Grouped Spatial-Temporal Aggregation For Efficient Action Recognition
2019 IEEE/CVF INTERNATIONAL CONFERENCE ON COMPUTER VISION (ICCV 2019)(2019)
摘要
Temporal reasoning is an important aspect of video analysis. 3D CNN shows good performance by exploring spatial-temporal features jointly in an unconstrained way, but it also increases the computational cost a lot. Previous works try to reduce the complexity by decoupling the spatial and temporal filters. In this paper, we propose a novel decomposition method that decomposes the feature channels into spatial and temporal groups in parallel. This decomposition can make two groups focus on static and dynamic cues separately. We call this grouped spatial-temporal aggregation (GST). This decomposition is more parameter-efficient and enables us to quantitatively analyze the contributions of spatial and temporal features in different layers. We verify our model on several action recognition tasks that require temporal reasoning and show its effectiveness.
更多查看译文
关键词
grouped spatial-temporal aggregation,efficient action recognition,temporal reasoning,spatial-temporal features,spatial filters,temporal filters,spatial groups,temporal groups,spatial features,GST,3D CNN
AI 理解论文
溯源树
样例
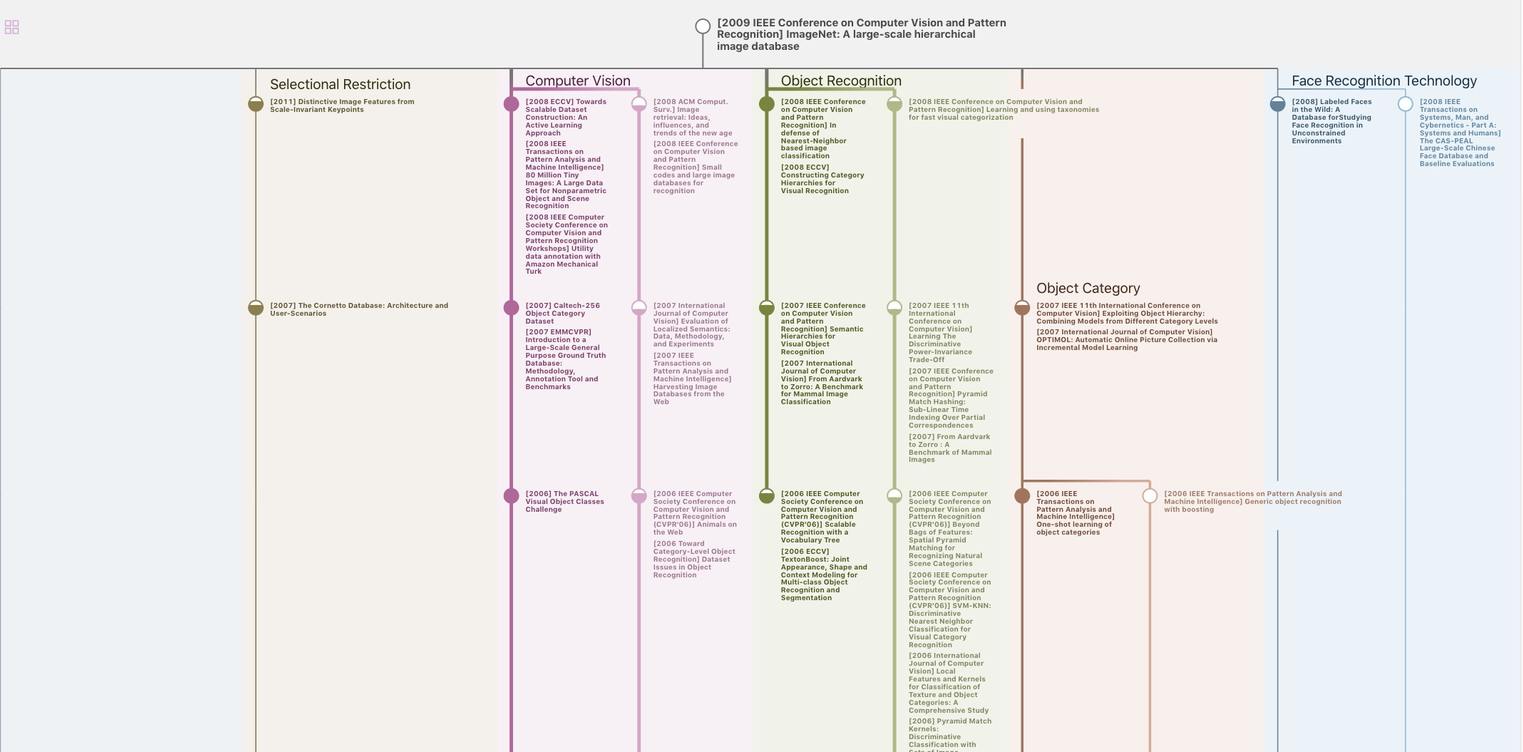
生成溯源树,研究论文发展脉络
Chat Paper
正在生成论文摘要