Building Task-Oriented Visual Dialog Systems Through Alternative Optimization Between Dialog Policy and Language Generation
EMNLP/IJCNLP (1)(2019)
摘要
Reinforcement learning (RL) is an effective approach to learn an optimal dialog policy for task-oriented visual dialog systems. A common practice is to apply RL on a neural sequence-to-sequence (seq2seq) framework with the action space being the output vocabulary in the decoder. However, it is difficult to design a reward function that can achieve a balance between learning an effective policy and generating a natural dialog response. This paper proposes a novel framework that alternatively trains a RL policy for image guessing and a supervised seq2seq model to improve dialog generation quality. We evaluate our framework on the GuessWhich task and the framework achieves the state-of-the-art performance in both task completion and dialog quality.
更多查看译文
AI 理解论文
溯源树
样例
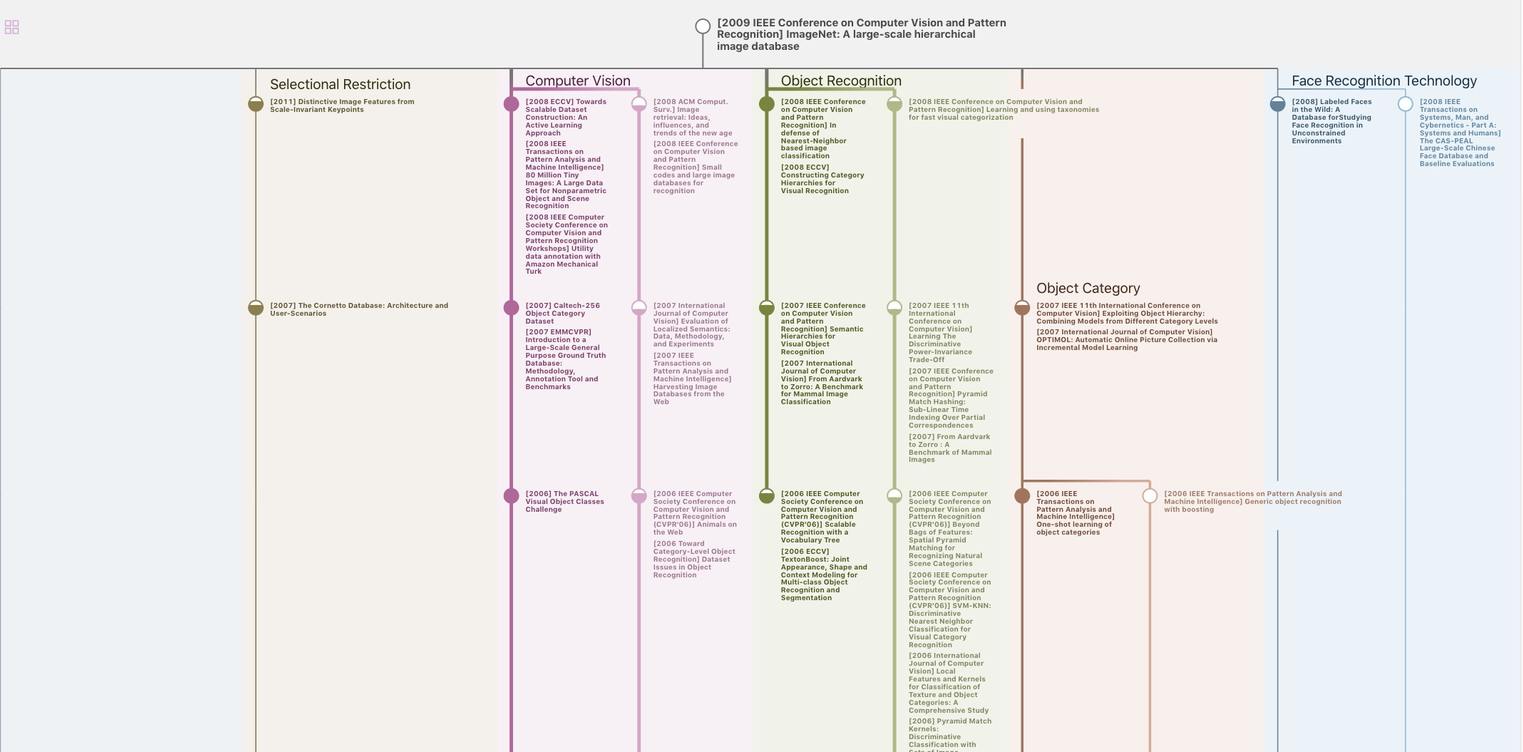
生成溯源树,研究论文发展脉络
Chat Paper
正在生成论文摘要