Multiclass classification of growth curves using random change points and heterogeneous random effects
arxiv(2019)
摘要
Faltering growth among children is a nutritional problem prevalent in low to medium income countries; it is generally defined as a slower rate of growth compared to a reference healthy population of the same age and gender. As faltering is closely associated with reduced physical, intellectual and economic productivity potential, it is important to identify faltered children and be able to characterise different growth patterns so that targeted treatments can be designed and administered. We introduce a multiclass classification model for growth trajectory that flexibly extends a current classification approach called the broken stick model, which is a piecewise linear model with breaks at fixed knot locations. Heterogeneity in growth patterns among children is captured using mixture distributed random effects, whereby the mixture components determine the classification of children into subgroups. The mixture distribution is modelled using a Dirichlet process prior, which avoids the need to choose the "true" number of mixture components, and allows this to be driven by the complexity of the data. Because children have individual differences in the onset of growth stages, we introduce child-specific random change points. Simulation results show that the random change point model outperforms the broken stick model because it has fewer restrictions on knot locations. We illustrate our model on a longitudinal birth cohort from the Healthy Birth, Growth and Development knowledge integration project funded by the Bill and Melinda Gates Foundation. Analysis reveals 9 subgroups of children within the population which exhibit varying faltering trends between birth and age one.
更多查看译文
AI 理解论文
溯源树
样例
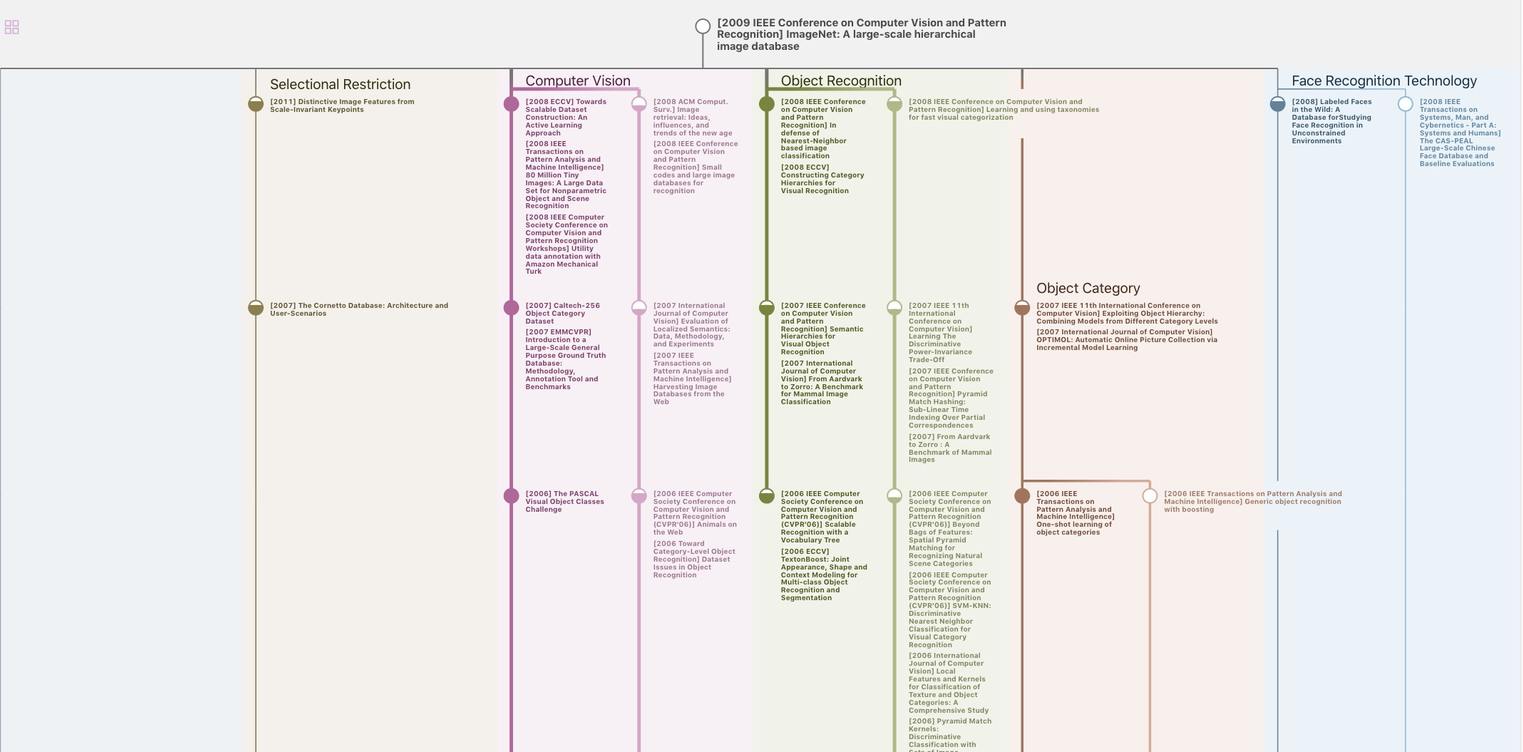
生成溯源树,研究论文发展脉络
Chat Paper
正在生成论文摘要