Collective Proposal Distributions for Nonlinear MCMC samplers: Mean-Field Theory and Fast Implementation
arxiv(2022)
摘要
Over the last decades, various "non-linear" MCMC methods have arisen. While appealing for their convergence speed and efficiency, their practical implementation and theoretical study remain challenging. In this paper, we introduce a non-linear generalization of the Metropolis-Hastings algorithm to a proposal that depends not only on the current state, but also on its law. We propose to simulate this dynamics as the mean field limit of a system of interacting particles, that can in turn itself be understood as a generalisation of the Metropolis-Hastings algorithm to a population of particles. Under the double limit in number of iterations and number of particles we prove that this algorithm converges. Then, we propose an efficient GPU implementation and illustrate its performance on various examples. The method is particularly stable on multimodal examples and converges faster than the classical methods.
更多查看译文
关键词
Sampling algorithm, particle method, propaga-tion of chaos, entropy methods, GPU
AI 理解论文
溯源树
样例
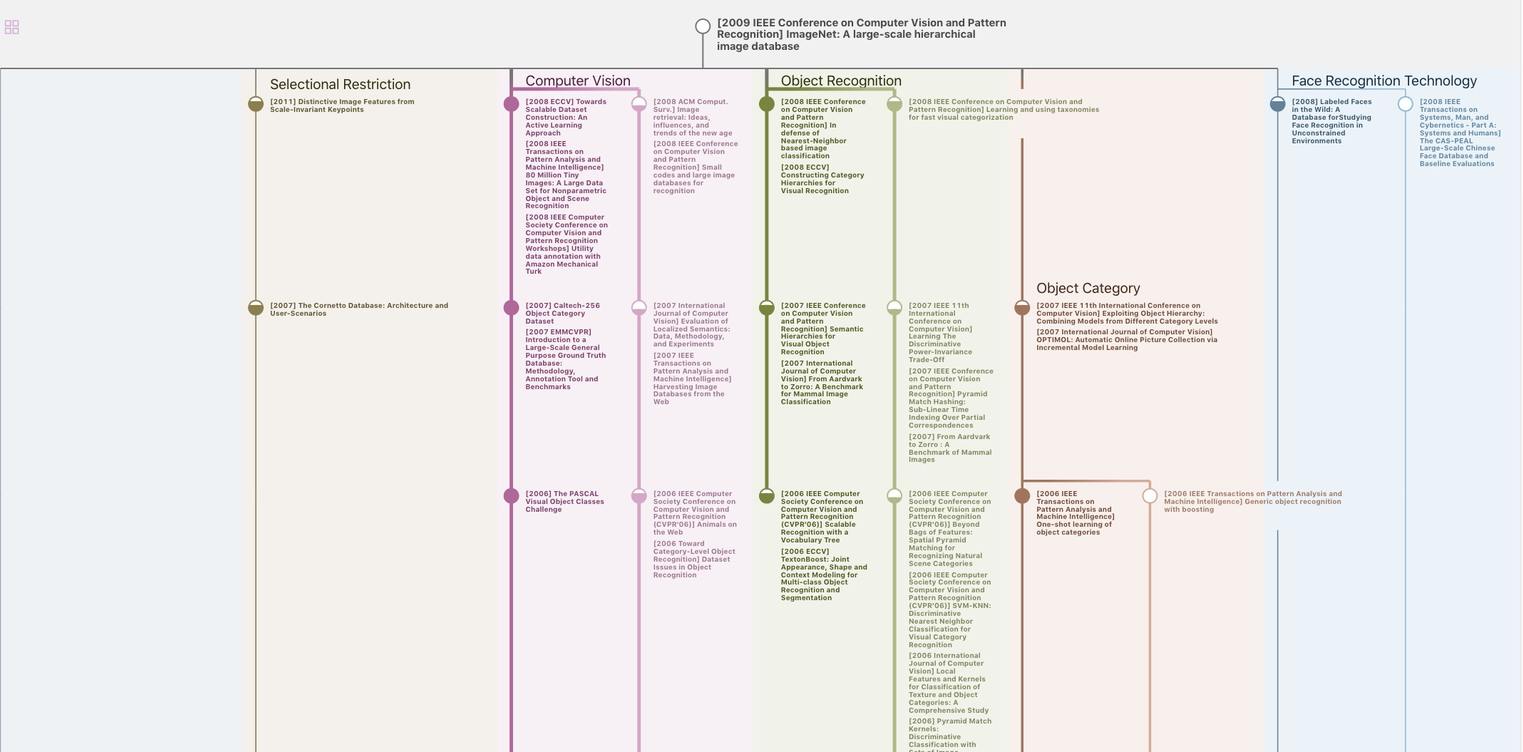
生成溯源树,研究论文发展脉络
Chat Paper
正在生成论文摘要