Integrated Learning Of Features And Ranking Function In Information Retrieval
PROCEEDINGS OF THE 2019 ACM SIGIR INTERNATIONAL CONFERENCE ON THEORY OF INFORMATION RETRIEVAL (ICTIR'19)(2019)
摘要
Recent deep learning models for information retrieval typically aim to learn features either about the contents of the document and the query, or about the interactions between them. However, the existing literature shows that document ranking depends simultaneously on many factors, including both content and interaction features. The integration of both types of neural features has not been extensively studied. In addition, many studies have also shown that the deep neural features cannot replace completely the traditional features, but are complementary. It is thus reasonable to combine deep neural features with traditional features. In this paper, we propose an integrated end-to-end learning framework based on learning-to-rank (L2R) to learn both neural features and the L2R ranking function simultaneously. The framework also has the flexibility to integrate arbitrary traditional features. Our experiments on public datasets confirm that such an integrated learning strategy is better than separate learning of features and ranking function, and integrating traditional features can further improve the results.
更多查看译文
关键词
Information Retrieval, Neural Network, Ranking
AI 理解论文
溯源树
样例
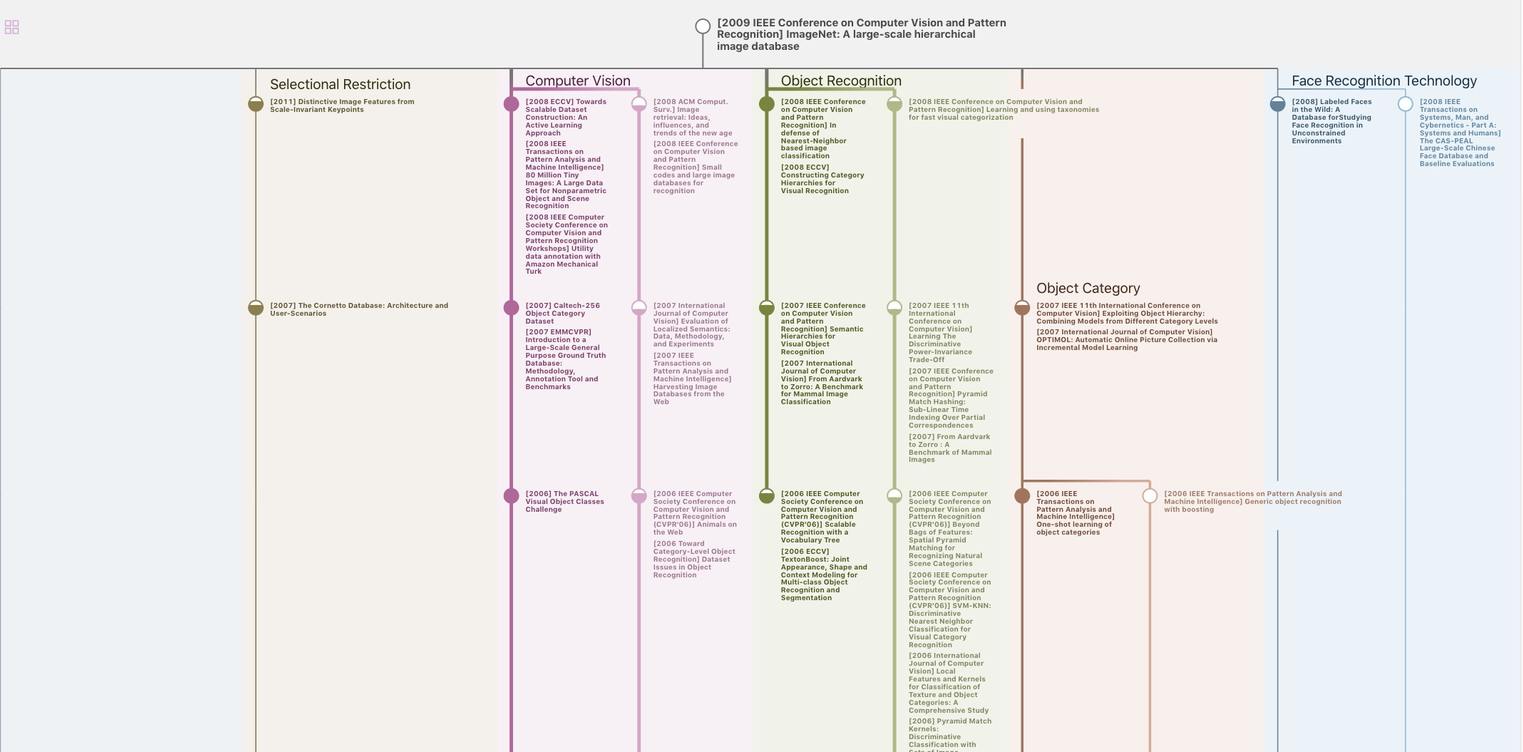
生成溯源树,研究论文发展脉络
Chat Paper
正在生成论文摘要