Minimizing Margin of Victory for Fair Political and Educational Districting
AAMAS '19: International Conference on Autonomous Agents and Multiagent Systems Auckland New Zealand May, 2020(2020)
摘要
In many practical scenarios, a population is divided into disjoint groups for better administration, such as electorates into political districts and students into school districts. However, grouping people arbitrarily may lead to biased partitions, raising concerns of gerrymandering in political districting and racial segregation in schools. To counter such issues, in this paper, we conceptualize such problems in a voting scenario, and given an initial grouping, we propose the Fair Regrouping problem to redistribute a given set of people into k groups, where each person has a preferred alternative and a set of groups they can be moved to, such that the maximum margin of victory of any group is minimized. We also propose the Fair Connected Regrouping problem which additionally requires the people within each group to be connected. We show that the Fair Regrouping problem is NP-complete for plurality voting even if we have only 3 alternatives, but admits polynomial time algorithms if everyone can be moved to any group. We further show that the Fair Connected Regrouping problem is NP-complete for plurality voting even if we have only 2 alternatives and k = 2. Finally, we propose heuristic algorithms for both problems and show their effectiveness in political districting in the U.K. and in lowering racial segregation in public schools in the U.S.
更多查看译文
AI 理解论文
溯源树
样例
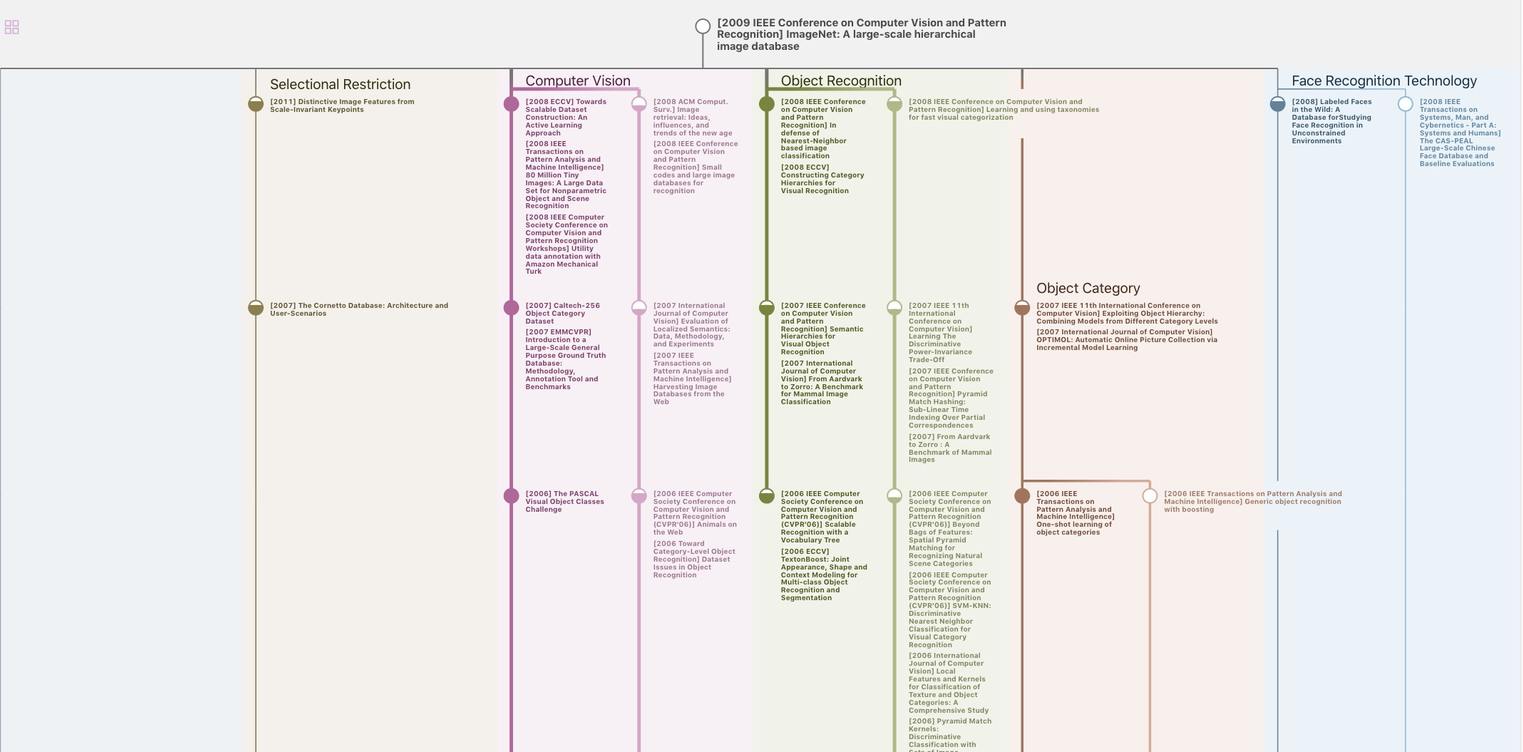
生成溯源树,研究论文发展脉络
Chat Paper
正在生成论文摘要