Learning To Map Nearly Anything
2019 IEEE INTERNATIONAL GEOSCIENCE AND REMOTE SENSING SYMPOSIUM (IGARSS 2019)(2019)
摘要
Looking at the world from above, it is possible to estimate many properties of a given location, including the type of land cover and the expected land use. Historically, such tasks have relied on relatively coarse-grained categories due to the difficulty of obtaining fine-grained annotations. In this work, we propose an easily extensible approach that makes it possible to estimate fine-grained properties from overhead imagery. In particular, we propose a cross-modal distillation strategy to learn to predict the distribution of fine-grained properties from overhead imagery, without requiring any manual annotation of overhead imagery. We show that our learned models can be used directly for applications in mapping and image localization.
更多查看译文
关键词
multi-task learning, weak supervision, semantic transfer, data fusion
AI 理解论文
溯源树
样例
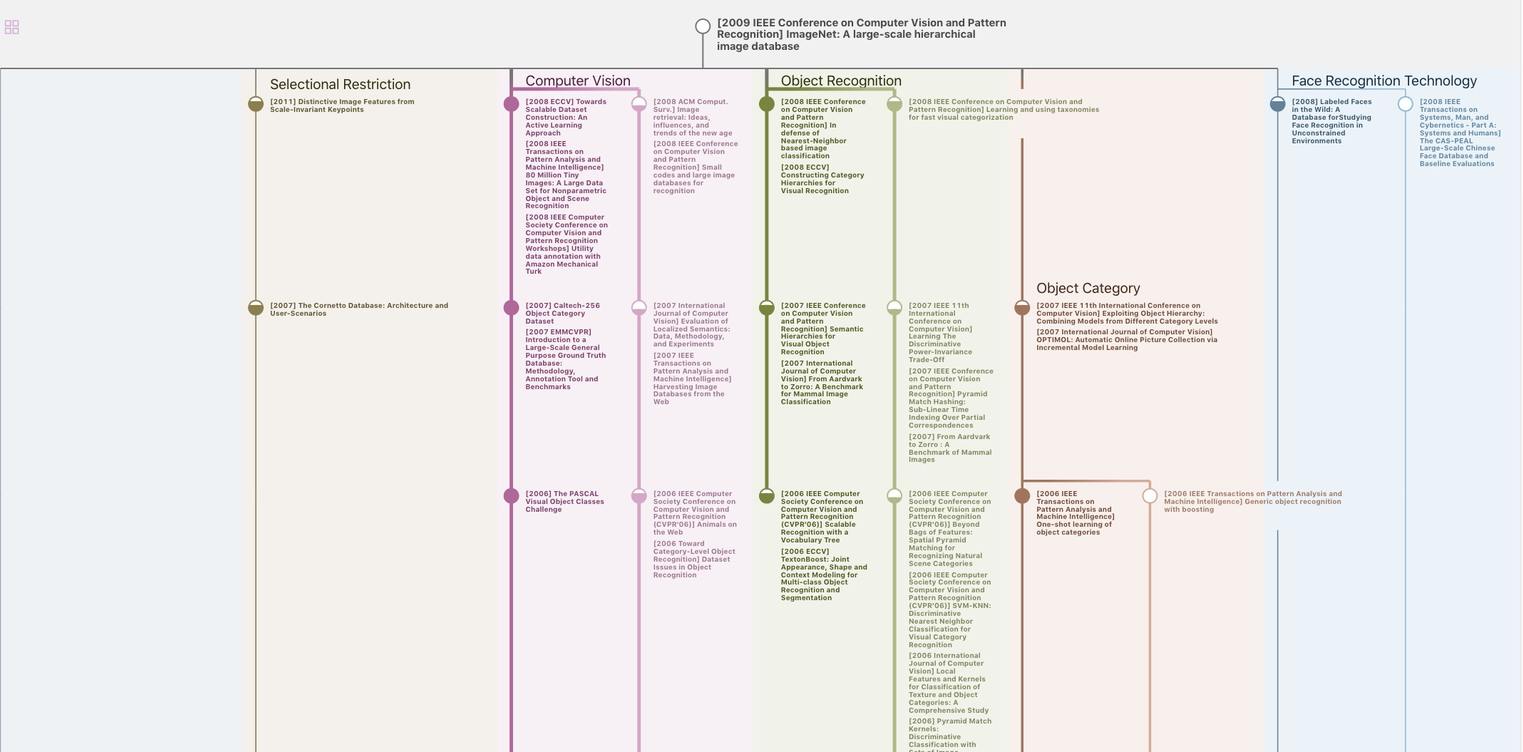
生成溯源树,研究论文发展脉络
Chat Paper
正在生成论文摘要