Robust Statistical Modeling of Monthly Rainfall: The Minimum Density Power Divergence Approach
Sankhya B(2024)
摘要
Statistical modeling of monthly, seasonal, or annual rainfall data is an important research area in meteorology. These models play a crucial role in rainfed agriculture, where a proper assessment of the future availability of rainwater is necessary. The rainfall amount during a rainy month or a whole rainy season can take any positive value and some simple (one or two-parameter) probability models supported over the positive real line that are generally used for rainfall modeling are exponential, gamma, Weibull, lognormal, Pearson Type-V/VI, log-logistic, etc., where the unknown model parameters are routinely estimated using the maximum likelihood estimator (MLE). However, the presence of outliers or extreme observations is a common issue in rainfall data and the MLEs being highly sensitive to them often leads to spurious inference. Here, we discuss a robust parameter estimation approach based on the minimum density power divergence estimator (MDPDE). We fit the above four parametric models to the detrended areally-weighted monthly rainfall data from the 36 meteorological subdivisions of India for the years 1951-2014 and compare the fits based on MLE and the proposed ‘optimum’ MDPDE; the superior performance of MDPDE is showcased for several cases. For all month-subdivision combinations, we discuss the best-fit models and median rainfall amounts.
更多查看译文
关键词
Adjusted-Boxplot method,maximum likelihood estimation,minimum density power divergence estimation,outliers or extreme observations,subdivision-wise areally-weighted rainfall of India,Wasserstein distance
AI 理解论文
溯源树
样例
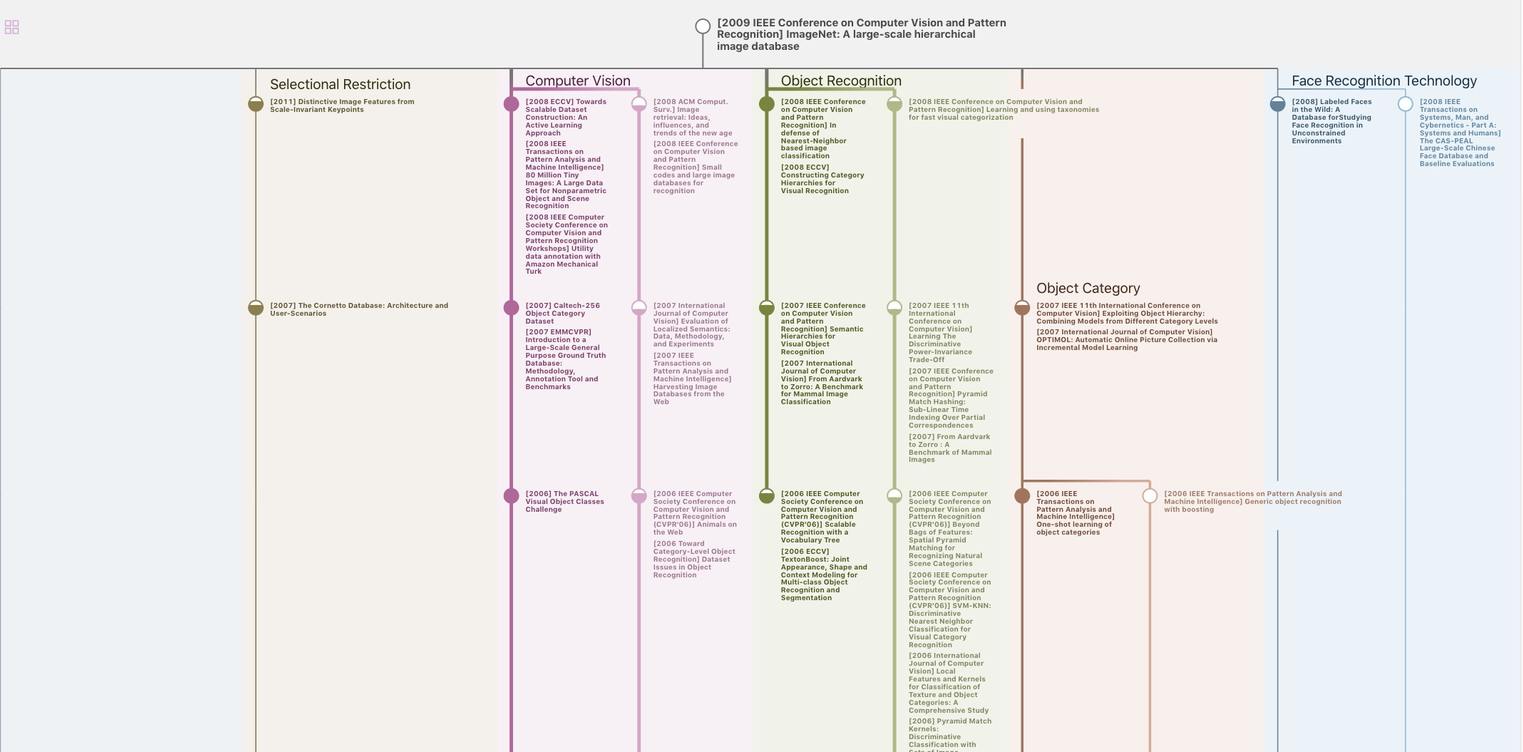
生成溯源树,研究论文发展脉络
Chat Paper
正在生成论文摘要