Learning Lightweight Pedestrian Detector with Hierarchical Knowledge Distillation
2019 IEEE INTERNATIONAL CONFERENCE ON IMAGE PROCESSING (ICIP)(2019)
摘要
It remains very challenging to build a pedestrian detection system for real world applications, which demand for both accuracy and speed. This work presents a novel hierarchical knowledge distillation framework to learn a lightweight pedestrian detector, which significantly reduces the computational cost and still holds the high accuracy at the same time. Following the `teacher–student' diagram that a stronger, deeper neural network can teach a lightweight network to learn better representations, we explore multiple knowledge distillation architectures and reframe this approach as a unified, hierarchical distillation framework. In particular, the proposed distillation is performed at multiple hierarchies, multiple stages in a modern detector, which empowers the student detector to learn both low-level details and high-level abstractions simultaneously. Experiment result shows that a student model trained by our framework, with 6 times compression in number of parameters, still achieves competitive performance as the teacher model on the widely used pedestrian detection benchmark.
更多查看译文
关键词
Pedestrian detection, knowledge distillation, model compression
AI 理解论文
溯源树
样例
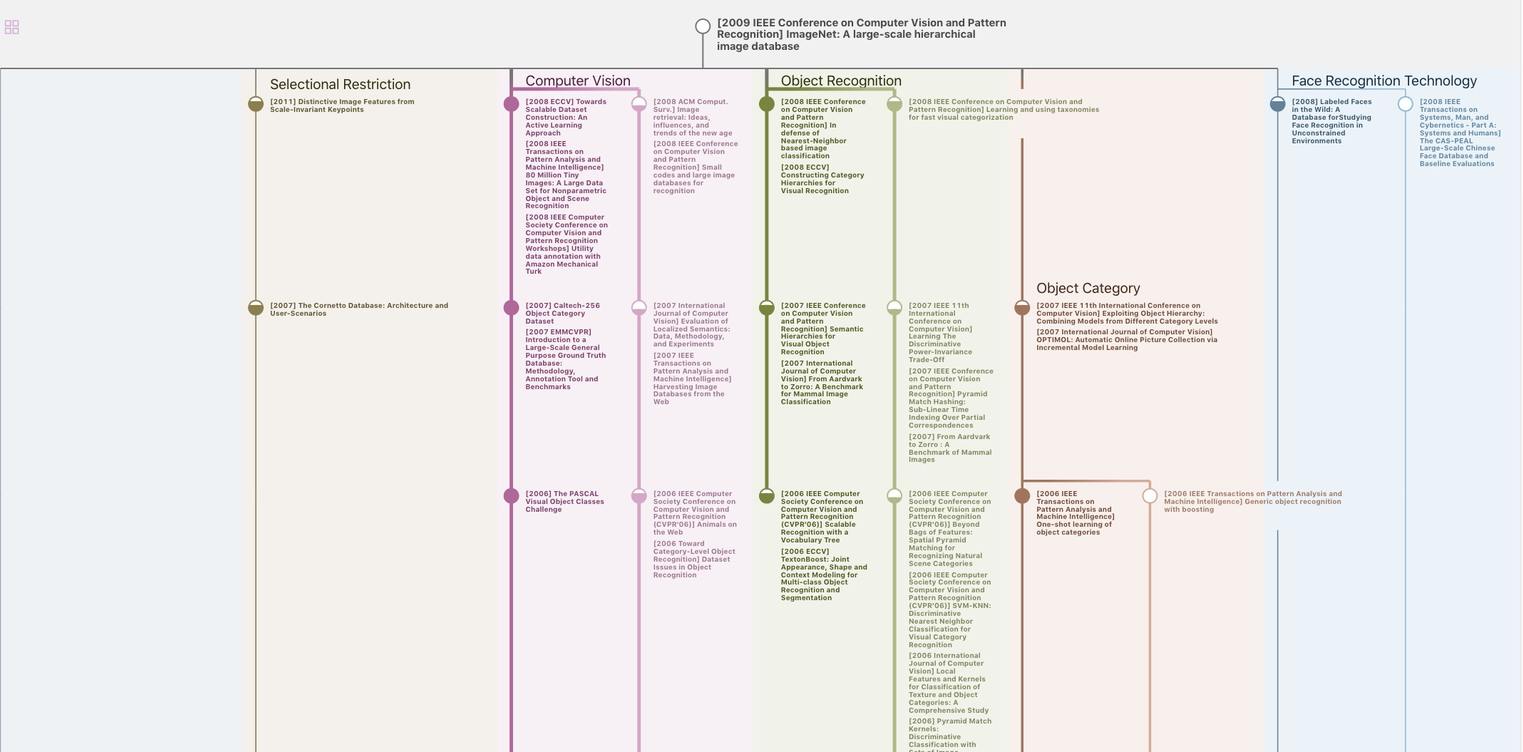
生成溯源树,研究论文发展脉络
Chat Paper
正在生成论文摘要