Multi-Grained Attention Networks for Single Image Super-Resolution
IEEE Transactions on Circuits and Systems for Video Technology(2021)
摘要
Deep Convolutional Neural Networks (CNN) have drawn great attention in image super-resolution (SR). Recently, visual attention mechanism, which exploits both of the feature importance and contextual cues, has been introduced to image SR and proves to be effective to improve CNN-based SR performance. In this paper, we make a thorough investigation on the attention mechanisms in a SR model and shed light on how simple and effective improvements on these ideas improve the state-of-the-arts. We further propose a unified approach called “multi-grained attention networks (MGAN)” which fully exploits the advantages of multi-scale and attention mechanisms in SR tasks. In our method, the importance of each neuron is computed according to its surrounding regions in a multi-grained fashion and then is used to adaptively re-scale the feature responses. More importantly, the “channel attention” and “spatial attention” strategies in previous methods can be essentially considered as two special cases of our method. We also introduce multi-scale dense connections to extract the image features at multiple scales and capture the features of different layers through dense skip connections. Ablation studies on benchmark datasets demonstrate the effectiveness of our method. In comparison with other state-of-the-art SR methods, our method shows the superiority in terms of both accuracy and model size.
更多查看译文
关键词
Super-resolution,convolutional neural networks,multi-grained attention,multi-scale dense connections
AI 理解论文
溯源树
样例
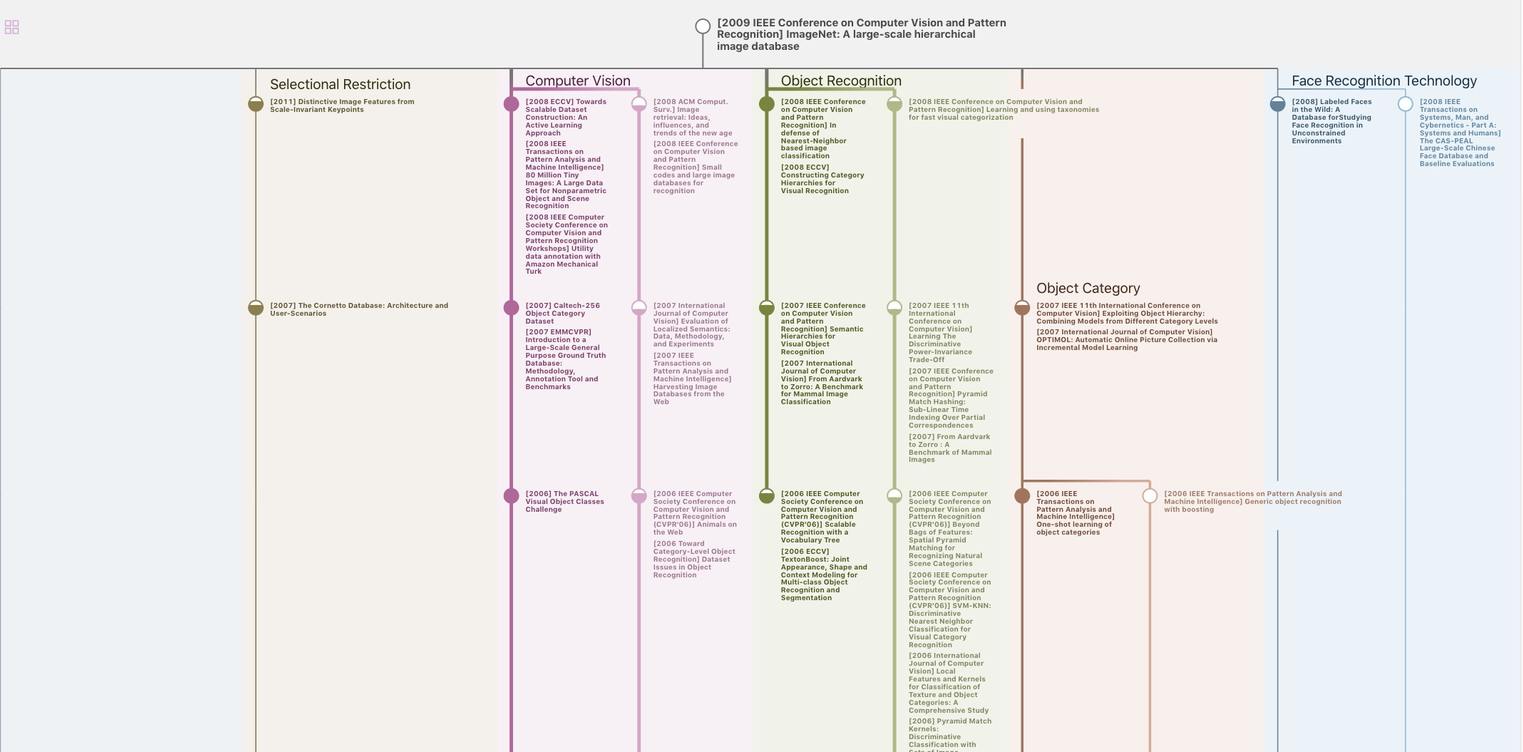
生成溯源树,研究论文发展脉络
Chat Paper
正在生成论文摘要