
溯源树
样例
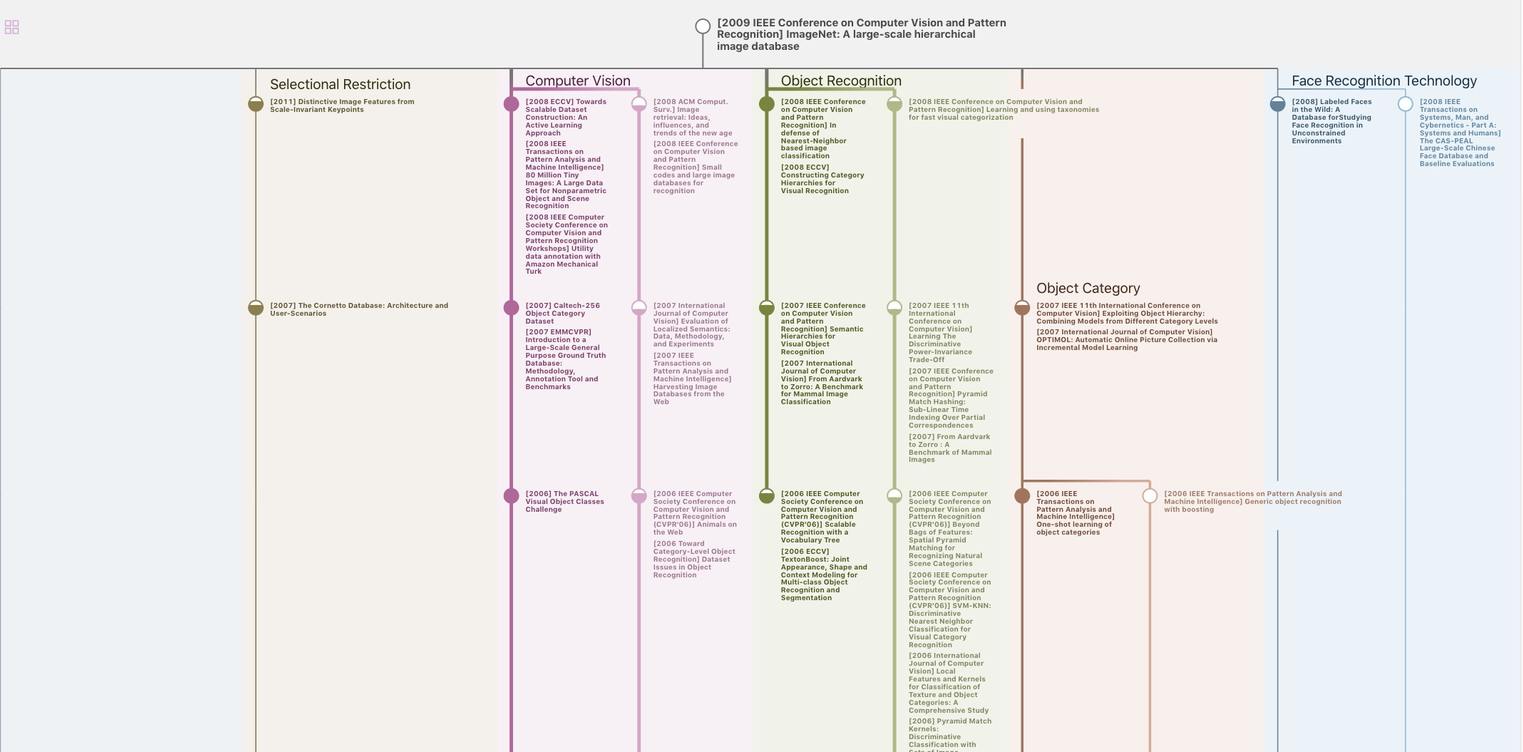
生成溯源树,研究论文发展脉络
Chat Paper
正在生成论文摘要