Improving Deep Generative Models With Randomized Smiles
ARTIFICIAL NEURAL NETWORKS AND MACHINE LEARNING - ICANN 2019: WORKSHOP AND SPECIAL SESSIONS(2019)
摘要
A Recurrent Neural Network (RNN) trained with a set of molecules represented as SMILES strings can generate millions of different valid and meaningful chemical structures. In most of the reported architectures the models have been trained using a canonical (unique for each molecule) representation of SMILES. Instead, this research shows that when using randomized SMILES as a data amplification technique, a model can generate more molecules and those are going to accurately represent the training set properties. To show that, an extensive benchmark study has been conducted using research from a recently published article which shows that models trained with molecules from the GDB13 database (975 million molecules) achieve better overall chemical space coverage when the posterior probability distribution is as uniform as possible. Specifically, we created models that generate nearly all the GDB-13 chemical space using only 1 million molecules as training set. Lastly, models were also trained with smaller training set sizes and show substantial improvement when using randomized SMILES compared to canonical.
更多查看译文
关键词
Cheminformatics, Molecular generative models, Randomized SMILES, Molecular databases, Recurrent Neural Networks, Benchmarking
AI 理解论文
溯源树
样例
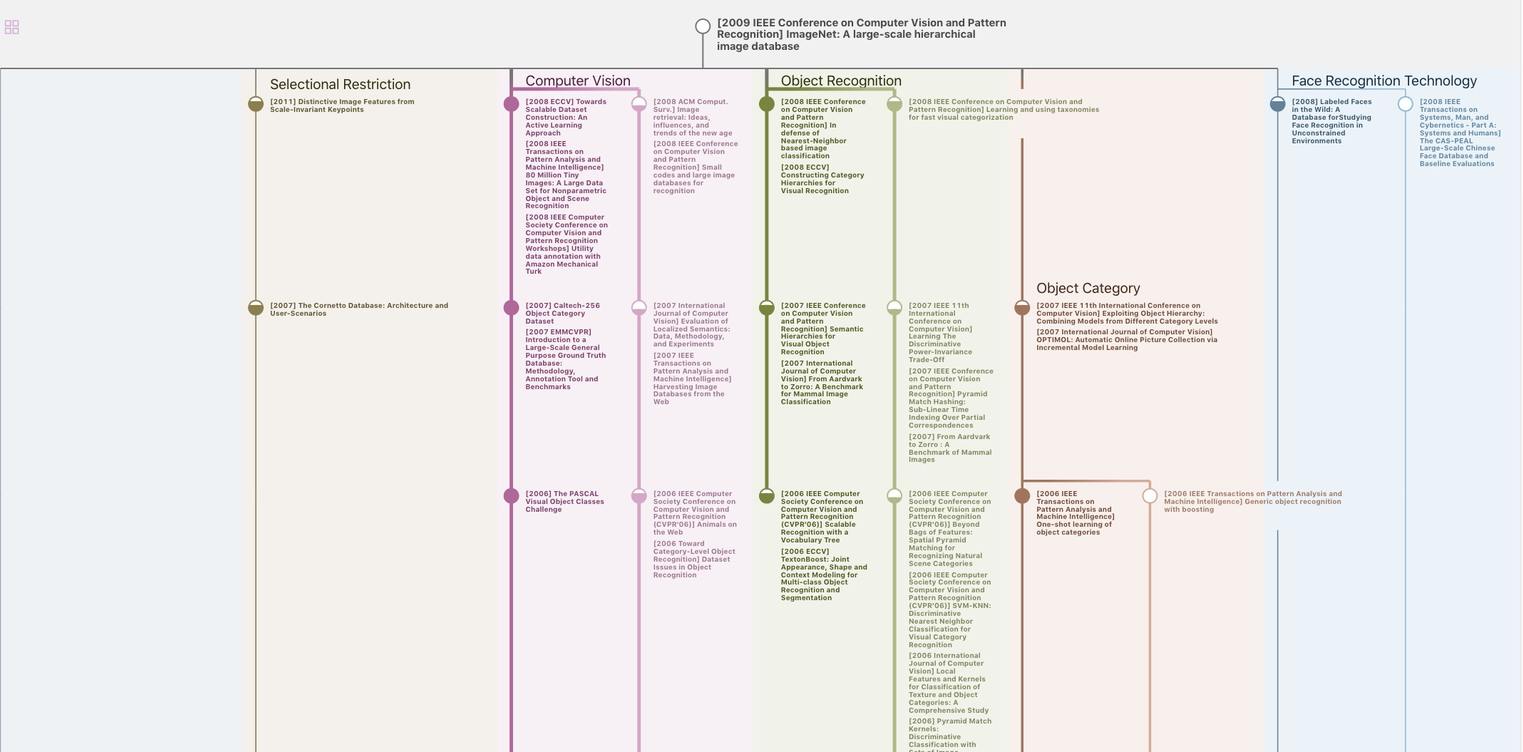
生成溯源树,研究论文发展脉络
Chat Paper
正在生成论文摘要