RPM-Net: Robust Pixel-Level Matching Networks for Self-Supervised Video Object Segmentation
arxiv(2019)
摘要
In this paper, we introduce a self-supervised approach for video object segmentation without human labeled data.Specifically, we present Robust Pixel-level Matching Net-works (RPM-Net), a novel deep architecture that matches pixels between adjacent frames, using only color information from unlabeled videos for training. Technically, RPM-Net can be separated in two main modules. The embed-ding module first projects input images into high dimensional embedding space. Then the matching module with deformable convolution layers matches pixels between reference and target frames based on the embedding features.Unlike previous methods using deformable convolution, our matching module adopts deformable convolution to focus on similar features in spatio-temporally neighboring pixels.Our experiments show that the selective feature sampling improves the robustness to challenging problems in video object segmentation such as camera shake, fast motion, deformation, and occlusion. Also, we carry out comprehensive experiments on three public datasets (i.e., DAVIS-2017,SegTrack-v2, and Youtube-Objects) and achieve state-of-the-art performance on self-supervised video object seg-mentation. Moreover, we significantly reduce the performance gap between self-supervised and fully-supervised video object segmentation (41.0% vs. 52.5% on DAVIS-2017 validation set)
更多查看译文
关键词
robust pixel-level matching networks,self-supervised video object segmentation,RPM-Net,unlabeled videos,high dimensional embedding space,matching module,deformable convolution layers,embedding features,fully-supervised video object segmentation,DAVIS-2017 dataset,SegTrack-v2 dataset,Youtube-Objects dataset
AI 理解论文
溯源树
样例
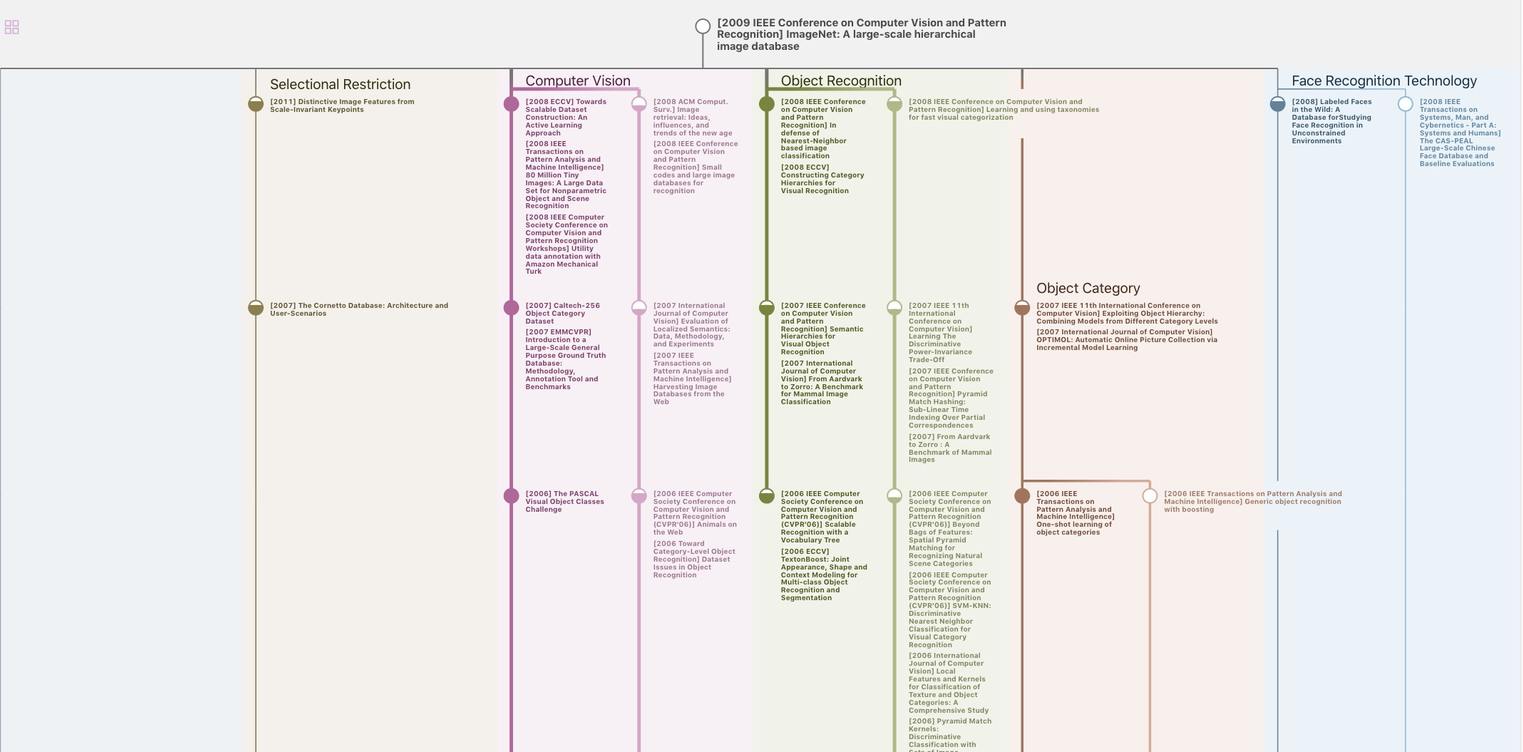
生成溯源树,研究论文发展脉络
Chat Paper
正在生成论文摘要