DeepBbox: Accelerating Precise Ground Truth Generation for Autonomous Driving Datasets
2019 IEEE INTELLIGENT TRANSPORTATION SYSTEMS CONFERENCE (ITSC)(2019)
摘要
Autonomous driving requires various computer vision algorithms, such as object detection and tracking.Precisely-labeled datasets (i.e., objects are fully contained in bounding boxes with only a few extra pixels) are preferred for training such algorithms, so that the algorithms can detect exact locations of the objects. However, it is very time-consuming and hence expensive to generate precise labels for image sequences at scale. In this paper, we propose DeepBbox, an algorithm that corrects loose object labels into right bounding boxes to reduce human annotation efforts. We use Cityscapes dataset to show annotation efficiency and accuracy improvement using DeepBbox. Experimental results show that, with DeepBbox,we can increase the number of object edges that are labeled automatically (within 1% error) by 50 annotation time.
更多查看译文
关键词
DeepBbox,precise ground truth generation,autonomous driving datasets,computer vision algorithms,object detection,object tracking,precisely-labeled datasets,bounding boxes,exact locations,precise labels,image sequences,loose object labels,Cityscapes dataset,object edges,manual annotation time
AI 理解论文
溯源树
样例
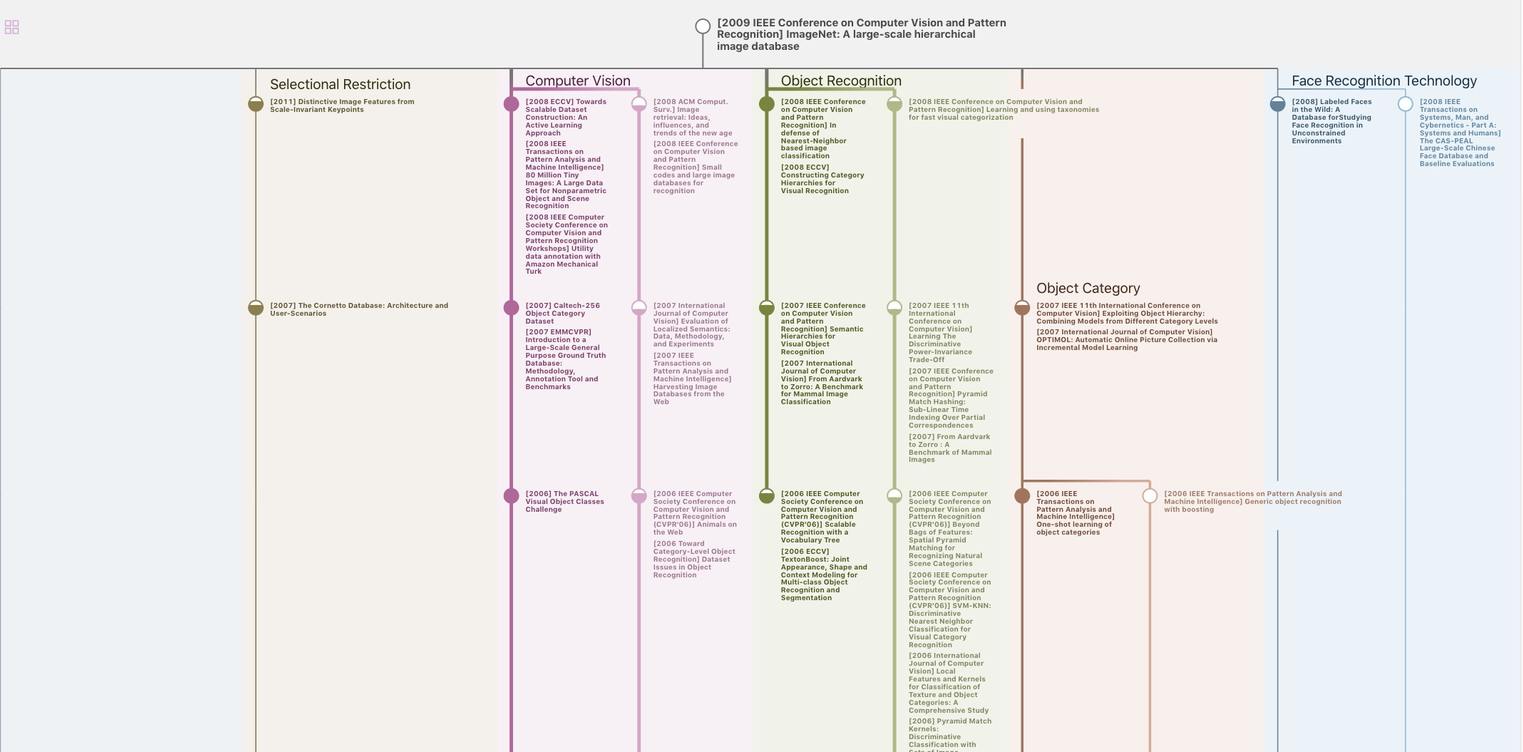
生成溯源树,研究论文发展脉络
Chat Paper
正在生成论文摘要