Deep Multi-view Learning from Sequential Data without Correspondence
2019 International Joint Conference on Neural Networks (IJCNN)(2019)
摘要
Multi-view representation learning has become an active research topic in machine learning and data mining. One underlying assumption of the conventional methods is that training data of the views must be equal in size and sample-wise matching. However, in many real-world applications, such as video analysis, text streaming, and signal processing, data for the views often come in the form of sequences, that are different in length and misaligned, resulting in the failure of directly applying existing methods to such problems. In this paper, we first introduce a novel deep multi-view model that can implicitly discover sample correspondence while learning the representation. It can be shown that our method generalizes deep canonical correlation analysis - a popular multi-view learning method. We then extend our model by integrating the objective function with the reconstruction losses of autoencoders, forming a new variant of the proposed model. Extensively experimental results demonstrate the superior performances of our models over competing methods.
更多查看译文
关键词
sequential data,multiview representation learning,machine learning,training data,sample-wise matching,deep canonical correlation analysis,data mining
AI 理解论文
溯源树
样例
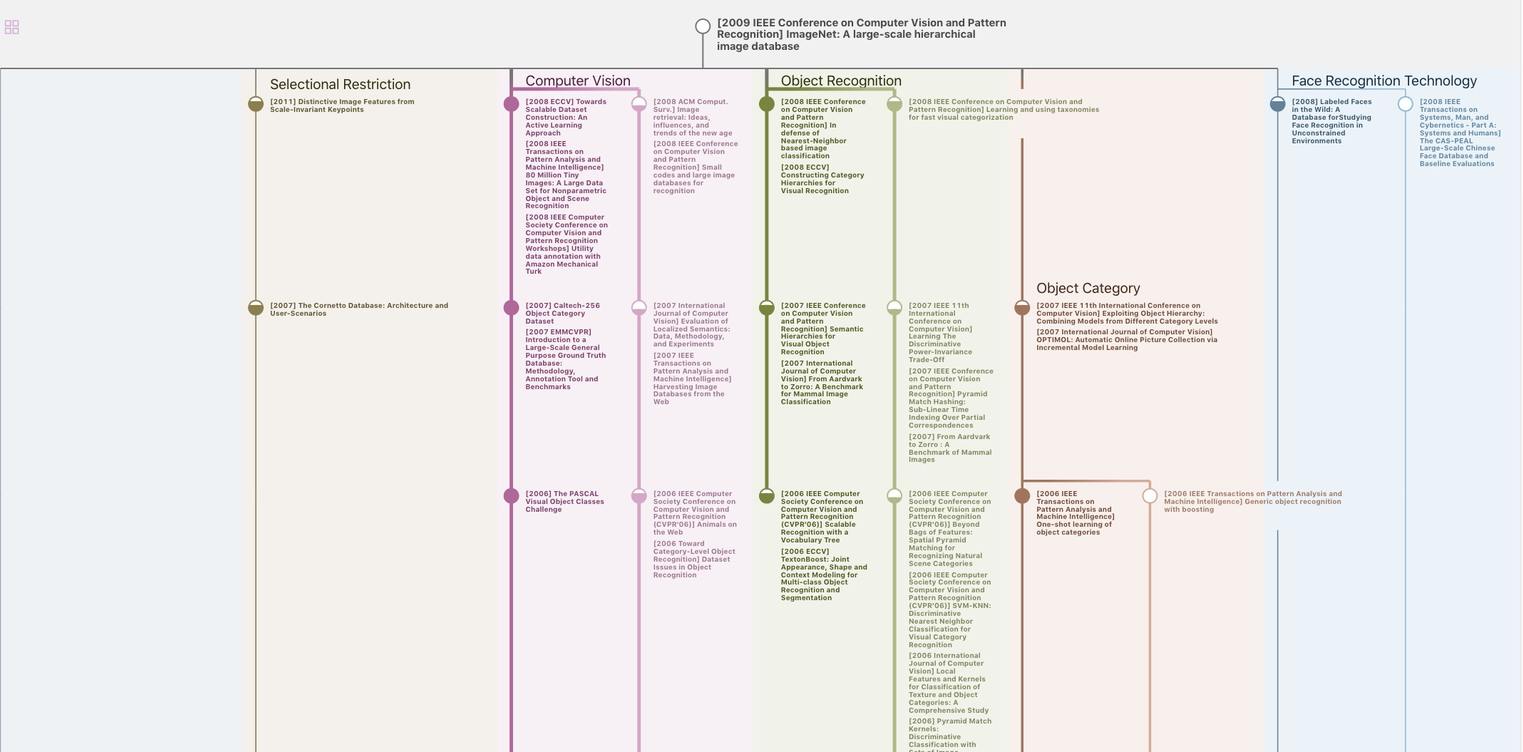
生成溯源树,研究论文发展脉络
Chat Paper
正在生成论文摘要