A Human-Machine Adversarial Scoring Framework For Urban Perception Assessment Using Street-View Images
INTERNATIONAL JOURNAL OF GEOGRAPHICAL INFORMATION SCIENCE(2019)
摘要
Though global-coverage urban perception datasets have been recently created using machine learning, their efficacy in accurately assessing local urban perceptions for other countries and regions remains a problem. Here we describe a human-machine adversarial scoring framework using a methodology that incorporates deep learning and iterative feedback with recommendation scores, which allows for the rapid and cost-effective assessment of the local urban perceptions for Chinese cities. Using the state-of-the-art Fully Convolutional Network (FCN) and Random Forest (RF) algorithms, the proposed method provides perception estimations with errors less than 10%. The driving factor analysis from both the visual and urban functional aspects demonstrated its feasibility in facilitating local urban perception derivations. With high-throughput and high-accuracy scorings, the proposed human-machine adversarial framework offers an affordable and rapid solution for urban planners and researchers to conduct local urban perception assessments.
更多查看译文
关键词
Street view, urban perception, deep learning, urban planning, human-machine adversarial scoring
AI 理解论文
溯源树
样例
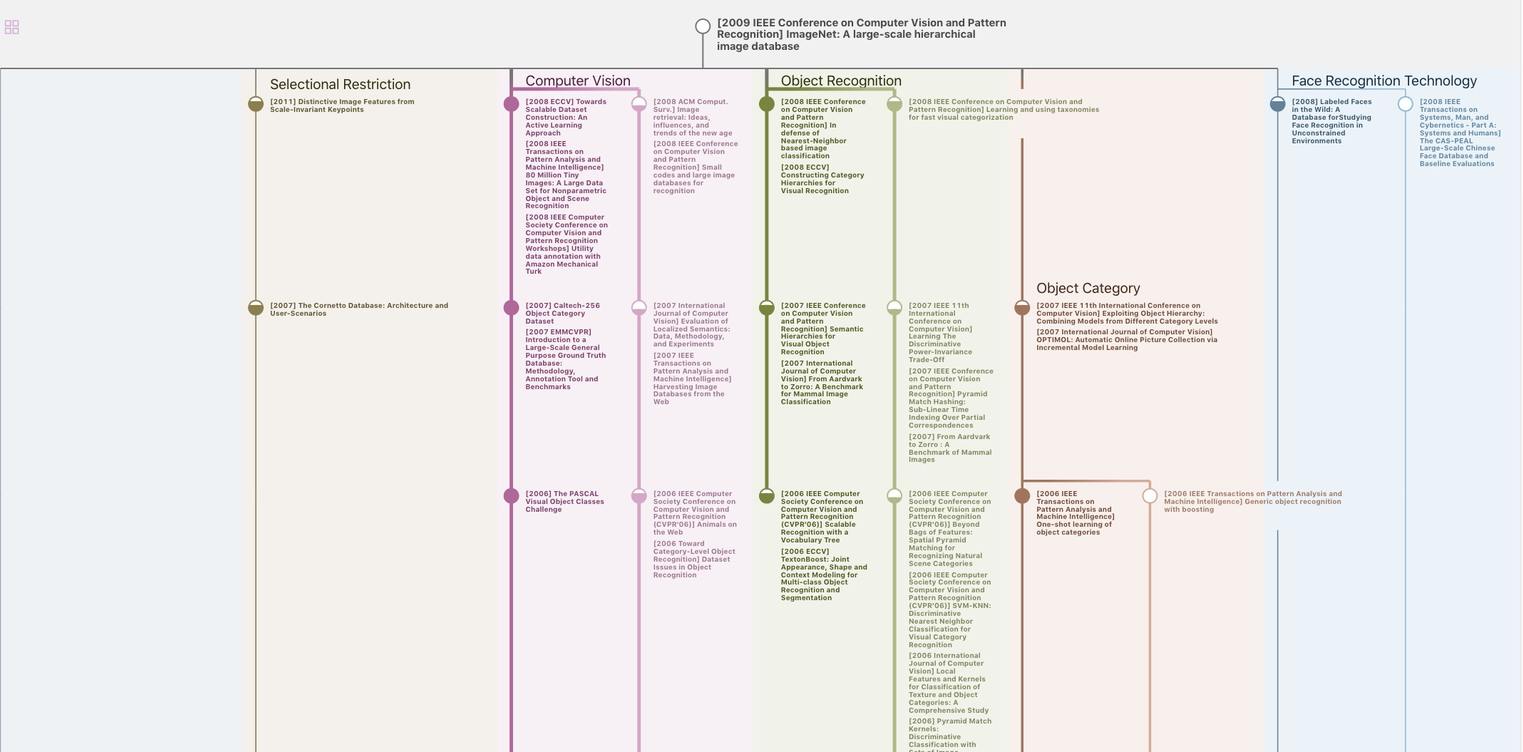
生成溯源树,研究论文发展脉络
Chat Paper
正在生成论文摘要