Elastic Deep Learning in Multi-Tenant GPU Clusters
IEEE Transactions on Parallel and Distributed Systems(2022)
摘要
We study how to support elasticity, that is, the ability to dynamically adjust the parallelism (i.e., the number of GPUs), for deep neural network (DNN) training in a GPU cluster. Elasticity can benefit multi-tenant GPU cluster management in many ways, for example, achieving various scheduling objectives (e.g., job throughput, job completion time, GPU efficiency) according to cluster load variations, utilizing transient idle resources, and supporting performance profiling, job migration, and straggler mitigation. We propose EDL, which enables elastic deep learning with a simple API and can be easily integrated with existing deep learning frameworks such as TensorFlow and PyTorch. EDL also incorporates techniques that are necessary to reduce the overhead of parallelism adjustments, such as stop-free scaling and dynamic data pipeline. We demonstrate with experiments that EDL can indeed bring significant benefits to the above-listed applications in GPU cluster management.
更多查看译文
关键词
Deep learning system,elastic deep learning,GPU cluster management
AI 理解论文
溯源树
样例
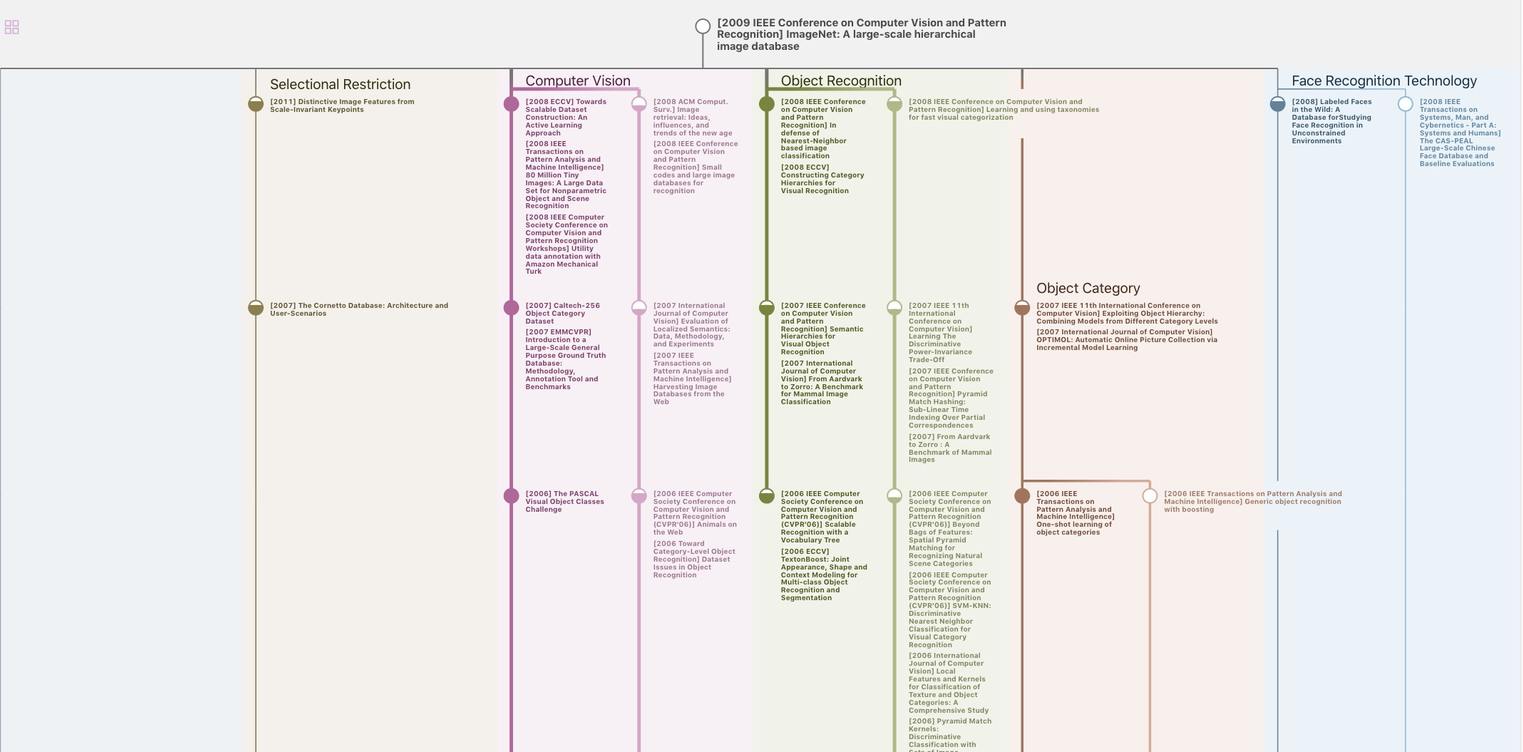
生成溯源树,研究论文发展脉络
Chat Paper
正在生成论文摘要