Scalable Statistical Introgression Mapping Using Approximate Coalescent-Based Inference
BCB(2019)
摘要
Recent advances in biomolecular sequencing have revealed the important role that interspecific gene flow has played in genome evolution throughout the Tree of Life. Current and future genomic studies will bring large amounts of genomic sequence to bear upon this topic, and scalable computational methodologies are needed to detect and analyze genomic signatures of interspecific introgression in large-scale datasets. To address the methodological gap, we introduce a new computational framework known as PHiMM (or "fast PhyloNet + Hidden Markov Model"). PHiMM combines inference and learning under a combined model of genetic drift, substitutions, recombination, and gene flow with a coalescent-based approximation technique. We compare the performance of PHiMM against the state of the art using synthetic and empirical genomic sequence data. We find that PHiMM offers better computational runtime and main memory usage by multiple orders of magnitude, while returning comparable inference accuracy.
更多查看译文
关键词
introgression, coalescent, phylogenetic network, gene flow, hidden Markov model, non-parametric resampling, mouse, butterfly
AI 理解论文
溯源树
样例
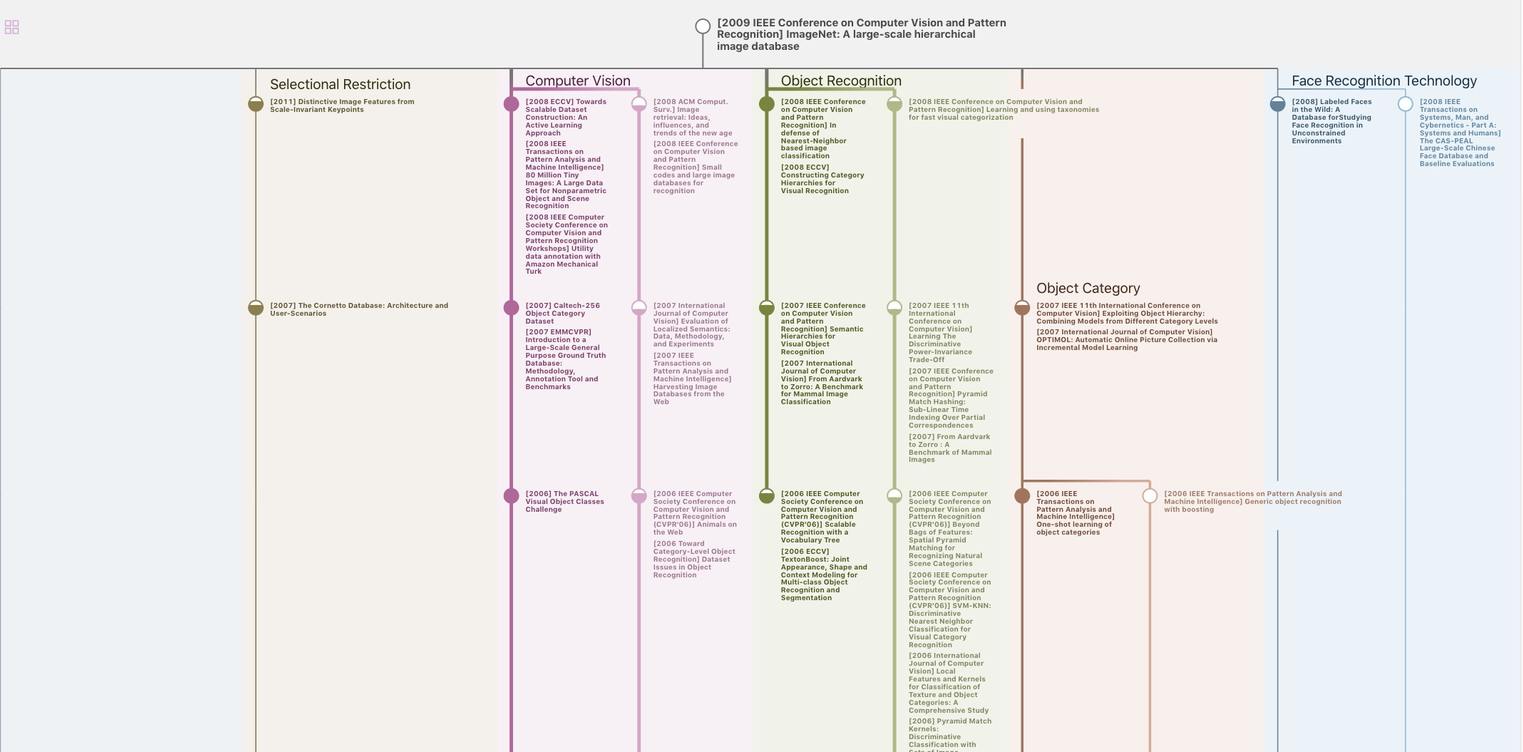
生成溯源树,研究论文发展脉络
Chat Paper
正在生成论文摘要