Improving CNN-based Planar Object Detection with Geometric Prior Knowledge
arxiv(2019)
摘要
In this paper, we focus on the question: how might mobile robots take advantage of affordable RGB-D sensors for object detection? Although current CNN-based object detectors have achieved impressive results, there are three main drawbacks for practical usage on mobile robots: 1) It is hard and time-consuming to collect and annotate large-scale training sets. 2) It usually needs a long training time. 3) CNN-based object detection shows significant weakness in predicting location. We propose a novel approach for the detection of planar objects, which rectifies images with geometric information to compensate for the perspective distortion before feeding it to the CNN detector module, typically a CNN-based detector like YOLO or MASK RCNN. By dealing with the perspective distortion in advance, we eliminate the need for the CNN detector to learn that. Experiments show that this approach significantly boosts the detection performance. Besides, it effectively reduces the number of training images required. In addition to the novel detection framework proposed, we also release an RGB-D dataset for hazmat sign detection. To the best of our knowledge, this is the first public-available hazmat sign detection dataset with RGB-D sensors.
更多查看译文
关键词
CNN-based planar object detection,mobile robots,RGB-D sensors,current CNN-based object detectors,large-scale training sets,long training time,planar objects,geometric information,perspective distortion,CNN detector module,detection performance,training images,CNN-based object detection,public-available hazmat sign detection dataset
AI 理解论文
溯源树
样例
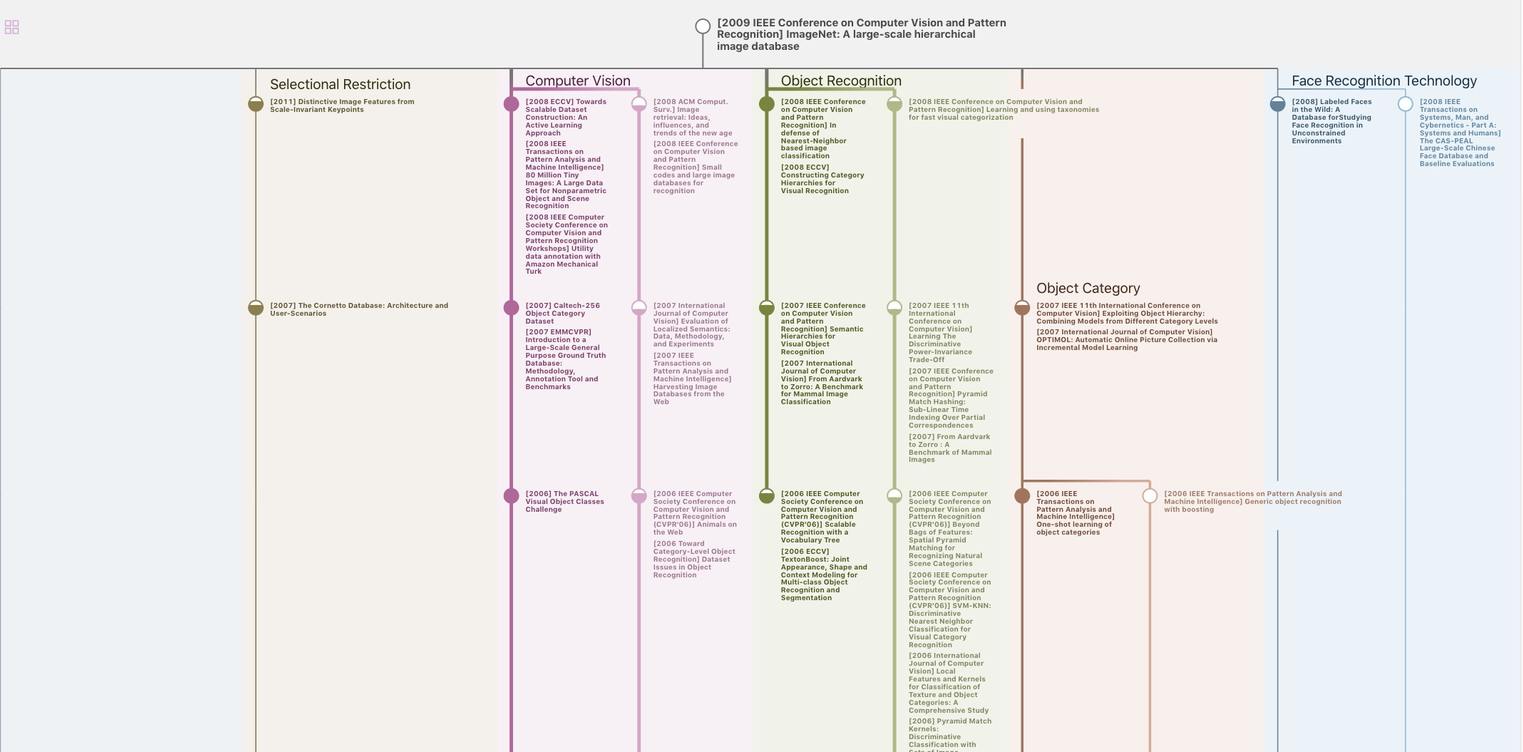
生成溯源树,研究论文发展脉络
Chat Paper
正在生成论文摘要