A Time-Frequency based Machine Learning System for Brain States Classification via EEG Signal Processing.
IJCNN(2019)
摘要
In the last decades, the use of Machine Learning (ML) algorithms have been widely employed to aid clinicians in the difficult diagnosis of neurological disorders, such as Alzheimer's disease (AD). In this context, here, a data-driven ML system for classifying Electroencephalographic (EEG) segments (i.e. epochs) of patients affected by AD, Mild Cognitive Impairment (MCI) and Healthy Control (HC) individuals, is introduced. Specifically, the proposed ML system consists of evaluating the average Time-Frequency Map (aTFM) related to a 19-channels EEG epoch and extracting some statistical coefficients (i.e. mean, standard deviation, skewness, kurtosis and entropy) from the main five conventional EEG sub-bands (or EEG-rhythms: delta, theta, alpha
1
, alpha
2
, beta). Afterwards, the time-frequency features vector is fed into an Autoeconder (AE), a Multi-Layer Perceptron (MLP), a Logistic Regression (LR) and a Support Vector Machine (SVM) based classifier to perform the 2-ways EEG epoch-classification tasks: AD vs HC and AD vs MCI. The performances of the proposed approach have been evaluated on a dataset of 189 EEG signals (63 AD, 63 MCI and 63 HC), recorded during an eye-closed resting condition at IRCCS Centro Neurolesi Bonino Pulejo of Messina (Italy). Experimental results reported that the 1-hidden layer MLP (MLP
1
) outperformed all the other developed learning systems as well as recently proposed state-of-the-art methods, achieving accuracy rate up to 95.76 % ± 0.0045 and 86.84% ± 0.0098 in AD vs HC and AD vs MCI classification, respectively.
更多查看译文
关键词
Machine learning,Time-Frequency features,EEG recording,Alzheimer's disease,Mild Cognitive Impairment
AI 理解论文
溯源树
样例
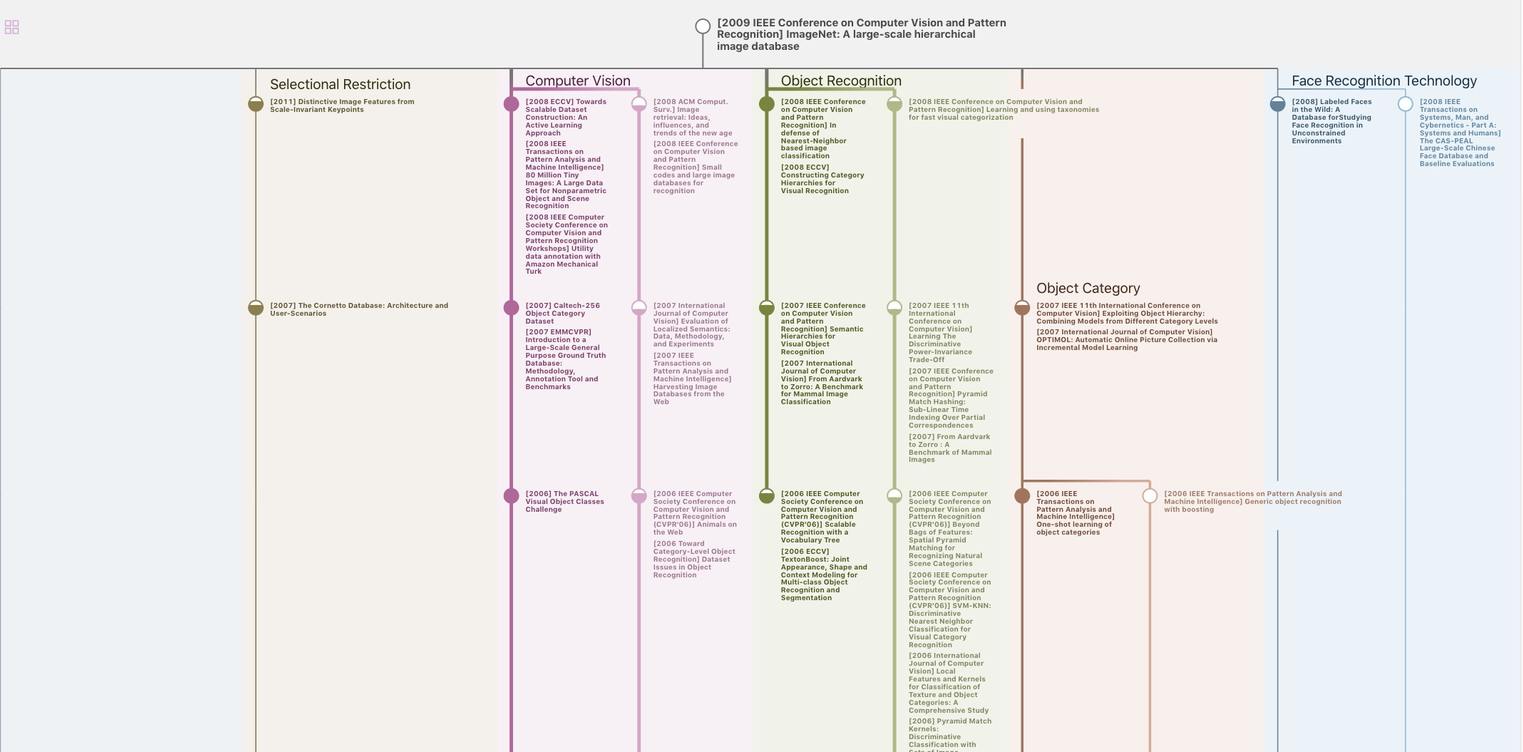
生成溯源树,研究论文发展脉络
Chat Paper
正在生成论文摘要