Correlation Filter Tracking Method Via Metric Learning And Adaptive Multi-Stage Appearance
2019 INTERNATIONAL JOINT CONFERENCE ON NEURAL NETWORKS (IJCNN)(2019)
摘要
In the complex tracking environment such as background clutter, generally there are multiple peaks in the response map of the correlation filter. It is difficult to distinguish the real object from the interference; and using the fixed learning rate to update the appearance model, it is not only difficult to maintain the sample diversity, but also easy to introduce noise information. Aiming at this problem, this paper proposes a correlation filter tracking method via metric learning and adaptive multi-stage appearance. By introducing metric learning to discriminate candidate samples corresponding to multiple peaks in the response map, the influence of multi-peak response map on tracking results in complex environments such as background clutter is eliminated; the Gaussian mixture model is used to divide the object appearance samples into groups and assign corresponding weights according to the duration, and the redundant information is eliminated while maintaining the diversity of the appearance model samples. The experimental results on OTB100 and VOT2017 datasets show that the overall precision score obtained by the algorithm in this paper is 0.866. The overall success plot score is 0.628, and the expected average overlap score is 0.211, which is better than most existing tracking methods.
更多查看译文
关键词
object tracking, correlation filtering, metric learning, Gaussian mixture model
AI 理解论文
溯源树
样例
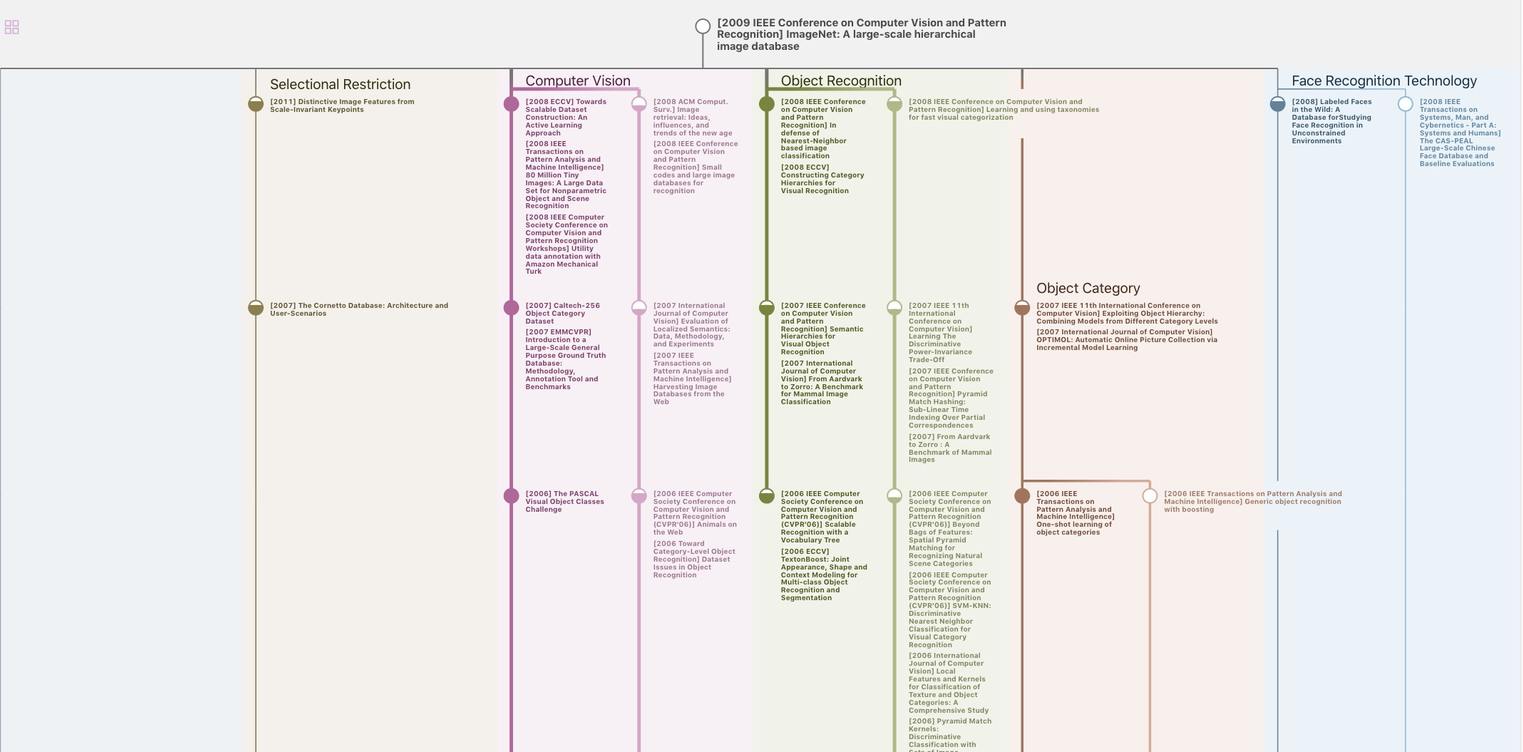
生成溯源树,研究论文发展脉络
Chat Paper
正在生成论文摘要