Using A Recurrent Kernel Learning Machine For Small-Sample Image Classification
2019 INTERNATIONAL JOINT CONFERENCE ON NEURAL NETWORKS (IJCNN)(2019)
摘要
Many machine learning algorithms, like Convolutional Neural Networks (CNNs), have excelled in image processing tasks; however, they have many practical limitations. For one, these systems require large datasets that accurately represent the sample distribution in order to optimize performance. Secondly, they have difficulty transferring previously learned knowledge when evaluating data from slightly different sample distributions. To overcome these drawbacks, we propose a recurrent kernel-based approach for image processing using the Kernel Adaptive Autoregressive Moving Average algorithm (KAARMA). KAARMA minimizes the amount of training data required by using the Reproducing Kernel Hilbert Space to build inference into the system. The recurrent nature of KAARMA additionally allows the system to better learn the spatial correlations in the images through one-shot or near oneshot learning. We demonstrate KAARMA's superiority for small-sample image classification using the JAFFE Face Dataset and the UCI hand written digit dataset.
更多查看译文
关键词
Image Classification, Recurrent Systems, Kernel Machines
AI 理解论文
溯源树
样例
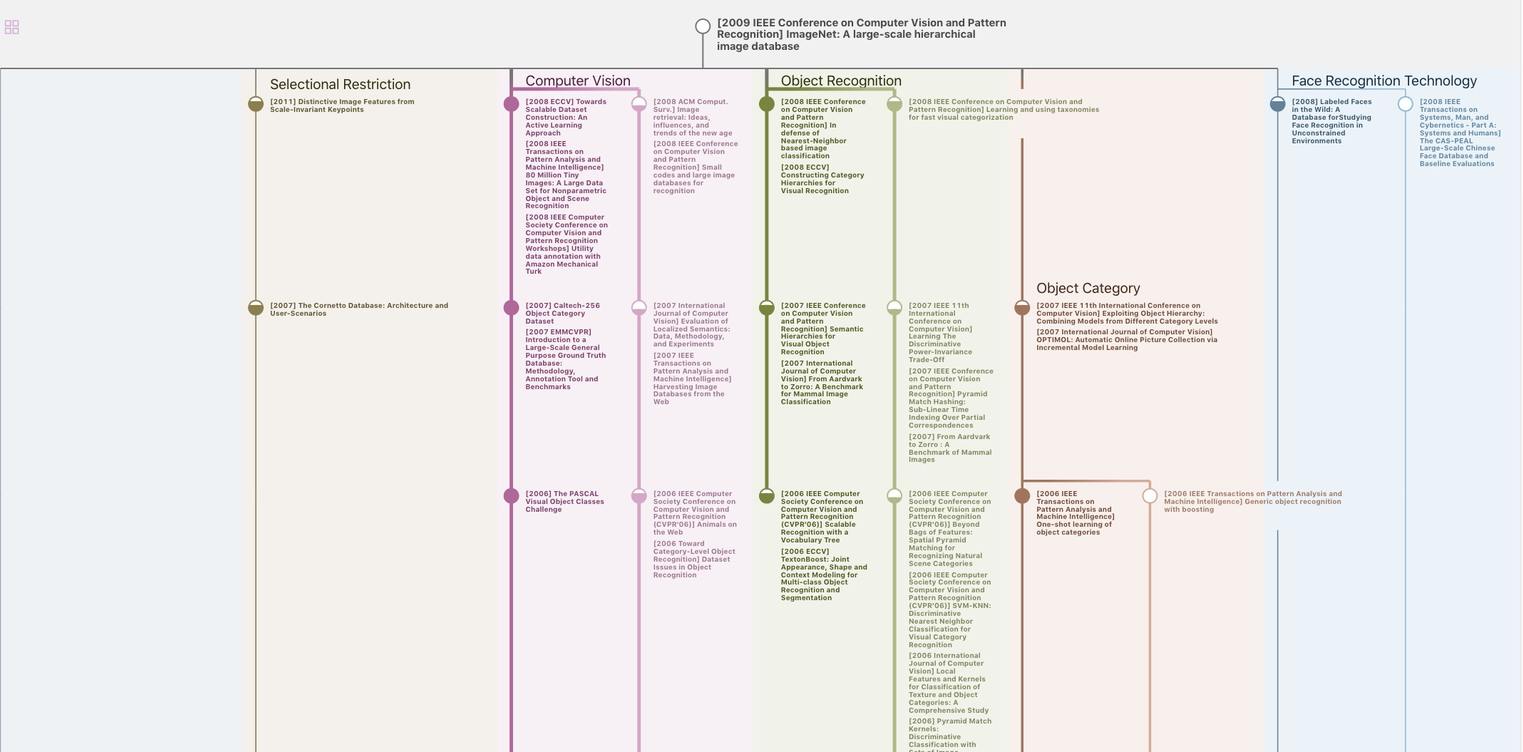
生成溯源树,研究论文发展脉络
Chat Paper
正在生成论文摘要