Real-Time, Highly Accurate Robotic Grasp Detection using Fully Convolutional Neural Network with Rotation Ensemble Module
ICRA(2020)
摘要
Rotation invariance has been an important topic in computer vision tasks. Ideally, robot grasp detection should be rotation-invariant. However, rotation-invariance in robotic grasp detection has been only recently studied by using rotation anchor box that are often time-consuming and unreliable for multiple objects. In this paper, we propose a rotation ensemble module (REM) for robotic grasp detection using convolutions that rotates network weights. Our proposed REM was able to outperform current state-of-the-art methods by achieving up to 99.2% (image-wise), 98.6% (object-wise) accuracies on the Cornell dataset with real-time computation (50 frames per second). Our proposed method was also able to yield reliable grasps for multiple objects and up to 93.8% success rate for the real-time robotic grasping task with a 4-axis robot arm for small novel objects that was significantly higher than the baseline methods by 11-56%.
更多查看译文
关键词
highly accurate robotic grasp detection,fully convolutional neural network,rotation ensemble module,rotation invariance,computer vision tasks,rotation anchor box,multiple objects,4-axis robot arm,Cornell dataset,REM
AI 理解论文
溯源树
样例
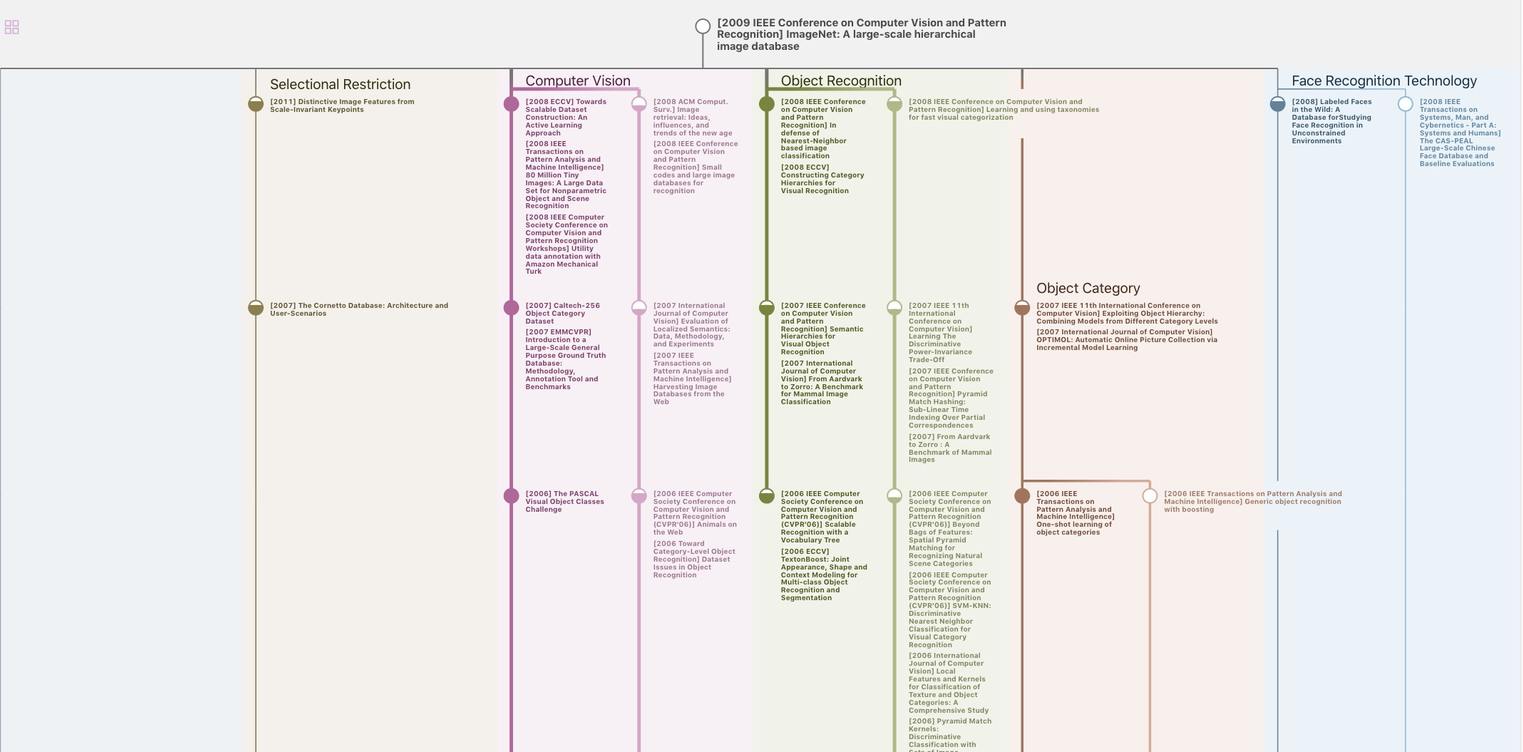
生成溯源树,研究论文发展脉络
Chat Paper
正在生成论文摘要