ORSUM 2019 2nd workshop on online recommender systems and user modeling
Proceedings of the 13th ACM Conference on Recommender Systems(2019)
摘要
The ever-growing nature of user generated data in online systems poses obvious challenges on how we process such data. Typically, this issue is regarded as a scalability problem and has been mainly addressed with distributed algorithms able to train on massive amounts of data in short time windows. However, data is inevitably adding up at high speeds. Eventually one needs to discard or archive some of it. Moreover, the dynamic nature of data in user modeling and recommender systems, such as change of user preferences, and the continuous introduction of new users and items make it increasingly difficult to maintain up-to-date, accurate recommendation models. The objective of this workshop is to bring together researchers and practitioners interested in incremental and adaptive approaches to stream-based user modeling, recommendation and personalization, including algorithms, evaluation issues, incremental content and context mining, privacy and transparency, temporal recommendation or software frameworks for continuous learning.
更多查看译文
关键词
data streams, online learning, recommender systems
AI 理解论文
溯源树
样例
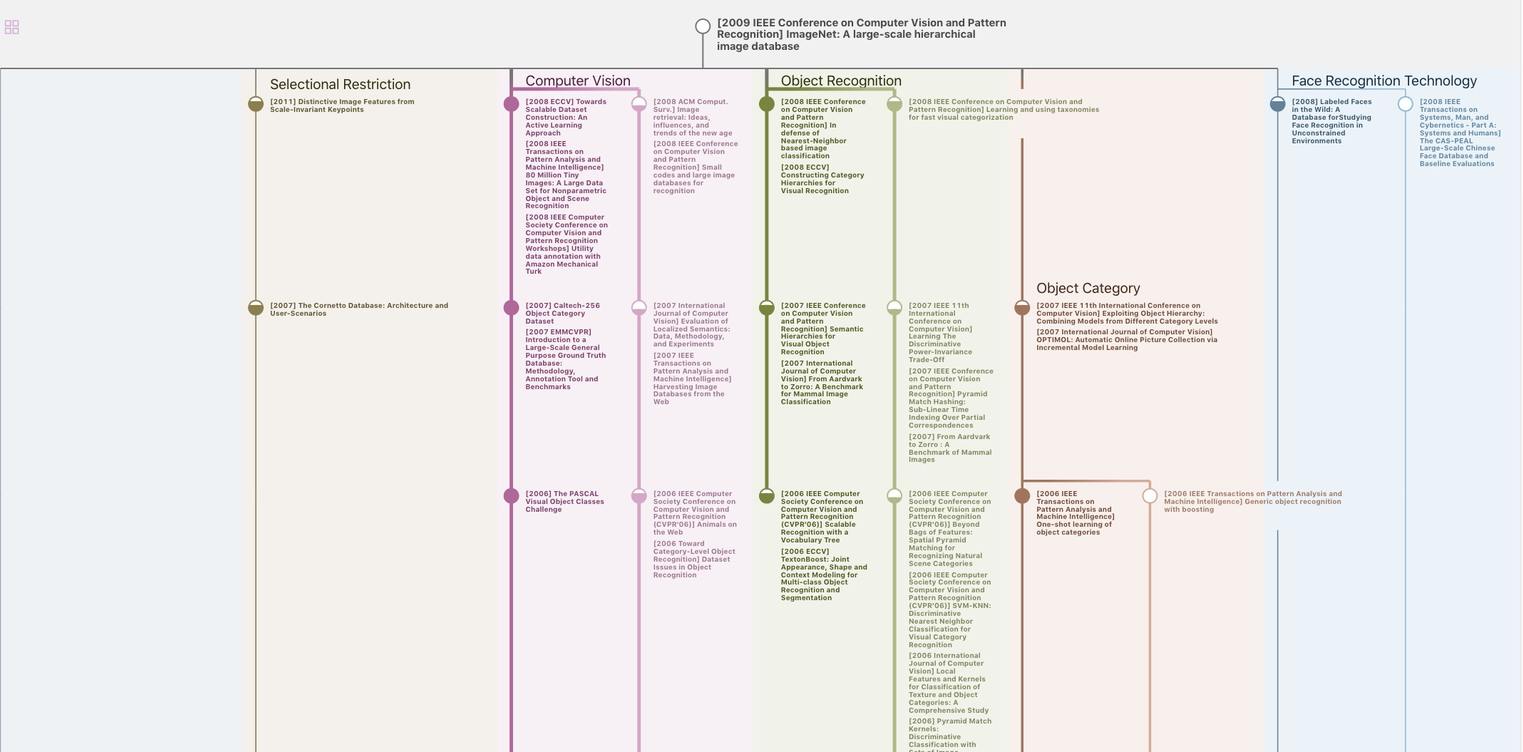
生成溯源树,研究论文发展脉络
Chat Paper
正在生成论文摘要