Playing Chess at a Human Desired Level and Style.
HAI(2019)
摘要
Human chess players prefer training with human opponents over chess agents as the latter are distinctively different in level and style than humans. Chess agents designed for human-agent play are capable of adjusting their level, however their style is not aligned with that of human players. In this paper, we propose a novel approach for designing such agents by integrating the theory of chess players' decision-making with a state-of-the-art Monte Carlo Tree Search (MCTS) algorithm. We demonstrate the benefits of our approach using two sets of analyses. Quantitatively, we establish that the agents attain their desired Elo ratings. Qualitatively, through a Turing-inspired test with a human chess expert, we show that our agents are indistinguishable from human players.
更多查看译文
关键词
Human-Agent Play, Chess, Game Playing Agents
AI 理解论文
溯源树
样例
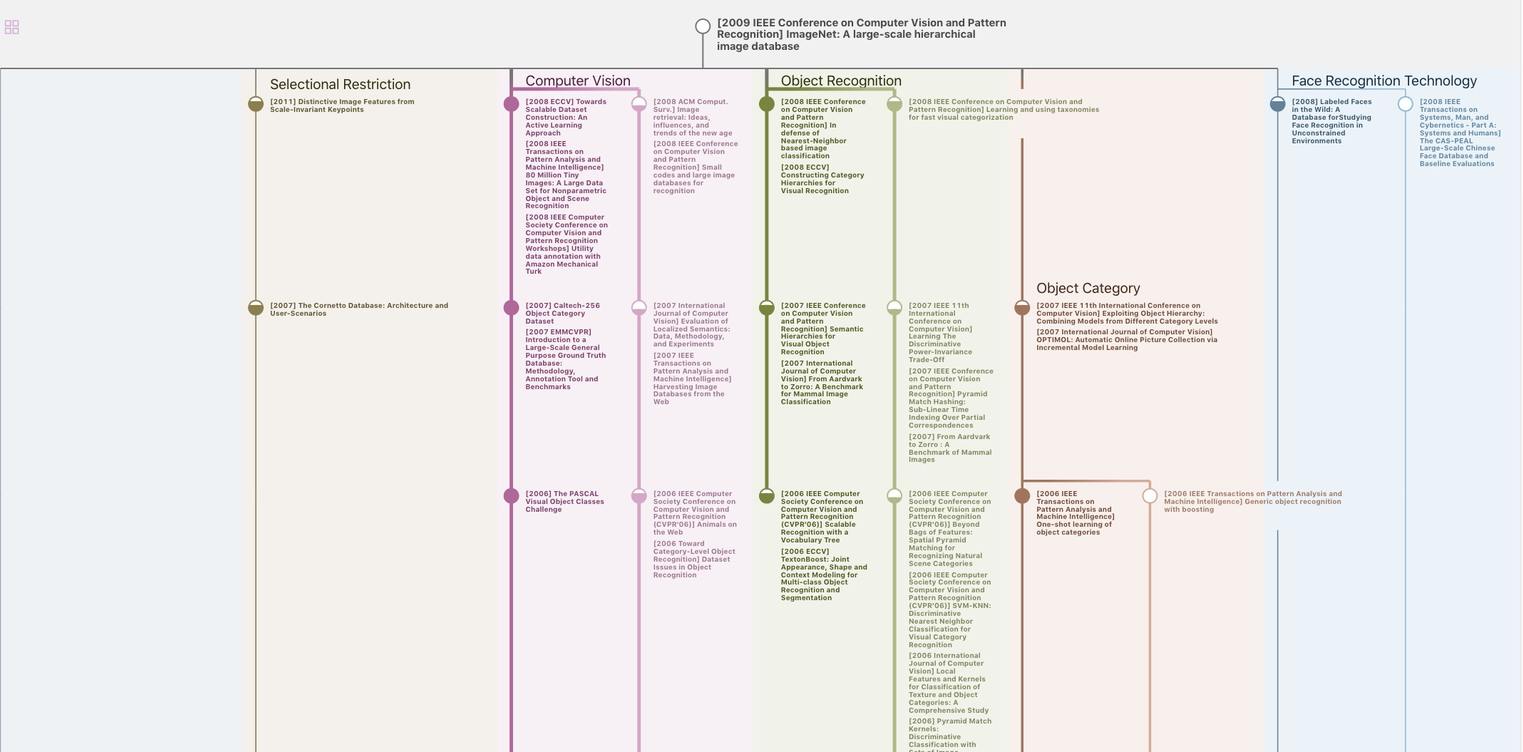
生成溯源树,研究论文发展脉络
Chat Paper
正在生成论文摘要