Automated active fault detection in fouled dissolved oxygen sensors.
Water research(2019)
摘要
Biofilm formation causes bias in dissolved oxygen (DO) sensors, which hamper their usage for automatic control and thereby balancing energy- and treatment efficiency. We analysed if a dataset that was generated with deliberate perturbations, can automatically be interpreted to detect bias caused by biofilm formation. We used a challenging set-up with realistic conditions that are required for a full-scale application. This included automated training (adapting to changing normal conditions) and automated tuning (setting an alarm threshold) to assure that the fault detection (FD)-methods are accessible to the operators. The results showed that automatic usage of FD-methods is difficult, especially in terms of automatic tuning of alarm thresholds when small training datasets only represent the normal conditions, i.e. clean sensors. Despite the challenging set-up, two FD-methods successfully improved the detection limit to 0.5 mg DO/L bias caused by biofilm formation. We showed that the studied dataset could be interpreted equally well by simpler FD-methods, as by advanced machine learning algorithms. This in turn indicates that the information contained in the actively generated data was more vital than its interpretation by advanced algorithms.
更多查看译文
AI 理解论文
溯源树
样例
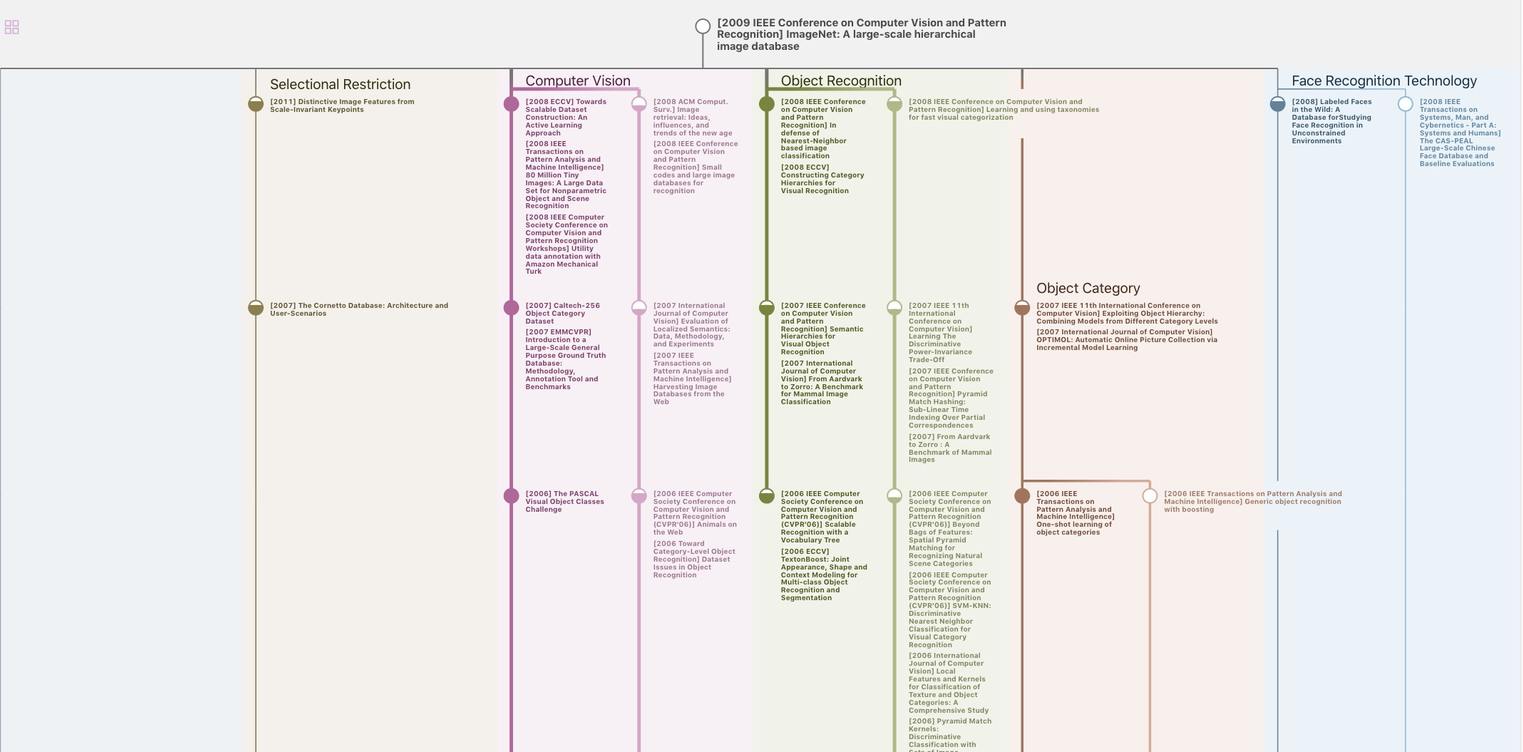
生成溯源树,研究论文发展脉络
Chat Paper
正在生成论文摘要