Cross-Dataset Person Re-Identification via Unsupervised Pose Disentanglement and Adaptation
2019 IEEE/CVF INTERNATIONAL CONFERENCE ON COMPUTER VISION (ICCV 2019)(2019)
摘要
Person re-identification (re-ID) aims at recognizing the same person from images taken across different cameras. To address this challenging task, existing re-ID models typically rely on a large amount of labeled training data, which is not practical for real-world applications. To alleviate this limitation, researchers now targets at cross-dataset re-ID which focuses on generalizing the discriminative ability to the unlabeled target domain when given a labeled source domain dataset. To achieve this goal, our proposed Pose Disentanglement and Adaptation Network (PDA-Net) aims at learning deep image representation with pose and domain information properly disentangled. With the learned cross-domain pose invariant feature space, our proposed PDA-Net is able to perform pose disentanglement across domains without supervision in identities, and the resulting features can be applied to cross-dataset re-ID. Both of our qualitative and quantitative results on two benchmark datasets confirm the effectiveness of our approach and its superiority over the state-of-the-art cross-dataset Re-ID approaches.
更多查看译文
关键词
cross-dataset person,re-ID models,labeled training data,real-world applications,discriminative ability,unlabeled target domain,labeled source domain dataset,Adaptation Network,PDA-Net,deep image representation,pose domain information,learned cross-domain,invariant feature space,benchmark datasets,Re-ID approaches,pose domain information,unsupervised pose disentanglement
AI 理解论文
溯源树
样例
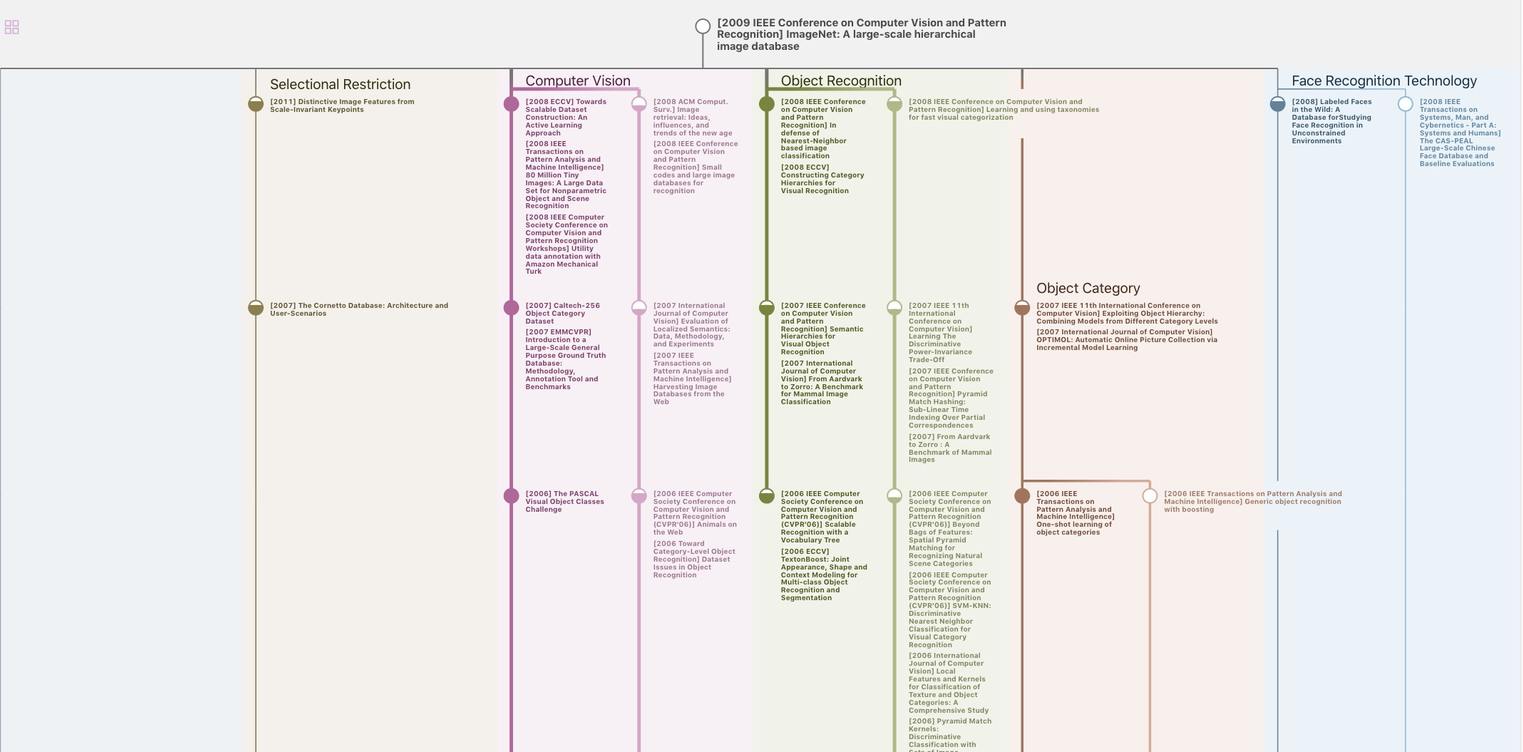
生成溯源树,研究论文发展脉络
Chat Paper
正在生成论文摘要