Efficient Single-Stage Pedestrian Detector By Asymptotic Localization Fitting And Multi-Scale Context Encoding
IEEE TRANSACTIONS ON IMAGE PROCESSING(2020)
摘要
Though Faster R-CNN based two-stage detectors have witnessed significant boost in pedestrian detection accuracy, they are still slow for practical applications. One solution is to simplify this working flow as a single-stage detector. However, current single-stage detectors (e.g. SSD) have not presented competitive accuracy on common pedestrian detection benchmarks. Accordingly, a structurally simple but effective module called Asymptotic Localization Fitting (ALF) is proposed, which stacks a series of predictors to directly evolve the default anchor boxes of SSD step by step to improve detection results. Additionally, combining the advantages from residual learning and multi-scale context encoding, a bottleneck block is proposed to enhance the predictors' discriminative power. On top of the above designs, an efficient single-stage detection architecture is designed, resulting in an attractive pedestrian detector in both accuracy and speed. A comprehensive set of experiments on two of the largest pedestrian detection datasets (i.e. CityPersons and Caltech) demonstrate the superiority of the proposed method, comparing to the state of the arts on both the benchmarks.
更多查看译文
关键词
Pedestrian detection, convolutional neural networks, asymptotic localization fitting
AI 理解论文
溯源树
样例
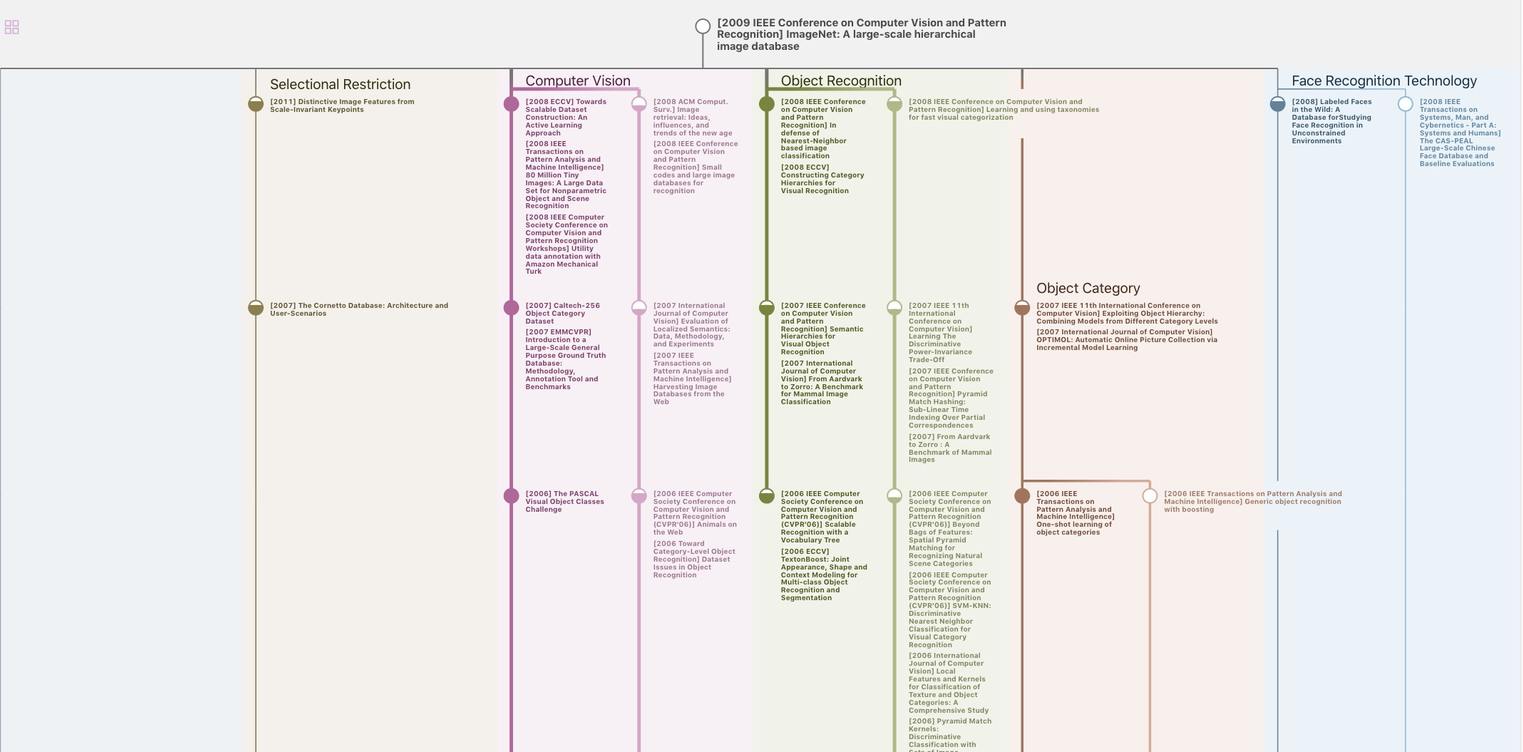
生成溯源树,研究论文发展脉络
Chat Paper
正在生成论文摘要