Slanted Stixels: A Way to Represent Steep Streets
International Journal of Computer Vision(2019)
摘要
This work presents and evaluates a novel compact scene representation based on Stixels that infers geometric and semantic information. Our approach overcomes the previous rather restrictive geometric assumptions for Stixels by introducing a novel depth model to account for non-flat roads and slanted objects. Both semantic and depth cues are used jointly to infer the scene representation in a sound global energy minimization formulation. Furthermore, a novel approximation scheme is introduced in order to significantly reduce the computational complexity of the Stixel algorithm, and then achieve real-time computation capabilities. The idea is to first perform an over-segmentation of the image, discarding the unlikely Stixel cuts, and apply the algorithm only on the remaining Stixel cuts. This work presents a novel over-segmentation strategy based on a fully convolutional network, which outperforms an approach based on using local extrema of the disparity map. We evaluate the proposed methods in terms of semantic and geometric accuracy as well as run-time on four publicly available benchmark datasets. Our approach maintains accuracy on flat road scene datasets while improving substantially on a novel non-flat road dataset.
更多查看译文
关键词
Stereo vision, Stixel world, Self-driving cars, Scene understanding, Automotive vision, Intelligent vehicles
AI 理解论文
溯源树
样例
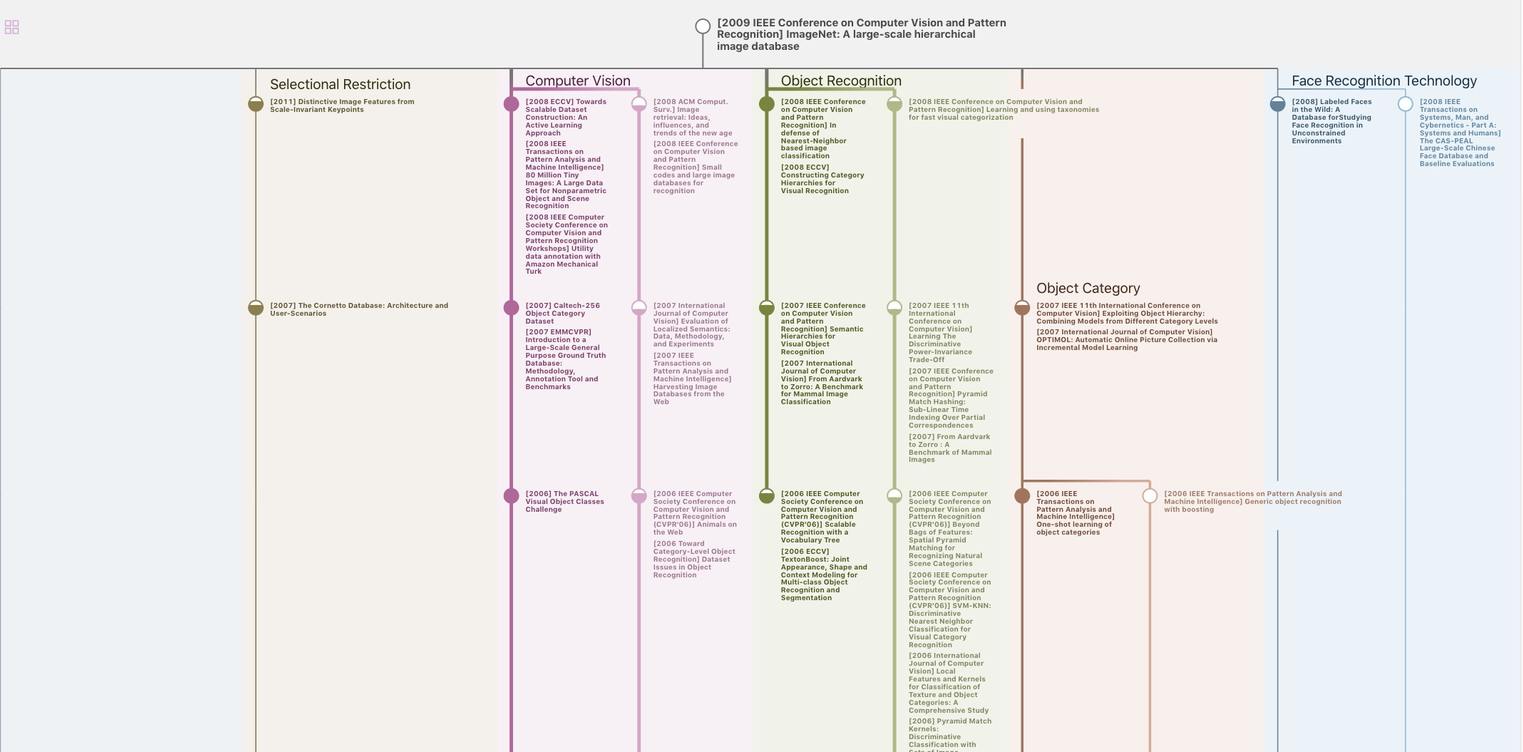
生成溯源树,研究论文发展脉络
Chat Paper
正在生成论文摘要