The effects of degrees of freedom estimation in the Asymmetric GARCH model with Student-t Innovations
arxiv(2019)
摘要
This work investigates the effects of using the independent Jeffreys prior for the degrees of freedom parameter of a Student-t model in the asymmetric generalised autoregressive conditional heteroskedasticity (GARCH) model. To capture asymmetry in the reaction to past shocks, smooth transition models are assumed for the variance. We adopt the fully Bayesian approach for inference, prediction and model selection We discuss problems related to the estimation of degrees of freedom in the Student-t model and propose a solution based on independent Jeffreys priors which correct problems in the likelihood function. A simulated study is presented to investigate how the estimation of model parameters in the Student-t GARCH model are affected by small sample sizes, prior distributions and misspecification regarding the sampling distribution. An application to the Dow Jones stock market data illustrates the usefulness of the asymmetric GARCH model with Student-t errors.
更多查看译文
AI 理解论文
溯源树
样例
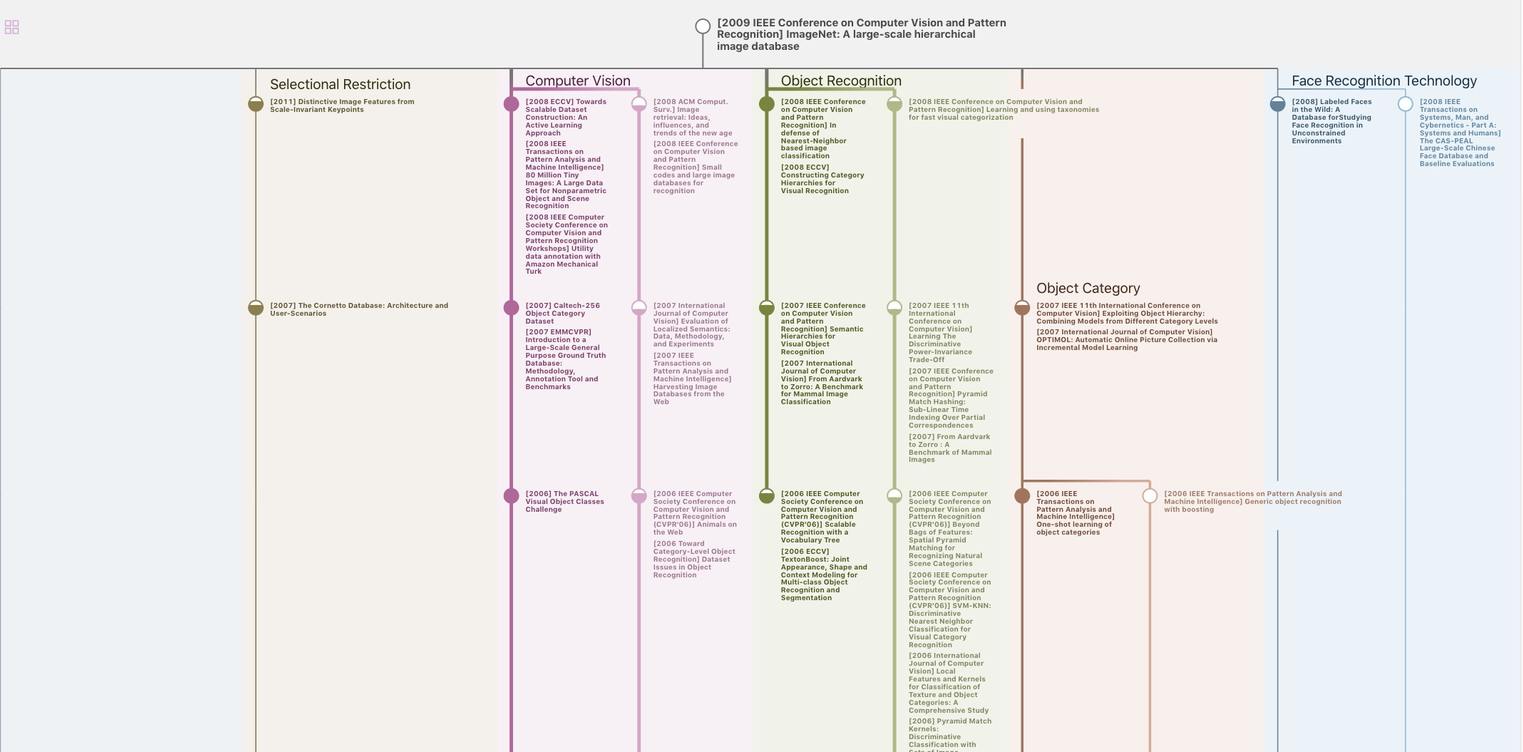
生成溯源树,研究论文发展脉络
Chat Paper
正在生成论文摘要