Deep Structure Learning For Rumor Detection On Twitter
2019 INTERNATIONAL JOINT CONFERENCE ON NEURAL NETWORKS (IJCNN)(2019)
摘要
With the development of social media and the popularity of mobile devices, it becomes increasingly easy to post rumors and spread rumors on social media. Widespread rumors may cause public panic and negative impact on individuals, which makes the automatic detection of rumors become necessary. Most existing methods for automatic rumor detection focus on modeling features related to contents, users and propagation patterns based on feature engineering, but few work consider the existence of graph structural information in the user behavior. In this paper, we propose a model that leverages graph convolutional networks to capture user behavior effectively for rumor detection. Our model is composed of three modules: 1) a user encoder that models users attributes and behaviors based on graph convolutional networks to obtain user representation; 2) a propagation tree encoder, which encodes the structure of the rumor propagation tree as a vector with bridging the content semantics and propagation clues; 3) an integrator that integrates the output of the above modules to identify rumors. Experimental results on two public Twitter datasets show that our model achieves much better performance than the state-of-the-art methods.
更多查看译文
关键词
rumor detection, user embedding, graph convolutional networks
AI 理解论文
溯源树
样例
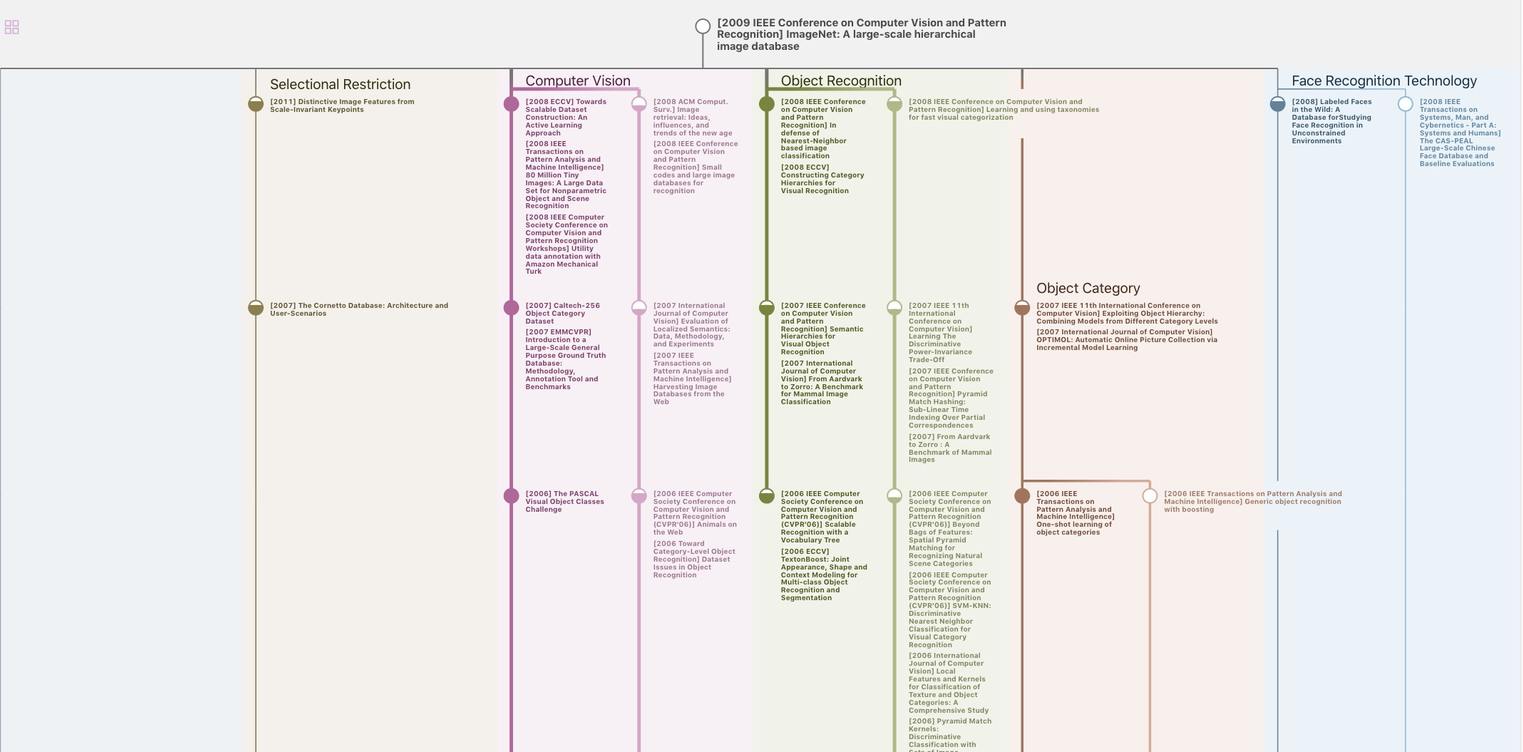
生成溯源树,研究论文发展脉络
Chat Paper
正在生成论文摘要