50 Fps Object-Level Saliency Detection Via Maximally Stable Region
IEEE TRANSACTIONS ON IMAGE PROCESSING(2020)
摘要
The human visual system tends to consider saliency of an object as a whole. Some object-level saliency detection methods have been proposed by leveraging object proposals in bounding boxes, and regarding the entire bounding box as one candidate salient region. However, the bounding boxes can not provide exact object position and a lot of pixels in bounding boxes belong to the background. Consequently, background pixels in bounding box also show high saliency. Besides, acquiring object proposals needs high time cost. In order to compute object-level saliency, we consider region growing from some seed superpixels, to find one surrounding region which probably represents the whole object. The desired surrounding region has similar appearance inside and obvious difference with the outside, which is proposed as maximally stable region (MSR ) in this paper. In addition, one effective seed superpixel selection strategy is presented to improve speed. MSR based saliency detection is more robust than pixel or superpixel level methods and object proposal based methods. The proposed method significantly outperforms the state-of-the-art unsupervised methods at 50 FPS. Compared with deep learning based methods, we show worse performance, but with about 12001600 times faster, which means better trade-off between performance and speed.
更多查看译文
关键词
Saliency detection, Proposals, Object detection, Graphical models, Visual systems, Deep learning, Visualization, Saliency detection, salient object detection, maximally stable region, minimum barrier distance, seed selection
AI 理解论文
溯源树
样例
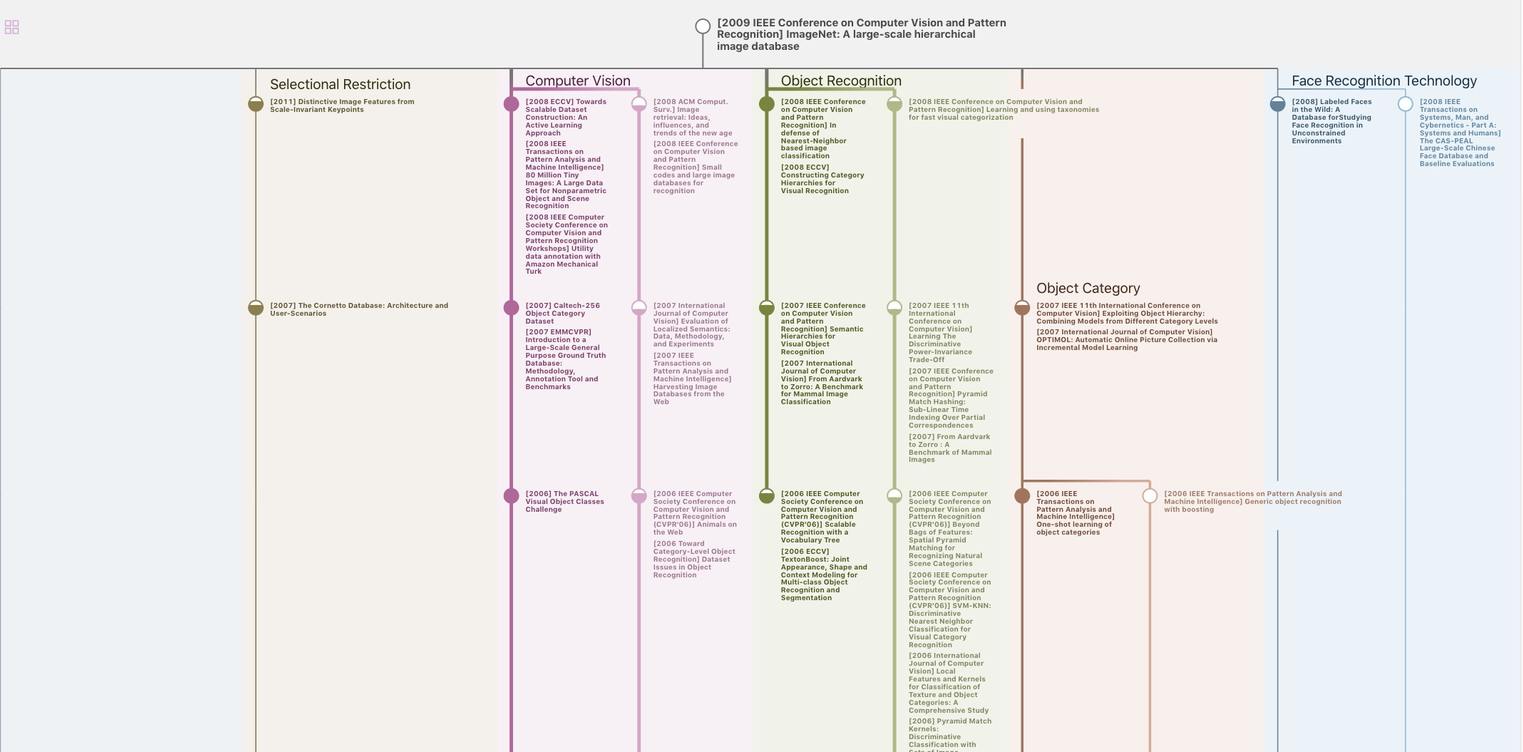
生成溯源树,研究论文发展脉络
Chat Paper
正在生成论文摘要