IR-NAS: Neural Architecture Search for Image Restoration
arxiv(2019)
摘要
Recently, neural architecture search (NAS) methods have attracted much attention and outperformed manually designed architectures on a few high-level vision tasks. In this paper, we propose IR-NAS, an effort towards employing NAS to automatically design effective neural network architectures for low-level image restoration tasks, and apply to two such tasks: image denoising and image de-raining. IR-NAS adopts an flexible hierarchical search space, including inner cell structures and outer layer widths. The proposed IR-NAS is both memory and computationally efficient, which takes only 6 hours for searching using a single GPU and saves memory by sharing cell weights across different feature levels. We evaluate the effectiveness of our proposed IR-NAS on three different datasets, including an additive white Gaussian noise dataset BSD500, a realistic noise dataset SIM1800 and a challenging de-raining dataset Rain800. Results show that the architectures found by IR-NAS have fewer parameters and enjoy a faster inference speed, while achieving highly competitive performance compared with state-of-the-art methods. We also present analysis on the architectures found by NAS.
更多查看译文
AI 理解论文
溯源树
样例
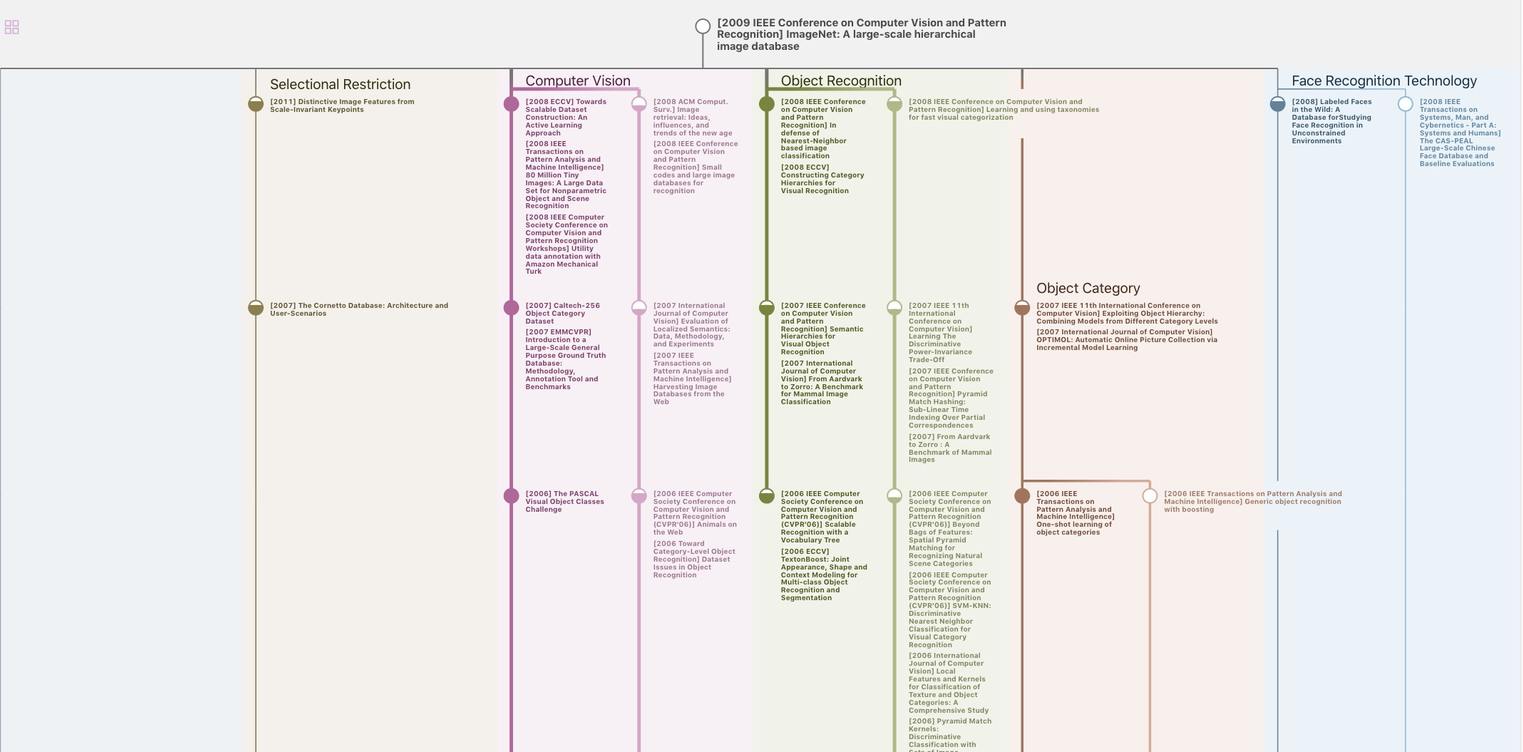
生成溯源树,研究论文发展脉络
Chat Paper
正在生成论文摘要