Maximum Information Gain Relief Feature Weighting
Proceedings of the 2019 International Conference on Artificial Intelligence and Computer Science(2019)
摘要
Features selection can improve the accuracy of the model by reducing the number of input features and has become the focus of many researches in areas of application [15]. Feature selection mainly includes two classes: Filter and Wrapper [16]. In this paper, an extensively-used filter method, Relief feature weighting algorithm, is analyzed.
In 1990s, Kira proposed the Relief algorithm [10], which is originally designed for binary classification. The test results suggest that Relief has advantages in terms of learning time and learning concept and the method is easy to implement for practice.
The iterative Relief algorithm proposed in [12] constructs objective function based on the margin maximization theoretically, it can make up for the lack of clear objective function in the traditional Relief. Afterwards, the EM-like learning strategy is used to derive the weight vector. Recently, Maximum Entropy Relief, which is based on the Maximum Entropy Principle, was proposed in [1] and extended to multi-class classification problems. Local consistency Relief was proposed in [2], and quotes local consistency regularization term.
In this paper, we propose the Maximum Information Gain Relief (MIG-Relief) and present the theoretical analysis and case studies. We analyze the mathematical theory and applicability for the proposed method and show the criterion of parameter selection for feature dimensions with cross validation. A new measuring function of fuzzy difference degree and objective function are proposed and followed by the solution of optimization objective function by Lagrange optimization [8].
更多查看译文
关键词
Feature selection, Lagrange optimization, Maximum Information Gain, Relief
AI 理解论文
溯源树
样例
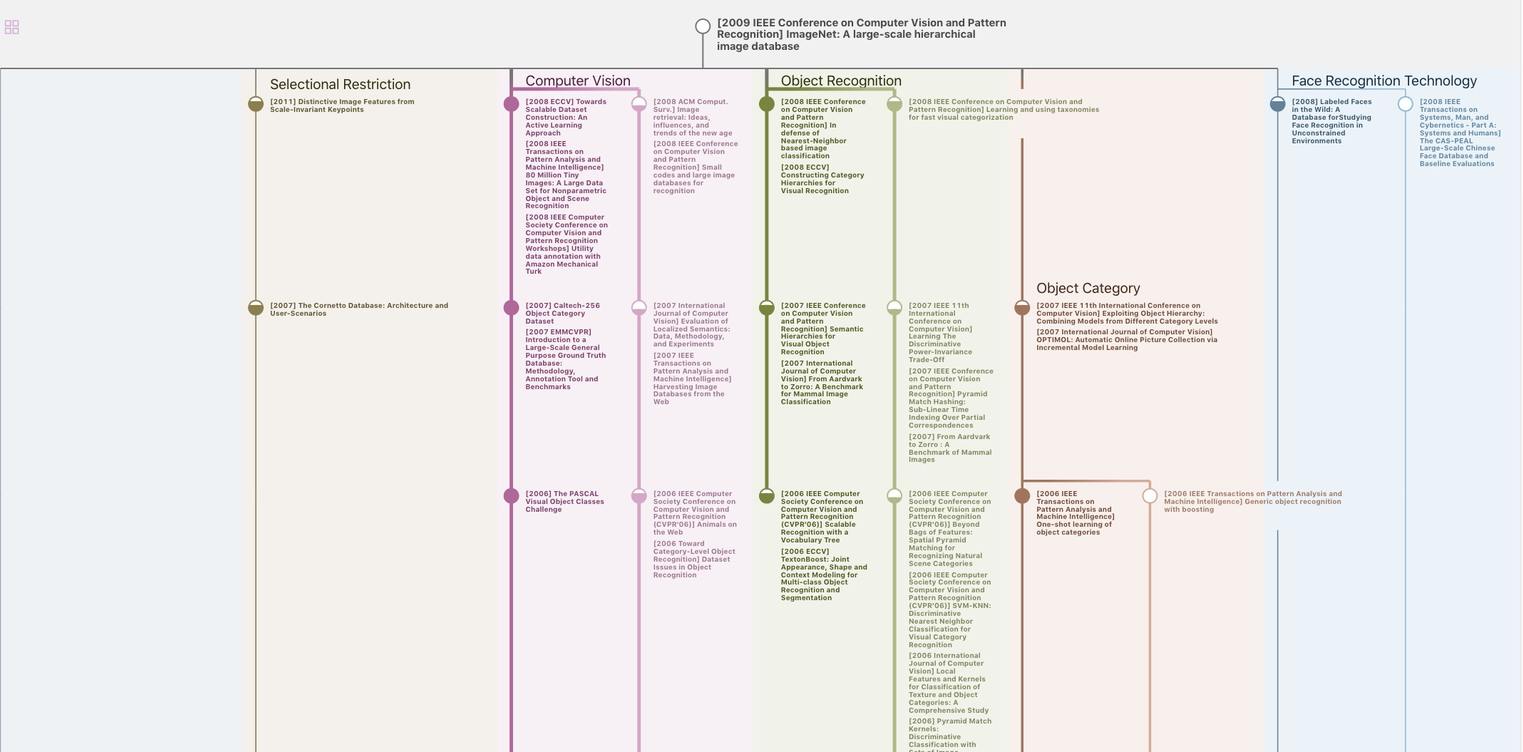
生成溯源树,研究论文发展脉络
Chat Paper
正在生成论文摘要