An Adaptive Interval Forecast Cnn Model For Fault Detection Method
2019 IEEE 15TH INTERNATIONAL CONFERENCE ON AUTOMATION SCIENCE AND ENGINEERING (CASE)(2019)
摘要
The machine fault detection (MFD) is critical for the safety operation of the petrochemical production. Aiming to automatically optimizing the pre-warning bounds of the control chart, an interval forecasting convolutional neural network (IFCNN) model has been proposed to forecast the warning interval of the signal with the raw dynamic data. Essentially, the IFCNN model is an improved convolutional neural network with dual output value to construct the warning interval directly and adaptively. To guide the model to learn the interval automatically during the model training, the loss function is customized to improve the fault detection accuracy. The proposed method is compared with the fixed threshold and the adaptive interval method with exponentially weighted moving average on a petrochemical equipment data set. The results indicated that the proposed method is of stronger robustness with lower failure rate in the fault detection of the petrochemical pump.
更多查看译文
关键词
adaptive interval forecast CNN model,fault detection method,machine fault detection,safety operation,petrochemical production,pre-warning bounds,control chart,interval forecasting convolutional neural network model,warning interval,raw dynamic data,IFCNN model,improved convolutional neural network,dual output value,model training,fault detection accuracy,adaptive interval method,petrochemical equipment data
AI 理解论文
溯源树
样例
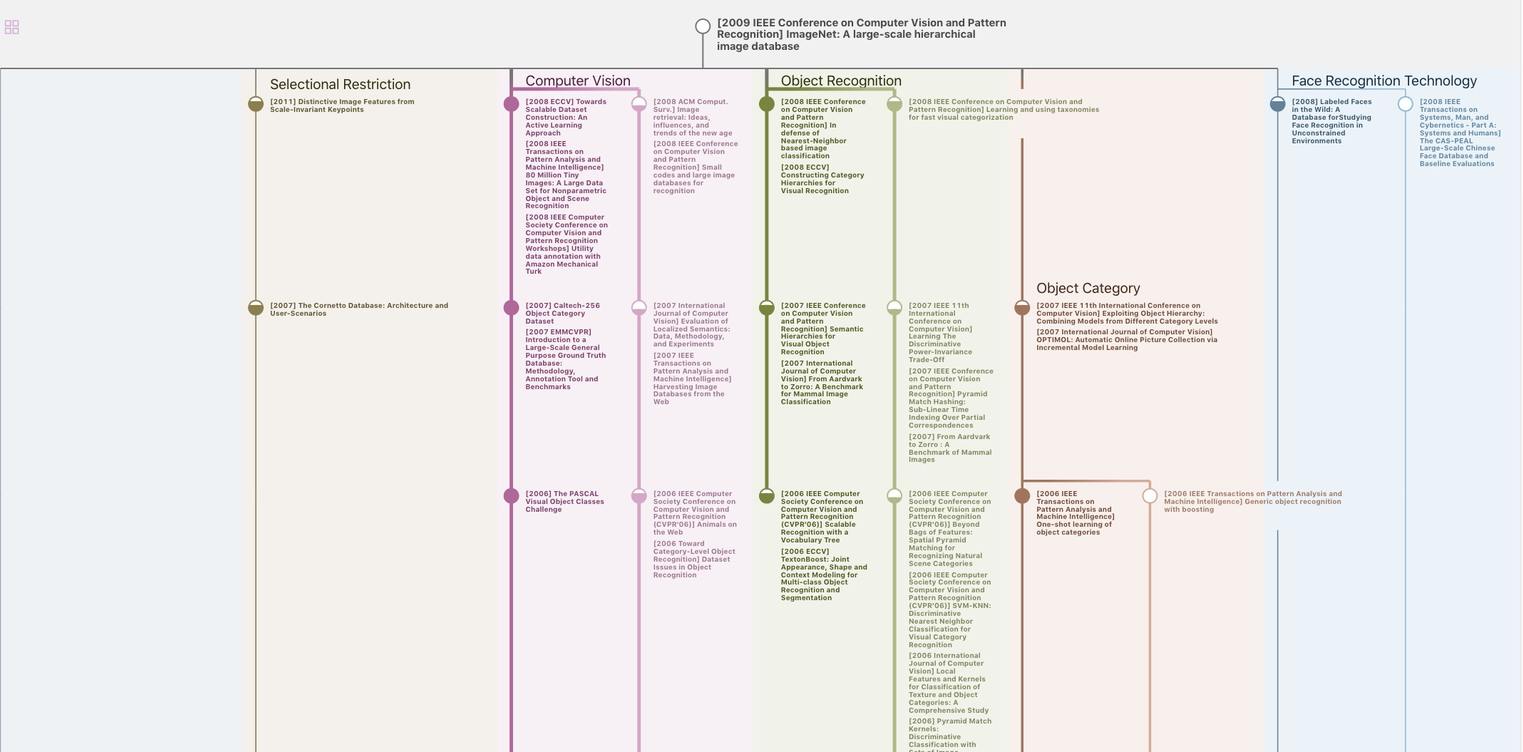
生成溯源树,研究论文发展脉络
Chat Paper
正在生成论文摘要