Mip-Boost: Efficient And Effective L-0 Feature Selection For Linear Regression
JOURNAL OF COMPUTATIONAL AND GRAPHICAL STATISTICS(2021)
摘要
Recent advances in mathematical programming have made mixed integer optimization a competitive alternative to popular regularization methods for selecting features in regression problems. The approach exhibits unquestionable foundational appeal and versatility, but also poses important challenges. Here, we propose MIP-BOOST, a revision of standard mixed integer programming feature selection that reduces the computational burden of tuning the critical sparsity bound parameter and improves performance in the presence of feature collinearity and of signals that vary in nature and strength. The final outcome is a more efficient and effective L-0 feature selection method for applications of realistic size and complexity, grounded on rigorous cross-validation tuning and exact optimization of the associated mixed integer program. Computational viability and improved performance in realistic scenarios is achieved through three independent but synergistic proposals. including additional results, pseudocode, and computer code are available online.
更多查看译文
关键词
Cross-validation, Feature selection, LASSO, Mixed integer optimization, Regression, Whitening
AI 理解论文
溯源树
样例
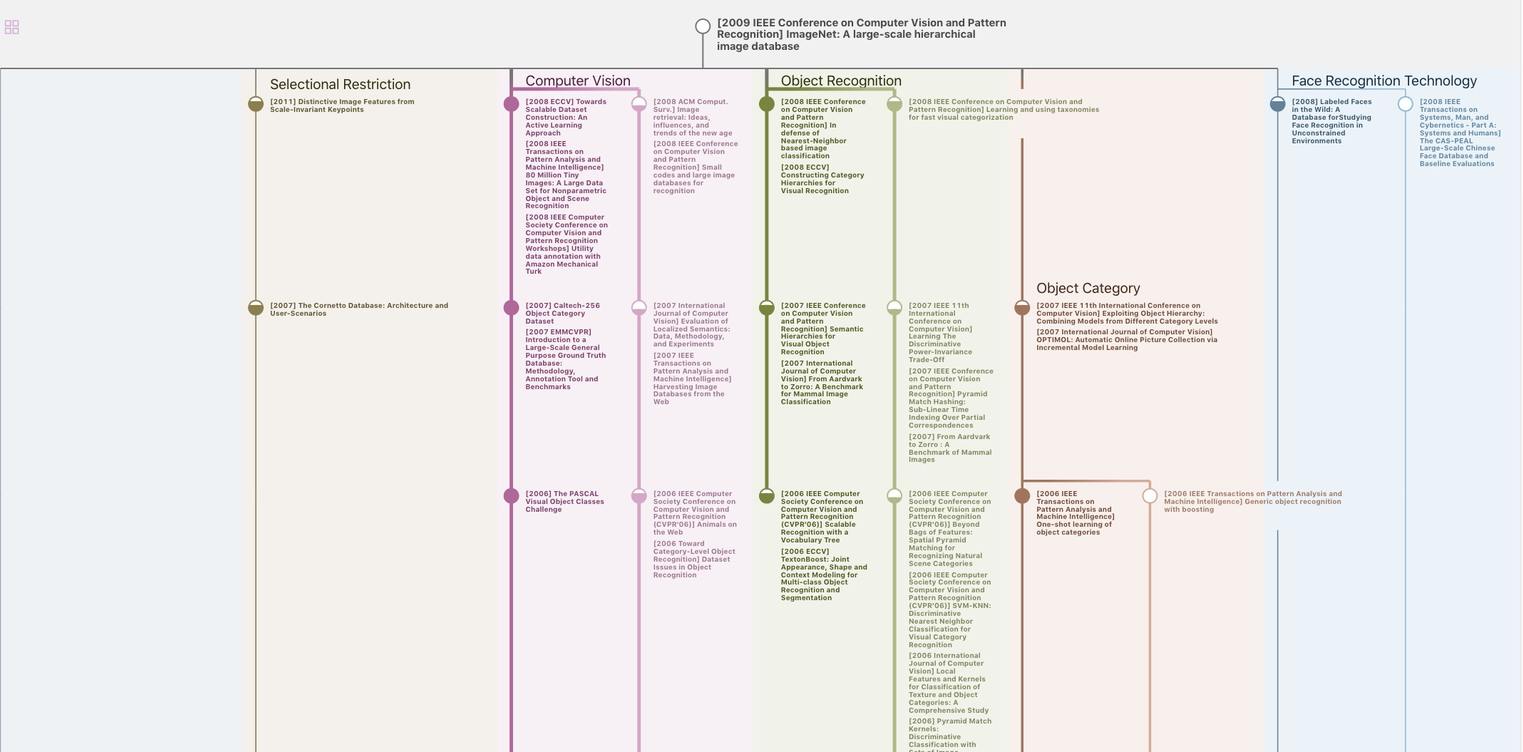
生成溯源树,研究论文发展脉络
Chat Paper
正在生成论文摘要