Symplectic Recurrent Neural Networks
ICLR(2020)
摘要
We propose Symplectic Recurrent Neural Networks (SRNNs) as learning algorithms that capture the dynamics of physical systems from observed trajectories. SRNNs model the Hamiltonian function of the system by a neural networks, and leverage symplectic integration, multiple-step training and initial state optimization to address the challenging numerical issues associated with Hamiltonian systems. We show SRNNs succeed reliably on complex and noisy Hamiltonian systems. Finally, we show how to augment the SRNN integration scheme in order to handle stiff dynamical systems such as bouncing billiards.
更多查看译文
关键词
Hamiltonian systems, learning physical laws, symplectic integrators, recurrent neural networks, inverse problems
AI 理解论文
溯源树
样例
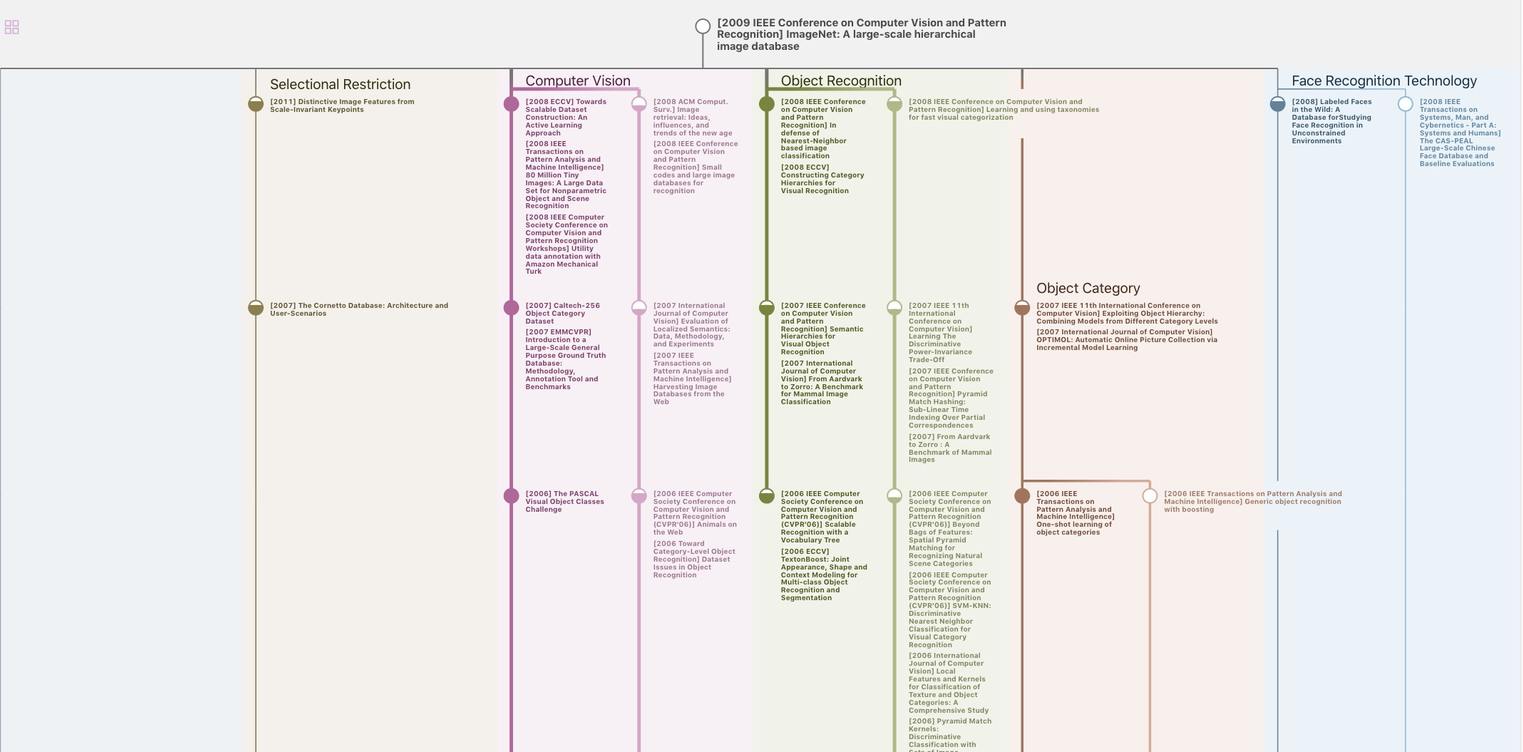
生成溯源树,研究论文发展脉络
Chat Paper
正在生成论文摘要