Nonnegative matrix tri-factorization with user similarity for clustering in point-of-interest.
Neurocomputing(2019)
摘要
With the widespread use of Location-based Social Networks (LBSNs), massive Point-of-Interest (POI) data continuously generated by users. POI clustering is an essential foundation for efficiently processing large amounts of POI data. However, the majority of existing studies only consider artificial labels and geographic information for clustering POIs and rarely take account of the characteristics of user behavior. The main challenge of POI clustering is lack of label information at present. To address the issues above, we propose a method of collaborative clustering based on Nonnegative Matrix Tri-factorization for POI (POI-NMTF), which combines the similarity of users based on time and location by exploiting the user check-in data in our study. Our algorithm provides a co-clustering method that allows clustering users and POIs simultaneously thereby discover the potential preference of users. Moreover, it can also better reflect the multiple interest attributes of users for a single POI, because our algorithm is a soft clustering method. We test our method using real dataset, and the experimental results show the validity and correctness of our algorithm, the clustering result is superior to other compared methods.
更多查看译文
关键词
Point of interest (POI),Nonnegative matrix tri-factorization,Clustering,User similarity
AI 理解论文
溯源树
样例
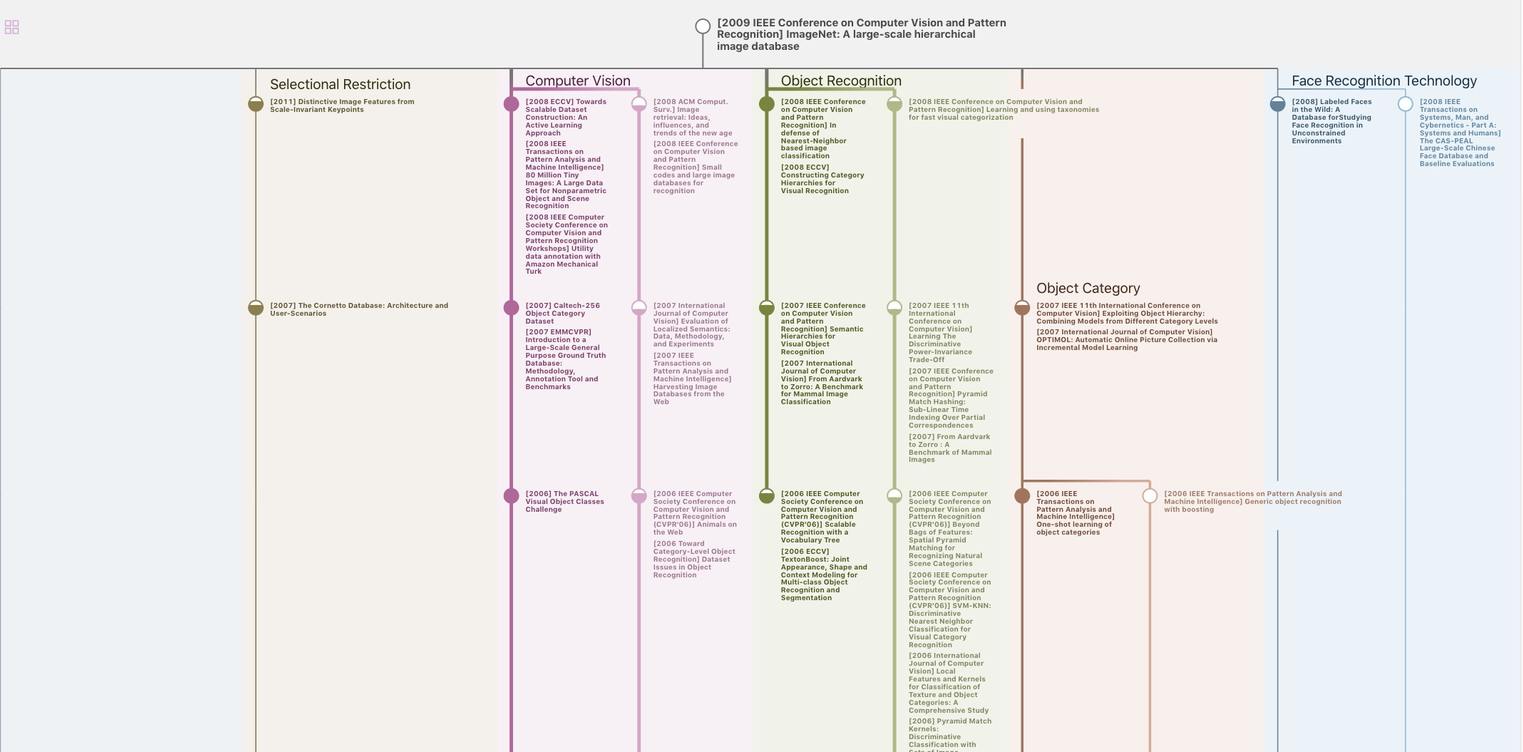
生成溯源树,研究论文发展脉络
Chat Paper
正在生成论文摘要